The Physical AI Revolution Part II: Dawn of the True Robotics Industry
Understanding the New Robotics Ecosystem
Introduction: On the Edge of Automation
Every few weeks, I hear someone confidently proclaiming "2025 will be the year of AI-powered robots". In many ways, they're right – we're witnessing unprecedented momentum in Physical AI, massive bets on humanoid robotics, and ambitious visions of robot butlers entering our homes. But this wave of excitement is remarkably new, and its sudden emergence masks a deeper truth about where we actually stand in the history of automation.
Not long ago, robotics was considered an unattainable dream, far too distant from commercialization to be taken seriously. Even the most successful examples remained confined to specially-designed factory floors, while the autonomous vehicle boom, despite consuming billions in capital, repeatedly fell short on its grand vision. More fundamentally, none of these attempts created robots that could perform one human unit of work. They were compromises and pale imitations of the capabilities people imagined when people dreamed of robots.
The challenges seemed insurmountable. Sensors and actuators were inefficient and fragile, failing to provide the dexterity required for human-level capabilities. Software systems lacked the sophistication to handle unpredictable environments. The decades-long hobbyist culture created an environment where commercial breakthroughs seemed perpetually out of reach. As recently as 2021, even optimistic projections didn't see functional humanoids arriving before 2030. The robotics industry didn't really exist, and is still yet to be born.
A new wave of robots is approaching fast. NVIDIA’s announcements in GTC 2025 (March) will trigger unprecedented momentum in Physical AI. Once it begins, there’s no stopping it.
But, no one could have predicted how dramatically the timeline would accelerate, pulling the future of robotics forward by half a decade. We now stand at the threshold of what promises to be the first true robotics industry in human history. Technological and geopolitical factors have aligned to create a wave of automation that will alter the very fabric of civilization. Within a year or two, we'll see mass-produced, general-purpose robots capable of serving as drop-in replacements for human labor.
The transformation we're about to see is far more profound than just another technological boom. As robots emancipate humanity from manual work, they will cause a perfect storm that will dismantle the age-old constraints of human physical labor that form the very backbone of our civilization. While this seismic shift presents a once-in-a-lifetime opportunity for a few visionaries, most will find themselves on the sidelines of history, forced into this cruel race against their will. There is no middle ground – you either survive or perish.
As an engineer and entrepreneur building physical AI technologies for this emerging industry, I feel compelled to share what I know about this pivotal shift. My goal is to provide a concrete understanding of where we stand, so we can prepare for what's coming. The tunnel ahead is long and dark, but by understanding the forces at play, we might just find our way through to the other side.
Contents
This article is the second in a series of five dedicated to covering various aspects of the upcoming humanoid/robotics industry. Part 1 traced the field's evolution through five distinct phases: from early dreams of the 1970s, through cycles of disappointment with Boston Dynamics and Google, to Tesla's 2021 reboot, the impact of generative AI, and the acceleration of 2024.
Throughout this piece (Part 2), we'll examine the forces shaping the emerging robotics industry. We'll analyze the technological breakthroughs and geopolitical changes converging to create this pivotal moment, alongside the products and players poised to determine the trajectory of the ecosystem.
The article covers five key areas:
What is a Robot: Defining robotics for the age of physical AI and examining why this technological breakthrough represents a fundamental shift in human civilization.
Five Levels of Autonomy: Tracing the evolution of autonomy from Structured Manipulation of early industrial automation (Level 1), through the age of Foundational Robotics we're about to enter (Level 3), to Autonomous Systems of fully-orchestrated supply chains, cities, and nations (Level 5).
Why It Never Worked: The obstacles that held back the creation of machines with human-level capabilities.
What’s Different Now: The technological readiness and geopolitical imperatives driving momentum toward the birth of the first true robotics industry.
Anatomy of the Emerging Industry: Products, players, and competitive dynamics that will shape the industry's turbulent early years.
Future articles will explore potential evolution scenarios of the industry, implications for civilization, and connections to artificial general intelligence (AGI). But first, we must understand what truly differentiates this moment in history – why after decades of false starts and failed promises, we finally stand at the dawn of a real robotics revolution.
I. What Is a Robot and Why Do We Care?
1. Beyond Assembly Lines: Redefining the Robot
When most people think of robots, they envision industrial arms welding car frames or autonomous vacuums roaming living room floors. While these examples represent important milestones in automation, they capture only a fraction of robotics' true potential. A robot, in its fullest sense, is a machine designed to serve as a drop-in replacement for human labor: one that navigates unstructured environments, manipulates objects with human-like dexterity, and executes complex tasks with minimal oversight.
This vision of robotics gives rise to "Physical AI" – the fusion of mechanical capability with software intelligence. Just as humans integrate sensory input, cognitive processing, and physical action to interact with the world, physical AI combines advanced sensors, sophisticated algorithms, and precise actuators to perceive, reason about, and shape its surroundings. These systems transcend pre-programmed routines, developing the ability to learn, adapt, and respond to novel situations. The true promise lies in this unity of agile hardware and advanced intelligence: machines that can transform any human-centric environment into their workspace without requiring radical modifications.
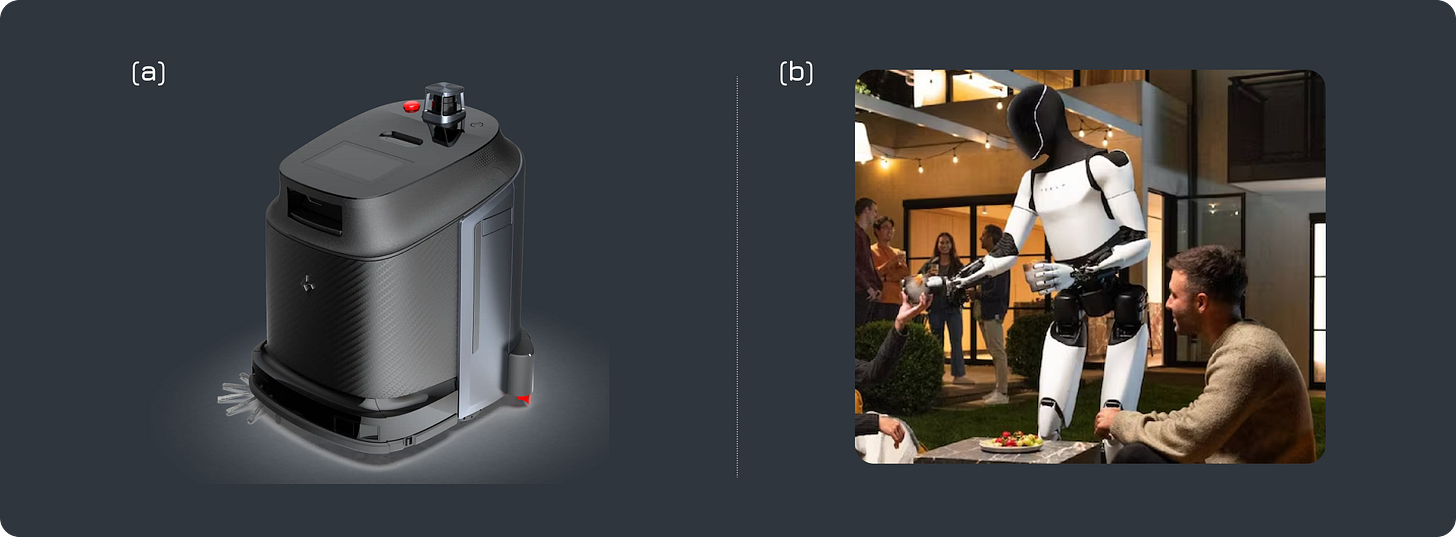
This marks a fundamental departure from traditional automation, which has historically confined itself to specialized machines performing narrow tasks in controlled settings. While such automation improved efficiency in specific contexts, it never achieved the versatility needed to handle the full spectrum of human work. Physical AI bridges this gap through an essential trinity of capabilities: perception, cognition, and manipulation. By empowering machines to operate in dynamic environments without constant reconfiguration or human babysitting, it represents not an incremental upgrade but a revolutionary leap toward systems capable of matching, and potentially exceeding, human labor capacity.
2. General-Purpose Mobile Manipulation
The next wave of robotics transcends traditional automation's narrow focus on repetitive tasks. At its core lies General-Purpose Mobile Manipulation (GPMM) – the sophisticated fusion of movement and dexterity that enables machines to operate as true replacements for human labor. Unlike specialized industrial robots that perform fixed functions in meticulously-configured settings, true robots adapt seamlessly to human environments, handling diverse tasks that involve both navigation and maneuvering without hardware modifications.
A robot is a machine that can perform one human unit of work. This definition requires General-Purpose Mobile Manipulation (GPMM) – the ability to both navigate and execute diverse tasks in unstructured environments with a single, versatile physical platform.
Multipurpose drones represent another critical vector, evolving beyond simple surveillance or delivery tasks toward active environmental interaction. Advanced aerial platforms equipped with manipulation capabilities can perform infrastructure maintenance, construction support, or emergency response operations. Their ability to coordinate in swarms while handling complex physical tasks opens entirely new possibilities for automated labor, particularly in environments too dangerous or inaccessible to human workers.
General-purpose mobile manipulation platforms share a crucial characteristic: they require no more setup or modification than human workers would need. Rather than forcing environments to adapt around machines, they integrate seamlessly into existing workflows and spaces. This compatibility, combined with increasingly sophisticated Physical AI, enables them to handle the dynamic, unstructured challenges that have historically required human adaptability. The emergence of these capable platforms signals robotics' evolution from isolated demonstrations into true industry.
3. Why Humanoids Matter
Among various form factors, humanoids represent the most direct path toward widespread automation. While critics argue that the human form is suboptimal for many physical tasks, this perspective misses a crucial reality: our world is fundamentally designed around human proportions and capabilities. From doorways and staircases to tools and workstations, our environment assumes bipedal mobility and two-handed manipulation. The humanoid form is uniquely positioned for zero-friction integration into existing spaces, with contemporary models featuring increasingly robust hardware and advanced physical AI capabilities.
The economic advantages of the humanoid approach extend beyond compatibility. Developing a single versatile platform rather than numerous specialized devices reduces R&D costs and accelerates innovation through concentrated effort. This unified approach enables economies of scale in manufacturing and maintenance while creating a standardized ecosystem for software development. As capabilities improve, these benefits compound across all deployment scenarios, allowing for rapid adaptation to new tasks without physical redesign. The result is an autonomy platform with unprecedented versatility and significantly lower implementation costs compared to task-specific alternatives.
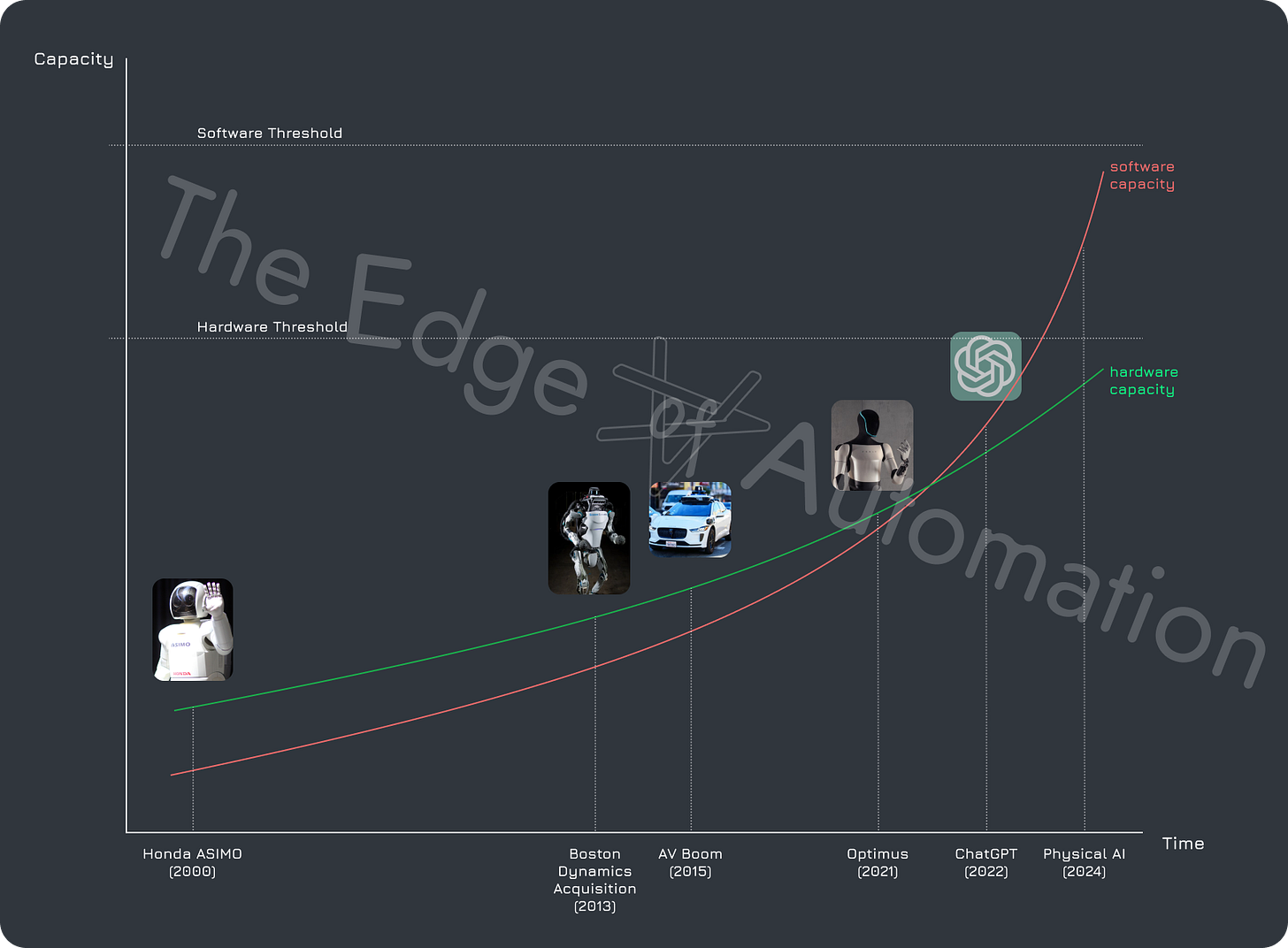
The implications stretch beyond practicality. As humanoids establish themselves in workplaces, they'll create powerful feedback loops reshaping physical infrastructure. While robots will initially adapt to human environments, spaces will gradually evolve to optimize for robots. This transformation enables a smooth transition from human labor to automated systems, setting the stage for more radical innovation: modular robots with interchangeable components, and eventually, orchestrated networks of entire supply chains, cities and nations. Humanoids represent not just an intermediate step, but the crucial bridge propelling us toward a new technological horizon.
The social and psychological dimensions of humanoid design prove equally significant. People naturally understand and relate to human-like forms, making humanoids easier to integrate into collaborative workflows. This inherent familiarity reduces training overhead and accelerates acceptance across diverse industries. While future automation may eventually evolve toward more specialized morphologies, humanoids serve as an essential intermediary that will not only enable the initial wave of widespread robotics deployment but also reshape civilization's relationship with physical labor.
4. The Imminent Surge
We stand at the threshold of the most radical transformation in human history. As robots move beyond isolated, heavily constrained applications into mainstream deployment, their impact will ripple through every aspect of our society – from manufacturing floors to supply chains, from domestic spaces to urban infrastructure. The pace of this revolution will likely accelerate exponentially as each breakthrough builds upon previous innovations. The convergence of advanced hardware, sophisticated AI models, and powerful on-board compute will yield machines that can be deployed across diverse settings without months of custom engineering.
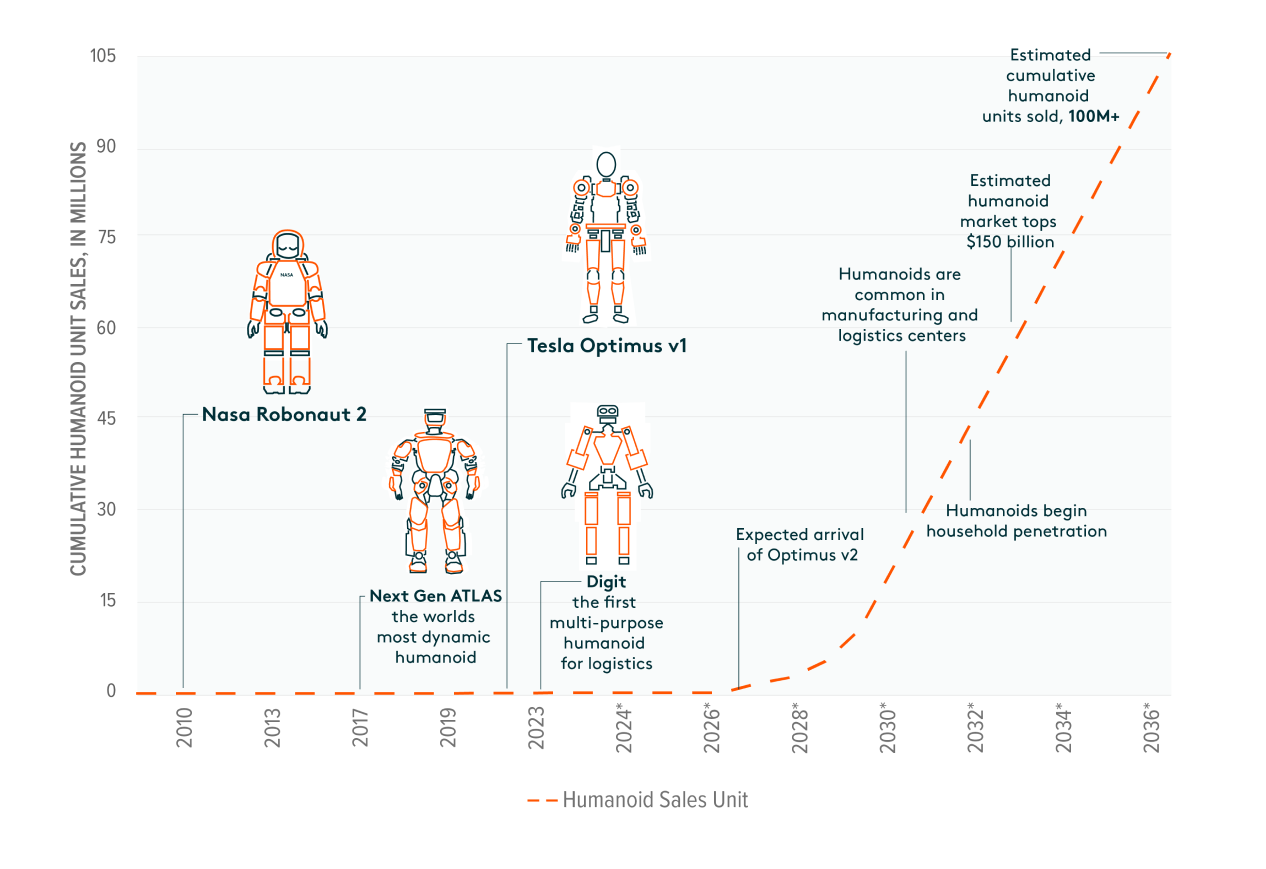
This shift represents more than technological progress. It means the dissolution of humanity's fundamental constraint: the scarcity of physical labor. Just as the internet rewrote the rules of information exchange, truly capable robots will trigger a global chain reaction that transforms our assumptions about productivity, labor value, and economic organization. Nations will race to secure the components of this emerging industry, forging new alliances and rivalries in their quest for manufacturing supremacy. The impact will be immediate and far-reaching, from 24/7 automated factories to the complete restructuring of supply chains, cities, and nations.
The stakes couldn't be higher. For individuals, this represents an unprecedented opportunity to position themselves at the forefront of a technological revolution. For institutions, failure to adapt poses an existential threat. After decades of gestation, we're witnessing the birth of the first true robotics industry, built on breakthroughs in hardware design, software intelligence, and unprecedented alignment of market incentives. The stage is set for a new era of machines that can physically transform the world around us in ways once relegated to science fiction.
To understand the magnitude of this transformation – and to separate genuine innovation from superficial hype – we must map the progression from basic automation to highly autonomous systems. In the next section, we'll explore the Five Levels of Autonomy, a framework that illuminates the path from simple task-specific machines to the versatile, intelligent robots poised to reshape our world.
II. Five Levels of Autonomy
The robotics revolution isn't a sudden leap but a carefully orchestrated progression toward machines that can truly replace human labor. While "autonomy" often appears as an empty buzzword, understanding its spectrum is crucial for grasping why we stand at a unique moment in history. This framework of five distinct levels illuminates not just how far we've come, but why we're finally approaching the birth of a true robotics industry.
These five levels of autonomy are crucial to understanding the robotics revolution. We'll explore each level in greater technical depth in a future article, examining the specific hardware and software breakthroughs that enable progression through this framework.
1. LEVEL 1: Structured Manipulation
The foundation of industrial automation lies in machines that excel at repetitive tasks within tightly controlled environments. Industrial arms can weld, paint, and assemble with remarkable precision, but only because their world is deliberately engineered to eliminate variability. The entire production line must be built around them, with safety fences, conveyor systems, and precise product positioning ensuring they face only predetermined scenarios.
Video: Industrial Arms (Level 1 Autonomy)
While these machines revolutionized manufacturing, their "autonomy" is superficial. They follow rigid scripts that require extensive reprogramming for even minor product variations, and their focus on mechanical precision over adaptive intelligence results in expensive systems that can't handle real-world complexity. This represents everything the coming robotics revolution must overcome: instead of forcing environments to adapt to machines, we need machines that can adapt to human environments. Level 1 Autonomy may have transformed some factories, but falls far short of creating true replacements for human labor.
2. LEVEL 2: Autonomous Navigation
The second level introduces genuine mobility through autonomous vacuums, delivery drones, and self-driving vehicles. These systems use cameras, LIDAR, and GPS to map their environment and navigate dynamic spaces. This leads to a massive complexity increase: processing real-time data and reacting to unexpected obstacles rather than performing fixed actions in controlled settings. The autonomous vehicle industry's repeated setbacks highlight this challenge – even "simple" navigation in unstructured environments demands breakthroughs in perception and decision-making.
Video: Autonomous Vehicle Example by Waymo (Level 2 Autonomy)
Yet Level 2 systems remain severely limited in manipulation. They can transport payloads between fixed points but lack the dexterity needed for manufacturing, maintenance, or household tasks. This fundamental gap between mobility and manipulation explains why Level 2 Autonomy, despite its sophistication, falls short of true robotics. Creating machines that can replace human labor requires unifying these capabilities into single, adaptable systems – a challenge that defines the next level of autonomy and the dawn of real robotics.
3. LEVEL 3: Foundational Robotics
Level 3 Autonomy (Foundational Robotics, or General-Purpose Robotics) marks the beginning of true robotics. This is where navigation and manipulation finally merge, creating machines that can serve as genuine replacements for human labor. These robots combine the mobility to move through spaces with the dexterity to handle diverse objects and tasks, all while adapting to environmental changes with minimal oversight. Unlike specialized systems of previous generations, these general-purpose robots can tackle varied scenarios without hardware modifications, relying instead on adaptable software and versatile physical designs.
General-Purpose Mobile Manipulation (GPMM) represents the technological threshold that sets Level 3 systems apart from their predecessors – the integration point where machines transition from specialized automation to foundational robotics capable of generalized work.
Video: Tesla Optimus Gen 2 (Level 3 Autonomy)
The technological requirements for are immense. Robots must process multiple sensor streams while making split-second decisions about positioning, gripping, and task execution. Physical AI becomes essential, as pre-programmed routines can't handle the complexity of real-world interactions. These systems demand sophisticated models that combine perception, reasoning, and learning into unified frameworks to drive next-generation actuators and sensors for human-like dexterity. The computational requirements involve high-throughput edge processing capabilities that can handle multimodal inputs while maintaining real-time responsiveness. Recent breakthroughs in adaptive control systems and sim-to-real transfer learning have accelerated development, enabling robots to generalize from limited training data to novel situations.
We are entering this phase now. The surge in humanoid robotics and multi-purpose automation signals an unprecedented harmony: AI models sophisticated enough to understand physical interactions, hardware capable of human-like movement, and focused product strategies backed by desperate capital. We're about to see machines that can adapt to human environments rather than requiring environments to adapt to them – a fundamental shift leading to the birth of the true robotics industry. This transformation will reshape every sector of society that previously required human physical labor, from manucacturing and logistics to households and services. Organizations pioneering these systems are positioned to not only establish new platform monopolies but also to reshape the power dynamics of the entire human world.
4. LEVEL 4: Modular Robotics
Where Level 3 establishes the foundation, Level 4 creates an ecosystem of plug-and-play components. The focus shifts from vertically-integrated robots to standardized platforms where hardware and software can be mixed and matched like building blocks. Sensors, actuators, and entire limbs become interchangeable modules, while unified architectures enable rapid deployment of new capabilities across diverse form factors.
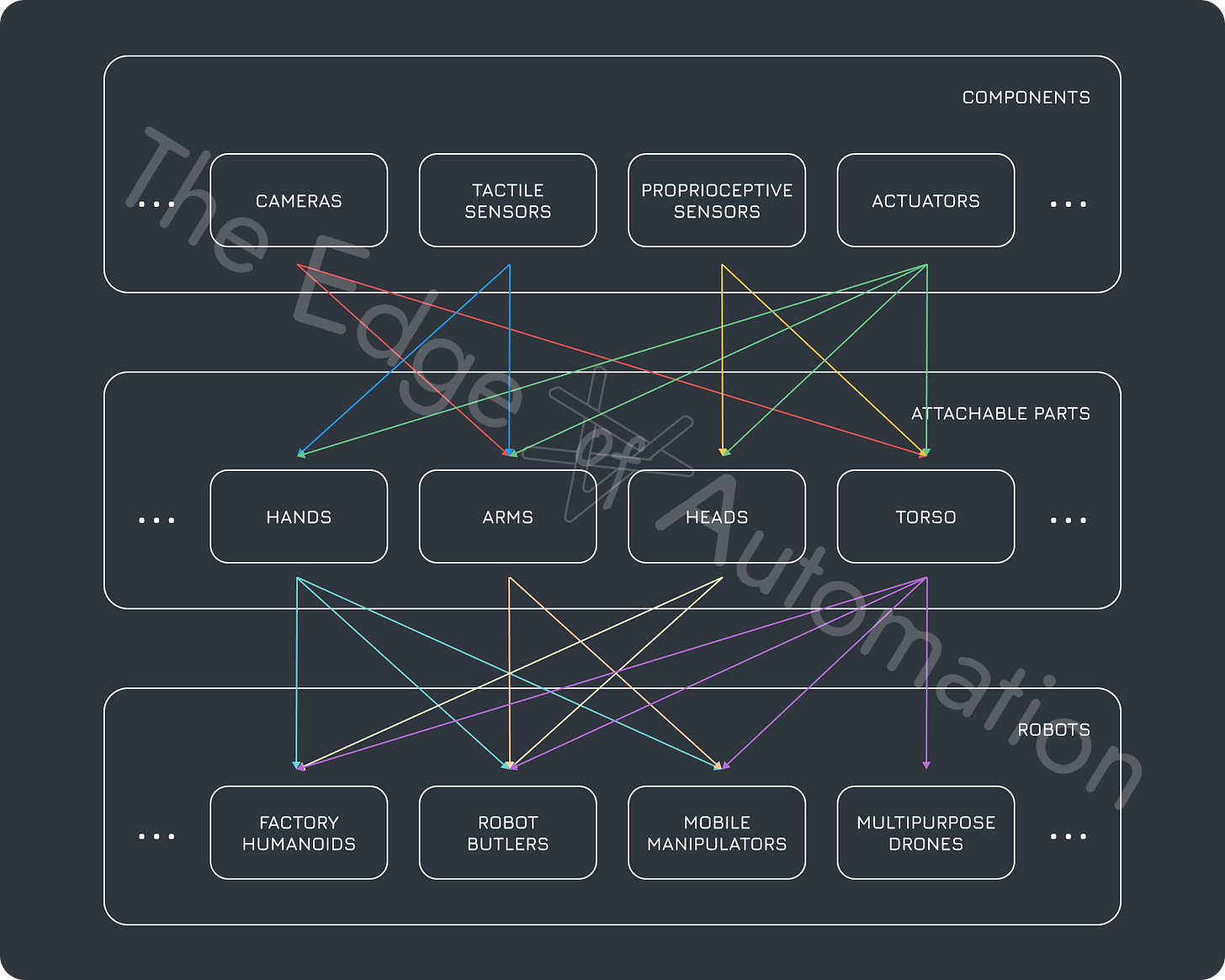
This modularity transforms the economics of robotics. Instead of each company building complete systems from scratch, specialists can focus on developing best-in-class components that plug into standardized interfaces. The result is accelerated innovation, lower costs, and the emergence of vibrant developer ecosystems – similar to how personal computing exploded once hardware and software were decoupled. As robotics moves from integrated systems to modular platforms, we'll see an explosion of new applications and capabilities that were once thought impossible to achieve.
5. LEVEL 5: Autonomous Systems
The final level transcends individual machines to orchestrate entire networks of automated infrastructure. Factories, supply chains, and even cities become integrated systems where physical AI coordinates complex operations without human oversight. Raw materials flow through automated production lines, autonomous vehicles manage logistics networks, and robot fleets maintain urban services in a seamless dance of coordinated automation.
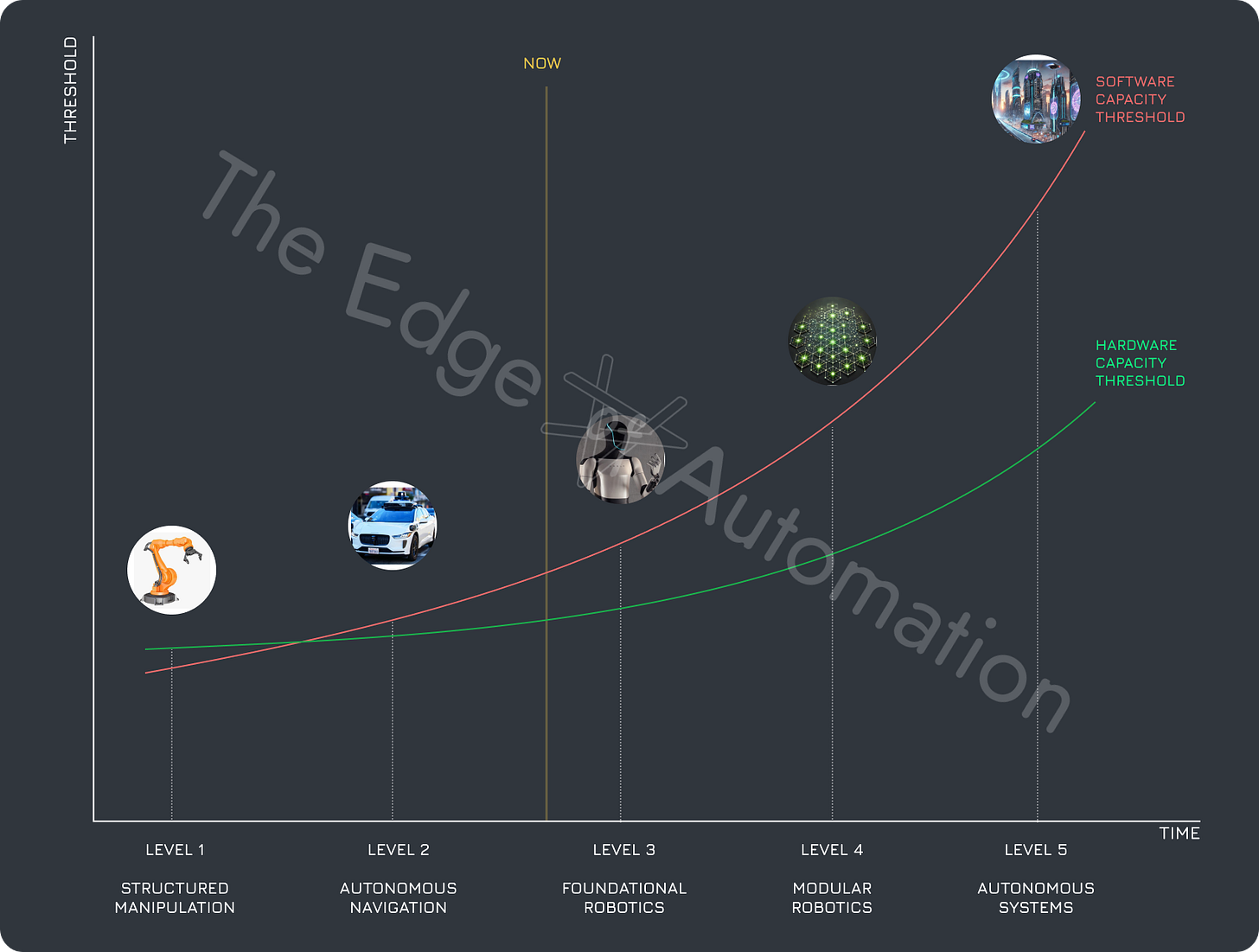
This represents civilization's pivot from human-supervised machines to machine-supervised ecosystems. The technological requirements are staggering: advanced simulation capabilities, orchestration AI handling billions of components in real-time, and hardware robust enough for continuous operation. Yet this is the ultimate destination where physical AI doesn't just replace human labor but reimagines how entire societies function.
The progression through these levels reveals why previous attempts at robotics revolution fell short. While Levels 1 and 2 brought valuable but limited automation, the leap to Level 3 demanded simultaneous breakthroughs in hardware, software, and system design that remained out of reach. Now, as these barriers finally fall, we're witnessing the birth of an industry that will fundamentally transform human civilization.
A future article will provide a comprehensive technical analysis of these five levels, including detailed examinations of the hardware configurations, software architectures, and AI models that enable each stage of autonomy.
III. Why It Never Worked: The Perfect Storm of Barriers
For decades, robotics remained trapped in a paradox: billions in investment and countless breakthroughs never translated into machines capable of matching human capabilities. While industrial arms conquered factory floors and autonomous vehicles made headlines, true robotics – the seamless fusion of mobility, manipulation, and intelligence – stayed perpetually out of reach.
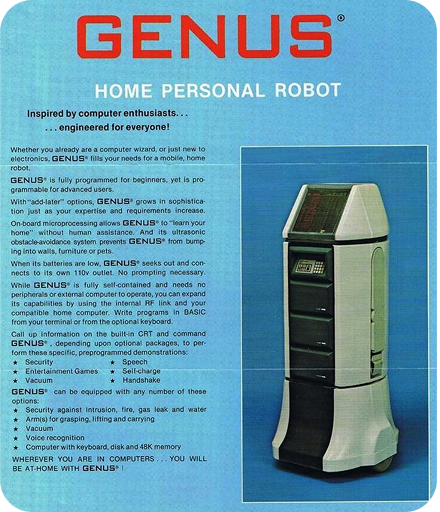
The challenges were comprehensive and mutually reinforcing. Hardware remained either too fragile or prohibitively expensive for real-world deployment. Software proved inadequate for the complexity of physical interaction. More fundamentally, the field never developed the cohesive culture needed to attract elite talent and drive commercial innovation. Instead of maturing into an industry, robotics limped along as a collection of isolated research projects and limited demonstrations. Understanding these obstacles is crucial to grasping why we stand at such a pivotal moment today, as these very barriers finally begin to fall.
1. The Curse of Mobile Manipulation
General-Purpose Mobile Manipulation (GPMM) represents the core challenge that kept robotics from achieving human-level capability. Combining autonomous movement with dexterous manipulation opens up exponential complexity. The instant you place an arm on a moving base – or give it legs – you create a system that must simultaneously maintain balance, navigate space, and manipulate objects with precision. This multifaceted challenge requires orchestrating dozens of joints, sensors, and control systems in millisecond-level coordination while accounting for environmental variability that humans instinctively handle. The computational demands alone would overwhelm processors available even five years ago, requiring breakthroughs in both hardware and software architectures.
This complexity manifests in three critical ways. First, robots must build real-time environmental understanding from noisy sensor data, interpreting not just object locations but their "affordances" – whether a handle can be grasped or a surface can support weight. Second, perception, planning, and motor control must work in perfect harmony. Finally, they must adapt instantly to unexpected changes, from shifting object positions to varying surface textures. Each challenge compounds the others, causing failure cascades where a minor perceptual error leads to catastrophic planning mistakes, or where slight motor miscalibration renders sophisticated environmental models useless. The interdependency of these systems created a technological bottleneck that traditional siloed approaches couldn't overcome.
Video: Humanoid Falls during 2015 DARPA Robotics Challenge
Early attempts highlighted these challenges. Honda's ASIMO could walk and gesture but struggled with novel tasks. The 2015 DARPA Robotics Challenge famously ended with humanoids failing at basic tasks like opening doors or recovering from falls. Even advanced prototypes remained brittle; the slightest deviation from expected conditions would cause system-wide failure. These high-profile disappointments revealed how naively optimistic early robotics timelines had been, with engineers underestimating the gap between controlled demonstrations and practical deployment. The sheer engineering complexity drove many promising startups into bankruptcy as they exhausted funding before achieving commercially viable capabilities.
The fundamental barrier was high-dimensional uncertainty. A mobile manipulator must track its location, monitor dynamic objects, plan complex movements, and maintain stability, all while processing noisy sensor data in real-time. Until recently, no integrated platform could handle this combinatorial explosion of complexity, leaving truly capable general-purpose robotics perpetually out of reach. This explains why factory floors remained rigidly structured for decades: simplifying environments was the only viable approach when machines couldn't adapt to natural variation. As a result, entire industries remained dependent on human dexterity despite massive investments in automation, leading to a technological ceiling that appeared insurmountable until the convergence of advanced AI and hardware finally shattered these longstanding barriers.
2. Hardware's Glass Ceiling: Sensors and Actuators
Hardware lies at the heart of robotics' long-standing limitations. Even the most sophisticated prototypes were hamstrung by fragile sensors, inefficient actuators, and power systems that couldn't sustain extended operation. These hardware constraints weren't just technical hurdles – they formed an impenetrable ceiling that relegated robots to sterile, structured workspaces and tightly orchestrated production lines, preventing the emergence of the versatile, adaptable machines needed for real-world applications.
2.1. Sensors
For robots to match human capabilities, they need sensor performance rivaling our own biological systems. While recent years have seen incremental improvements in machine vision and tactile sensing, the majority of sensor technology remained too limited, costly, or fragile for large-scale deployment.
2.1.1. Vision
Video: Why Computer Vision is a Hard Problem for AI
Vision systems, the cornerstone of perception, faced three critical limitations. First, camera hardware struggled with resolution, field of view, and latency. While humans seamlessly integrate peripheral and foveal vision, robots required multiple cameras for comparable coverage – driving up cost, power consumption, and processing overhead. Second, depth sensing remained a persistent challenge. Early 3D sensors were bulky and unreliable, forcing engineers to cobble together combinations of stereo cameras, structured light sensors, and LIDARs – each with significant trade-offs. Finally, real-world conditions like rain, snow, and dust significantly impacted the quality of visual data, affecting robots’ ability to interpret their surroundings.
2.1.2. Touch and Tactile
Video: Tactile Sensing Lecture
The gap between human and robot touch sensing was even more stark. Human hands contain thousands of mechanoreceptors providing continuous feedback on texture, pressure, and temperature. Replicating this sensory network proved extraordinarily difficult. High-density tactile arrays remained prohibitively expensive and fragile, while attempts at "robot skin" failed under normal wear and environmental stresses. Even when partial solutions emerged, they generated torrents of data that overwhelmed early processing systems. This absence of reliable tactile feedback forced robots to rely almost exclusively on vision and crude force sensing, making fine manipulation virtually impossible.
2.1.3. Proprioception
Video: Motion Capture vs Proprioception
Proprioception – the internal sense of body configuration and movement – represents another crucial capability that early robotics couldn't replicate. While humans possess specialized receptors throughout our musculoskeletal system, robots relied on basic accelerometers, gyros, and joint encoders prone to noise and drift. This limited sensor suite made precise balance and dynamic manipulation difficult, especially when robots needed to adjust their center of gravity while handling objects. High-fidelity proprioceptive systems existed but remained too expensive and power-hungry for practical deployment, forcing most designs to fall back on heavily scripted movements.
2.1.4. Force and Torque
Force and torque sensing provides critical feedback for physical interaction. Multi-axis force/torque (F/T) sensors were sensitive, fragile, and required constant recalibration. Their bandwidth limitations often forced compromises in joint design, while their high maintenance burden restricted them to specialized applications. This left most platforms with minimal force awareness – leading to clumsy manipulation, frequent hardware damage, and safety concerns for human-robot collaboration.
Video: Why You Need Torque Sensors
Collectively, these sensor limitations formed a formidable barrier to achieving human-level autonomy. Without reliable vision, touch, proprioception, and force sensing working in concert, robots remained confined to highly structured environments where their limited perception could be compensated for. Only recently have advances in sensor technology, coupled with breakthroughs in processing capabilities, begun to crack this longstanding ceiling.
Collectively, these sensor limitations formed a formidable barrier to achieving human-level autonomy. Without reliable vision, touch, proprioception, and force sensing working in concert, robots remained confined to highly structured environments where their limited perception could be compensated for. Only recently have advances in sensor technology, coupled with breakthroughs in processing capabilities, begun to crack this longstanding ceiling.
2.2. Actuators
Actuators are the muscles of a robot that convert energy into motion. Their limitations have arguably been the most rigid ceiling in robotics’ long pursuit of human-level dexterity. While sensors shape how robots perceive the world, actuators define their ability to act within it – and for decades, no actuator technology could match the power density, efficiency, and adaptability of human muscle tissue.
2.2.1. Power Density and Efficiency
The fundamental challenge was achieving strength without compromising mobility. Early humanoids faced an impossible dilemma: powerful but heavy hydraulic systems that drained energy reserves, or lightweight electric motors that lacked the torque for dynamic movement. Boston Dynamics' Atlas demonstrated the trade-offs perfectly – its hydraulic actuators enabled impressive demonstrations but required a massive power pack that limited practical deployment.
Video: Hydraulic vs Electric Actuation in Robotics
The efficiency problem compounded these limitations. Every watt wasted as heat meant larger cooling systems and heavier battery packs, creating a vicious cycle that kept robots tethered to external power sources or limited to brief demonstrations. This energy constraint alone made continuous operation impossible, forcing teams to compromise between power and endurance in ways that human workers never face.
2.2.2. Compliance and Safety
Even with sufficient power, rigid actuators proved dangerously unsuited for human environments. The slightest miscalculation in force control could damage hardware or injure nearby workers. While humans naturally adapt their muscle tension to different tasks, robots needed complex mechanical and software solutions to achieve similar compliance.
Traditional actuators – whether electric or hydraulic – lacked inherent "backdrivability”, the ability to yield when encountering resistance. Series elastic elements offered a partial solution but introduced their own challenges in control precision and durability. Without reliable compliance, robots remained confined to safety cages or forced to move so cautiously that their practical value evaporated.
2.2.3. Durability and Cost Effectiveness
The final barrier was economic: actuators robust enough for continuous operation remained prohibitively expensive for mass deployment. Soft tissues and high-precision components degraded quickly under real-world stress, while more durable alternatives sacrificed the fine control needed for complex manipulation.
Video: The Power of Soft Robotics
This cost barrier created a paralyzing feedback loop: low production volumes kept prices high, which limited adoption, which in turn prevented the economies of scale needed to make better actuators affordable. Until this cycle could be broken, the dream of ubiquitous robotics remained economically unfeasible, regardless of other technological advances.
The actuator problem proved as fundamental as the sensor challenge: even perfect perception remains useless if a robot can't move fluidly, handle objects securely, or operate for more than a few minutes without failing. Whether from insufficient power density, lack of compliance, or prohibitive costs, these hardware limitations were enough to stall early robotics efforts before they could even tackle advanced software challenges.
3. Software Bottleneck: From AI to Infrastructure
Even if perfect hardware had appeared overnight, robotics would have remained trapped by software limitations. The field struggled across three critical domains: adaptive intelligence (Physical AI), deployment platforms (infrastructure), and professional-grade tooling (ecosystem). These software gaps made it difficult for robots to effectively perceive and interact with the world.
3.1. Physical AI
Physical AI represents the intelligence layer that enables robots to perceive, reason about, and operate in unstructured real-world settings. Traditional robotics faced fundamental constraints in sensing, planning, and control that limited adaptation to complex, dynamic environments.
3.1.1. AI Models
The AI architecture powering most robots reflected a critical misunderstanding of what true autonomy requires. Instead of unified intelligence, teams cobbled together constellations of small, specialized models, each trained for an isolated task or specific sensor type. A typical autonomous vehicle might deploy separate neural networks meticulously tuned for front, side, and rear cameras, stitching their outputs together with brittle rule-based logic. This disjointed design created sharp discontinuities in robot behavior during critical moments. A vehicle might recognize pedestrians from the front camera but fail when they appeared in peripheral views, making it impossible to handle novel scenarios or generalize learning across interconnected real-world situations.
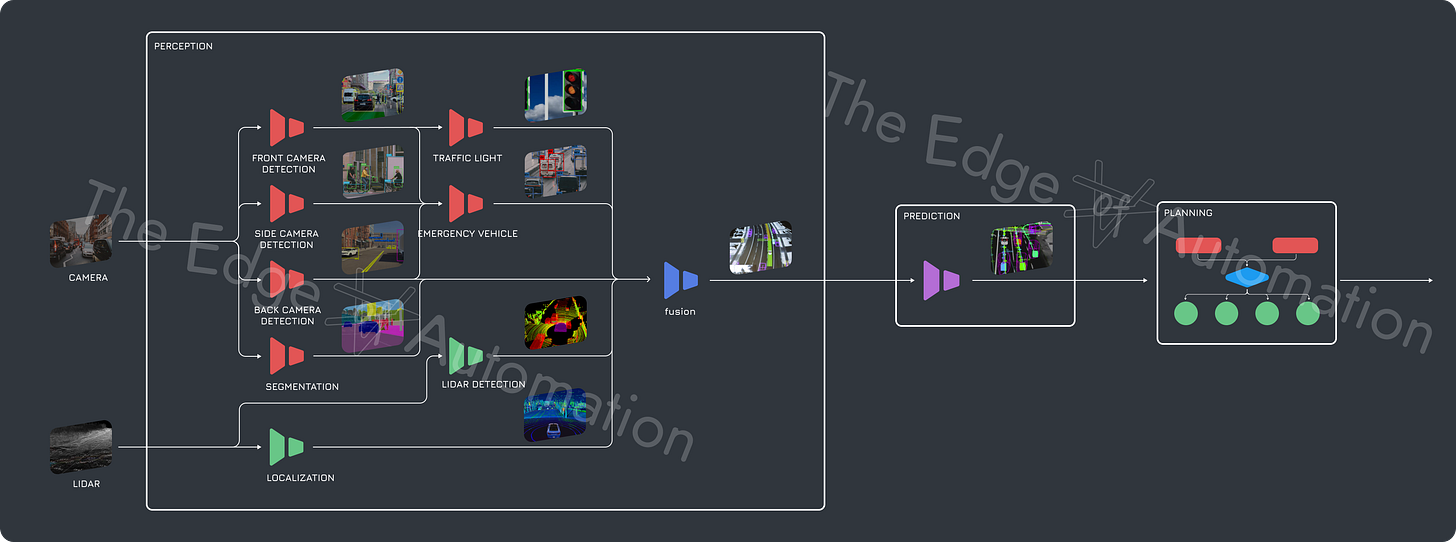
This architectural fragmentation spawned a maintenance nightmare that devoured engineering resources and created paralyzing technical debt. Each specialized model required its own training pipeline, evaluation metrics, and deployment infrastructure. Dependencies between systems grew exponentially complex, with updates to one component frequently causing cascading failures across seemingly unrelated modules. Teams found themselves trapped in endless cycles of retraining and recalibration, debugging interactions between models rather than advancing core capabilities. The result was robots that fell apart when facing the fluid challenges of cluttered human environments – precisely the scenarios defining useful real-world deployment.
The problem penetrated deeper than suboptimal architectural choices. While the broader AI community moved toward large foundation models capable of handling multiple modalities, robotics remained stuck in an outdated paradigm of narrow, task-specific networks. This isolation from mainstream AI progress proved costly as innovative architectures revolutionized fields from computer vision to natural language processing, resulting in a widening capability gap that left robotics years behind the cutting edge. While robots required the most sophisticated reasoning to navigate physical reality, they were paradoxically equipped with the least advanced AI models available, virtually guaranteeing their inability to achieve human-level adaptability.
3.1.2. Data
The data problem proved equally crippling. Unlike web companies that could harvest millions of user interactions with minimal cost, robotics teams faced formidable barriers to collecting the diverse, high-quality data needed to train robust AI models. Physical data gathering demanded operating expensive, fragile hardware in real environments – a slow and resource-intensive process that yielded relatively small datasets. This limitation was exacerbated by the industry's fragmentation: without standardized sensor configurations or shared data formats, even the limited data collected remained siloed within individual research groups or companies, preventing the pooling that might have accelerated progress.
Video: Can Robotics Overcome Its Data Scarcity Problem?
This data scarcity hit hardest when attempting sophisticated learning approaches like reinforcement learning, which might require billions of interactions to master complex tasks. The absence of high-fidelity simulation frameworks left teams unable to supplement scarce real-world data with synthetic examples. With no viable path to acquire sufficient training data, most robots defaulted to hand-crafted rules or simple models trained on woefully inadequate datasets. Data poverty condemned early robotics systems to rigid, preprogrammed behaviors that collapsed when confronting the complexity of real-world environments, creating a seemingly insurmountable barrier to developing machines with genuine adaptability.
3.1.3. Deployment
Even when teams managed to develop working AI models, they faced a final hurdle: no mature platform existed for shipping complex intelligence onto physical robots. ROS (Robot Operating System) promised a universal framework but exposed critical flaws in practice. Its TCP-based interprocess communication architecture induced latency spikes and memory thrashing, while attempts to optimize through "nodelets" introduced new layers of bugs and complexity.
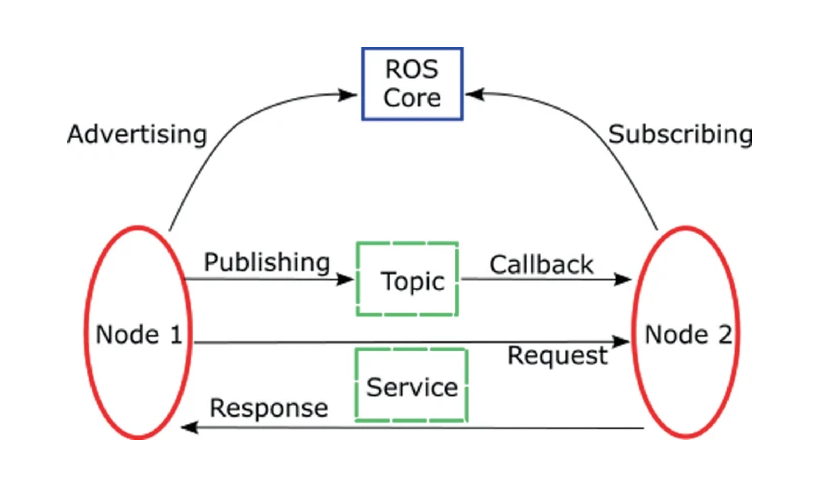
The containerization wave that revolutionized web services actually compounded these problems in robotics. Hardware drivers frequently broke inside containers, forcing teams to adopt brittle workarounds. Container images ballooned to tens of gigabytes as they accumulated NVIDIA drivers, CUDA libraries, and other dependencies. Without a robust, standardized platform, serious robotics companies either forked ROS into proprietary variants or abandoned it entirely – further fragmenting the ecosystem and preventing the emergence of shared best practices.
3.2. Infrastructure
Beyond AI models and algorithms, robotics demanded robust infrastructure to support deployment at scale – yet this foundation remained conspicuously absent. Three critical gaps prevented even promising prototypes from reaching commercial viability: the inability to port software across platforms, insufficient compute power for real-time operation, and unreliable hardware interfaces that made integration nearly impossible.
3.2.1. Software Portability
The dream of modular, reusable robotics software collided with harsh reality: even simple components proved nightmarishly difficult to port between platforms. Each robot required a unique combination of sensor configurations, hardware drivers, and control systems. What worked perfectly in one setting would fail catastrophically in another, forcing teams to rebuild basic functionality from scratch with every new deployment.
Video: Installing Sfotware and Preparing the Robot (Highlights Challenges in Software Portability)
Open-source efforts like ROS promised standardization but fell short in practice. Their packages, designed primarily for research and hobbyist use, buckled under commercial demands. Some companies responded by building proprietary frameworks, but this only accelerated fragmentation. Dependency management became a nightmare, and any attempt to upgrade one library would trigger cascade failures across entire software stack. The field became trapped in an endless cycle of rewrites and incompatibilities that made scaling impossible.
3.2.2. Compute
Video: Efficient Computing for Low-Energy Robotics
Real-time processing of sensor data and AI models demanded massive computational power, yet mobile robots couldn't afford the size, weight, or energy costs of traditional high-performance computing. Standard GPUs drained batteries in minutes while generating enough heat to require bulky cooling systems. The absence of powerful robotics processors meant teams had to choose between underpowered capabilities or power-hungry server components never designed for mobile use. This compute bottleneck effectively capped the sophistication of onboard AI, keeping robots from achieving the fluid intelligence needed for human-like operation.
3.2.3. Drivers and Hardware Interfaces
The software interface between high-level control and physical hardware remained perpetually unstable. Hardware vendors provided poorly maintained, proprietary drivers that lacked modern features and documentation. Critical tasks like sensor calibration or motor tuning became multi-week ordeals requiring deep expertise in vendor-specific protocols.
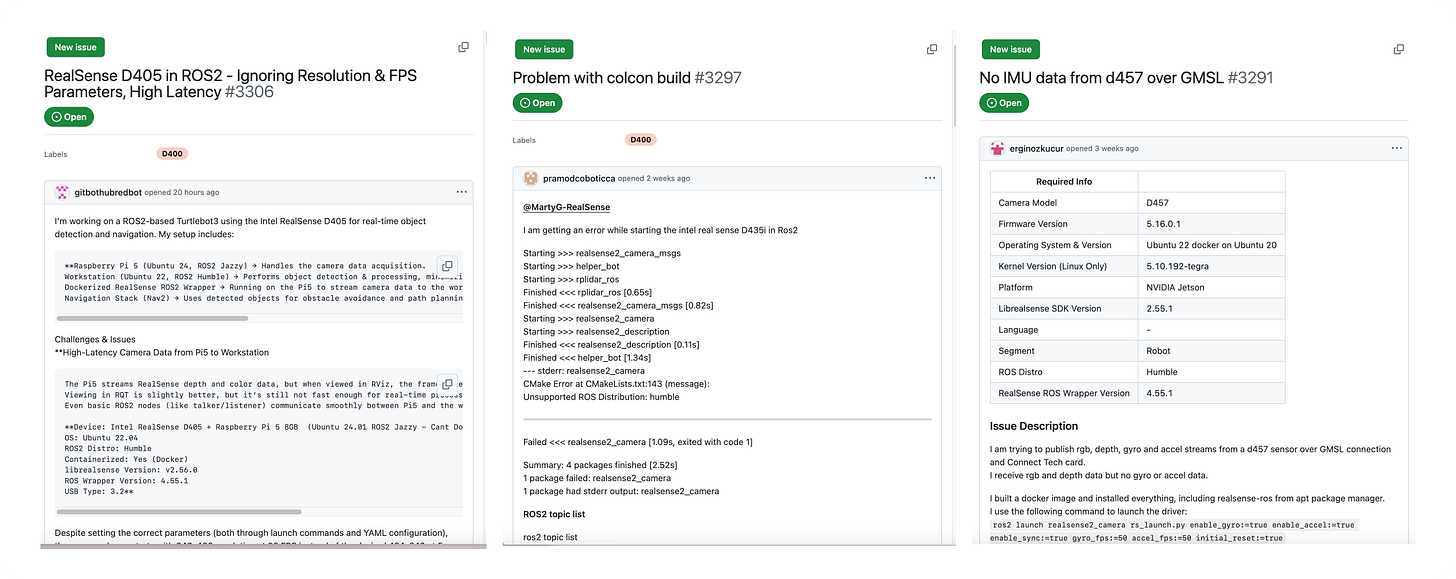
When firmware updates inevitably broke existing integrations, teams had to reverse-engineer interfaces or write new drivers from scratch. This fragile layer demanded constant maintenance, draining the already-scarce resources of robotics companies. Without reliable ways to interface with hardware, even the most sophisticated AI remained trapped in imagination.
3.3. Ecosystem and Tooling
Beyond core technology challenges, robotics suffered from a severely underdeveloped ecosystem of development tools. This gap didn't just slow progress – it systematically prevented the field from achieving the reliability and scalability needed for commercial deployment.
3.3.1. High Stakes, Low Quality
The inherent physical risks of robotics demanded enterprise-grade software quality, yet the field's tooling remained perpetually immature. Most open-source packages emerged from research labs or hobbyist projects, lacking systematic testing, documentation, or professional maintenance – a reality starkly illustrated by the near-total absence of unit tests in most robotics packages.
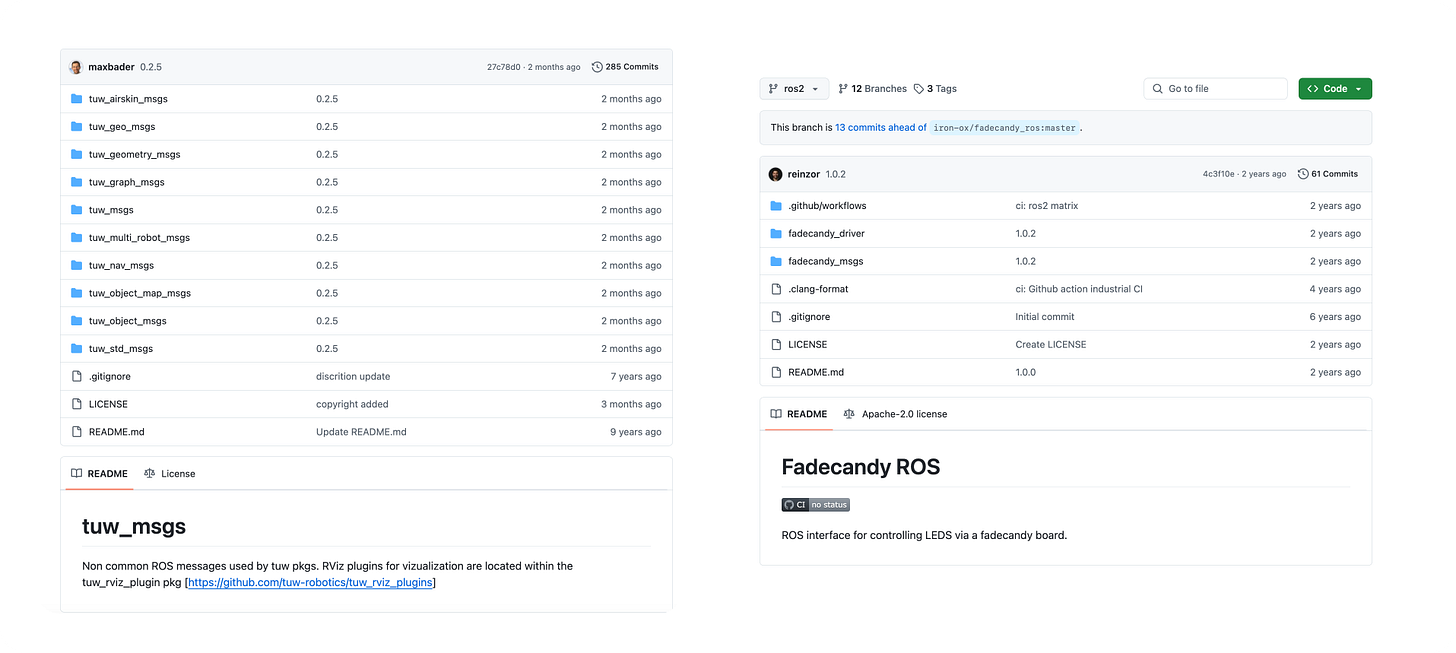
Without major tech companies fully committed to maintaining these projects, critical infrastructure remained dangerously unstable. When enterprises attempted to adopt these tools, they often discovered severe bugs only after months of integration work, forcing them to retreat into costly vertical integration.
3.3.2. Lack of Visualization and Observability
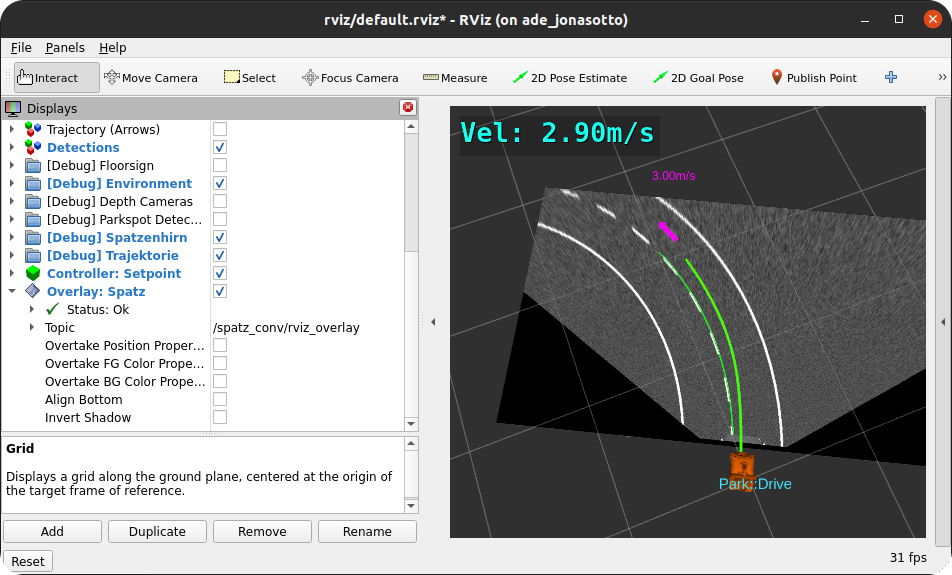
Developing autonomy systems requires interpreting complex streams of sensor data while diagnosing decisions made by multiple AI and control processes in real-time. Yet the field lacked robust tools for visualization, debugging, and system introspection. Popular frameworks like RViz offered only basic 3D overlays, while newer platforms like Foxglove missed crucial features for deep system observability until recently. This tooling gap made it challenging to effectively tune long-horizon action planners, diagnose sensor issues, or correlate complex system behaviors – turning basic development tasks into lengthy debugging ordeals.
3.3.3. Lack of Realistic Simulators
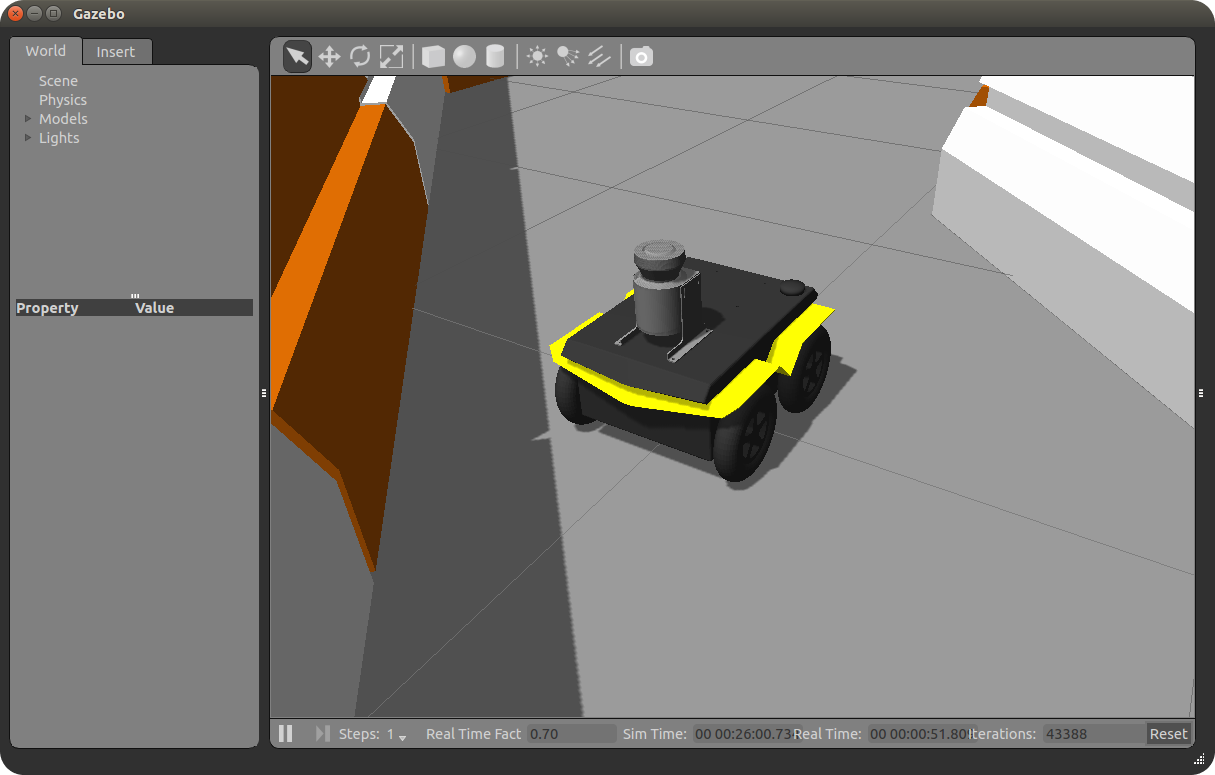
Perhaps the most crippling ecosystem gap was the absence of high-fidelity simulation platforms. While autonomous vehicles had partial solutions like CARLA and Applied Intuition, general-purpose robotics had no equivalent. Gazebo attempted to fill this role but lacked realistic physics, sophisticated sensor emulation, and industrial-grade stability. Unity's industrial simulation tools never reached the sophistication needed for serious AI development. Without reliable simulators, teams couldn't effectively use reinforcement learning or other data-intensive techniques, as real-world training proved too costly and dangerous. This limitation alone kept advanced AI approaches perpetually trapped in proof-of-concept stage.
The software bottleneck proved as fundamental as any hardware limitation in keeping robotics trapped in laboratories. No matter how sophisticated the mechanical platform, fragmented AI models and immature development tools made reliable deployment impossible. Only the recent emergence of unified intelligence architectures, robust simulation frameworks, and professional-grade infrastructure has finally shattered this ceiling, enabling the software maturity needed for true robotics.
4. Fragmented Landscape: Missing Industry Foundation
While other technologies like personal computers and smartphones flourished through shared standards, robotics remained trapped in a maze of incompatible hardware and software. The inability to establish standardized platforms is one of its most crippling structural failures.
4.1. Lack of Hardware Standardization
The absence of standardized hardware interfaces created a paralyzing feedback loop. Each manufacturer developed proprietary components – from custom motor controllers to specialized end effectors – that worked only within their ecosystem. Even basic elements like power supplies and communication protocols varied wildly between vendors. This fragmentation made it nearly impossible to mix components from different suppliers or upgrade individual parts without replacing entire subsystems.
The cost implications were severe. Without standardization, every new robot became a full-blown engineering project requiring custom adaptors, firmware modifications, and mechanical interfaces. The resulting complexity drove up both development time and unit costs, keeping robotics perpetually confined to specialized, high-margin applications. More fundamentally, the lack of standard hardware platforms prevented the emergence of the specialized component suppliers and economies of scale that had revolutionized other industries.
4.2. Lack of Software Compatibility
Software fragmentation proved equally damaging. Major manufacturers like ABB, Fanuc, and KUKA each maintained their own proprietary programming languages and development environments. Code written for one platform required complete rewrites to run on another, creating massive inefficiencies and preventing the emergence of a shared knowledge base. The open-source projects that attempted to bridge this gap were too academically focused and lacked the enterprise-grade reliability needed for commercial deployment
The absence of standardized software had far-reaching consequences. Instead of standing on the shoulders of giants, each team had to build their own ecosystem from scratch, draining resources that could have gone toward advancing Physical AI. This constant reinvention of basic functionalities prevented the formation of the shared development ecosystem that had accelerated progress in fields like web services and mobile applications.
This dual fragmentation of hardware and software created a systemic barrier to scaling. Without standardized platforms, innovations remained trapped in isolated silos, unable to spread through the broader community. The field became locked in a cycle where each new project started from scratch, preventing the accumulation of technological momentum. This lack of unified foundation kept robotics trapped as a collection of isolated achievements, forever short of evolving into an industry robots capable of delivering human-level autonomy.
5. Cultural Barriers: Why Innovation Stalled
Beyond technical hurdles, robotics was held back by deep-seated cultural limitations that systematically prevented innovation. The field never developed the commercial mindset needed to bridge the gap between prototypes and viable products. These cultural barriers proved especially damaging as they prevented the convergence of advances in hardware, software, and AI needed for general-purpose autonomy.
5.1. Lack of Product Focus
The robotics field remained trapped in a demonstration mindset, prioritizing minor achievements over commercial viability. Research labs and hobbyists built impressive prototypes that could fold laundry or fetch drinks but rarely developed product roadmaps addressing real market needs. Even well-funded projects like Willow Garage's PR2 lingered in demo mode until resources ran dry, leaving behind shelved experiments rather than industrial momentum.
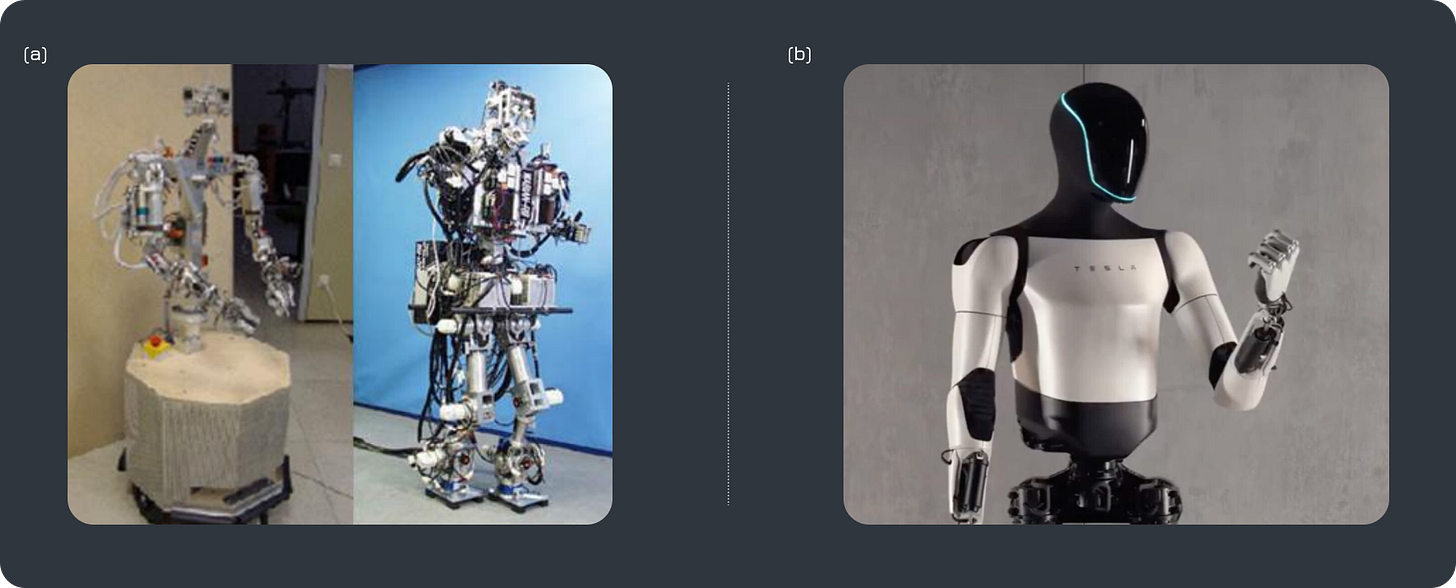
Boston Dynamics exemplified this pattern, pursuing ambitious visions of humanoid robots without a clear path to revenue. Their mesmerizing demonstrations felt more like science fair exhibitions than steps toward mass deployment. This stands in stark contrast to newer entrants like Tesla and Figure that prioritize sleek, user-friendly designs with a tangible goal: deploying robots people will actually buy.
5.2. Hardware-Bound Mindset
The field remained fixated on electromechanical perfection while neglecting the crucial AI layer needed for real-world adaptability. Founders with strong hardware backgrounds often treated software as an afterthought, assuming intelligence could be naturally added once physical systems were sound. This "build it and brains will follow" mentality repeatedly doomed projects, leaving them stranded without the AI to match.
Video: “Robots Are About Actuators, Not AI” (Incorrect)
Despite the clear rise of deep learning, teams were slow to embrace advanced software capabilities. When their machines ventured into unstructured environments, they quickly hit the limits of rigid rule-based control and underpowered AI models. The missing breakthroughs weren't about mechanical wizardry but enabling machines to perceive and reason their world.
5.3. Territorialism and Talent Deficiency
Robotics suffered from both a talent drain and cultural insularity. While building commercially-viable robots demands elite skills across mechanical, electrical, and software domains, the field struggled to attract and retain top-tier engineers. These specialists often gravitated toward better-funded roles in software, consumer electronics, or AI research at tech giants.
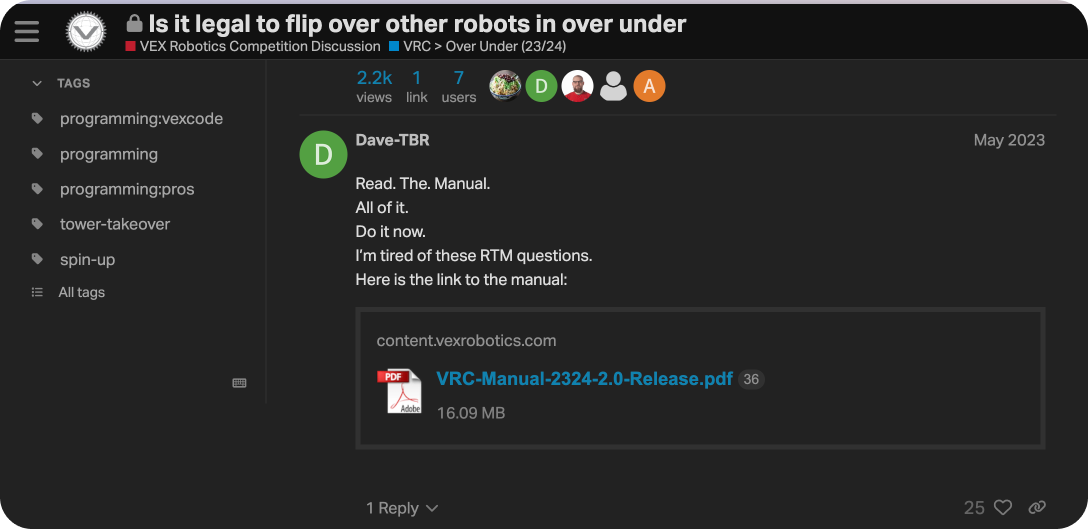
The old-guard robotics community's territorial attitude further compounded this problem. Ambitious software and AI specialists – crucial for next-generation autonomy – found the environment unwelcoming and moved on. Only recently has a fresh wave of interdisciplinary teams, often led by veterans from consumer hardware and AI powerhouses, begun attracting the world-class talent necessary for breakthrough innovation.
5.4. Misaligned Incentives
The financial ecosystem surrounding robotics created impossible tensions between long-term development needs and short-term return expectations. Investors, dazzled by viral demos, would funnel money into robotics startups only to demand rapid returns that clashed with the painstaking reality of building reliable machines. After years of sunk capital without revenue, these backers often withdrew, triggering boom-bust cycles that derailed promising research.
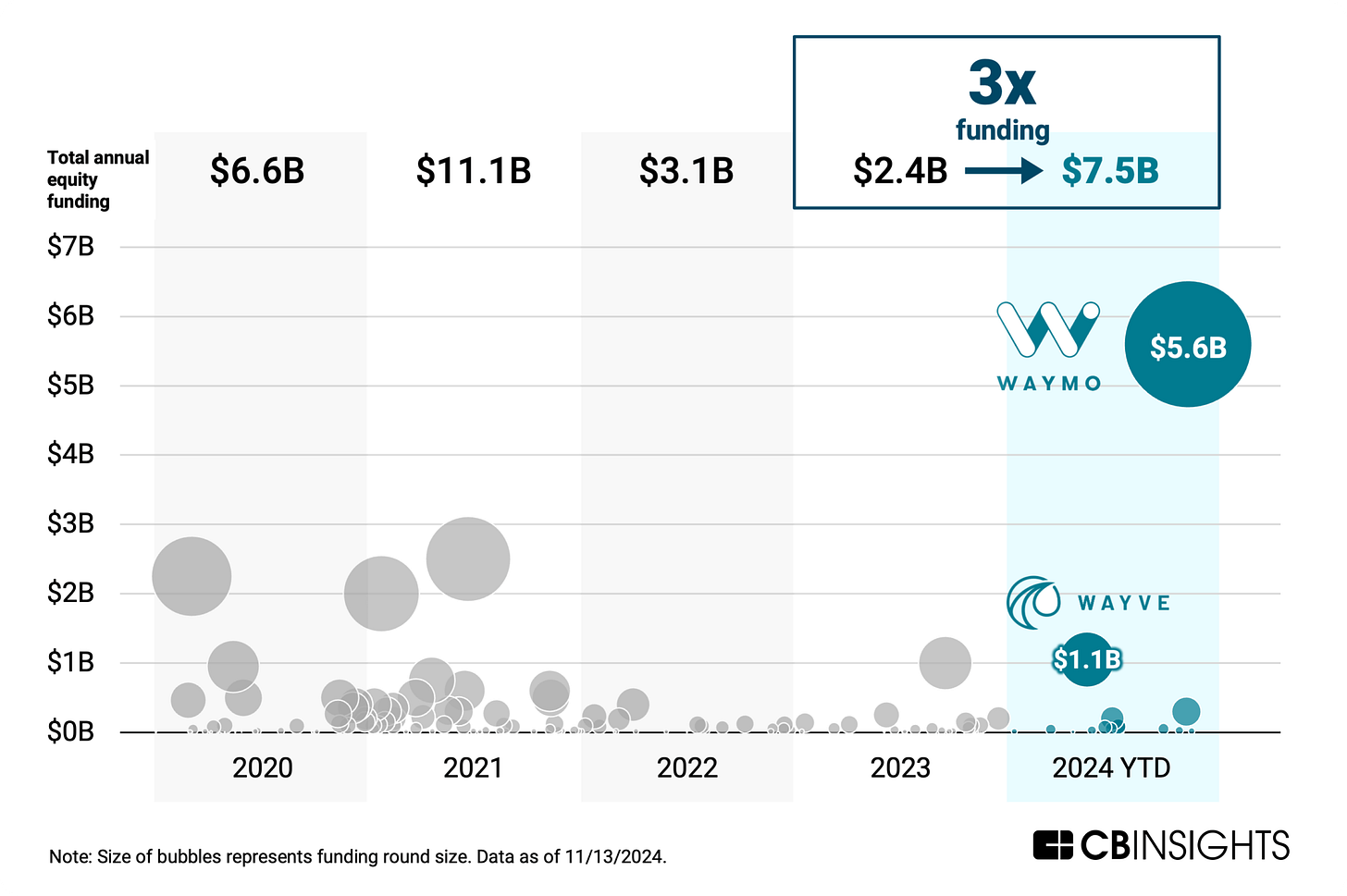
Corporate involvement complicated matters further. Major manufacturers sometimes treated robotics initiatives as hedging bets rather than core commitments. In some cases, success in robotics could undercut their existing business lines, creating unspoken incentives to keep ambitious autonomy projects on a tight leash. Without patient capital and coherent strategic backing, many promising ventures collapsed just when they needed the most resources to transition from prototype to product.
These cultural barriers – lack of product focus, hardware-centric thinking, territorialism, and misaligned incentives – proved as formidable as any technical challenge. The field remained fragmented and underpowered, surviving on sporadic sparks of interest rather than sustained collaboration. Only now, as global forces demand practical automation and AI finally offers robust perception and reasoning, have we seen the perfect storm of talent, capital, and ambition necessary to deliver on robotics' true promise.
IV. What’s Different Now: Gearing Up for Transformation
Despite billions in investment and countless breakthroughs, no one managed to create machines capable of matching human capabilities. Today, this stalemate is finally breaking. A perfect combination of breakthrough hardware, sophisticated AI architectures, and desperate market pressure has transformed robotics from perpetual research into imminent reality. What once seemed decades away now stands at the threshold of mass deployment.
1. Hardware for Human-Level Dexterity
Robots need hardware that matches our innate dexterity, resilience, and efficiency – a challenge that has, until recently, stalled every serious effort at general-purpose automation. Today, however, sensor and actuator technologies are finally catching up, driven by massive research investments and demanding product roadmaps. From high-fidelity vision and tactile arrays to power-dense electric actuators and flexible "robot muscles," we're witnessing the birth of a hardware ecosystem capable of supporting true physical AI.
1.1. Sensor Breakthroughs
Recent advances in vision, touch, proprioception, and force sensing have enabled machines to perceive and interact with unstructured environments at human-like fidelity. These capabilities shatter the constraints that once kept robots trapped in carefully controlled factory floors.
1.1.1. Vision
Vision systems have undergone a fundamental transformation, moving beyond simple cameras to integrated perception suites that rival human spatial awareness. Modern humanoids combine multiple sensor modalities – high-resolution cameras, structured light sensors, and compact LiDAR – to achieve comprehensive environmental understanding. Tesla's Optimus leverages automotive-grade vision systems for near-360° coverage, while Figure's latest platform integrates depth sensing and peripheral vision in a form factor suitable for mass production.
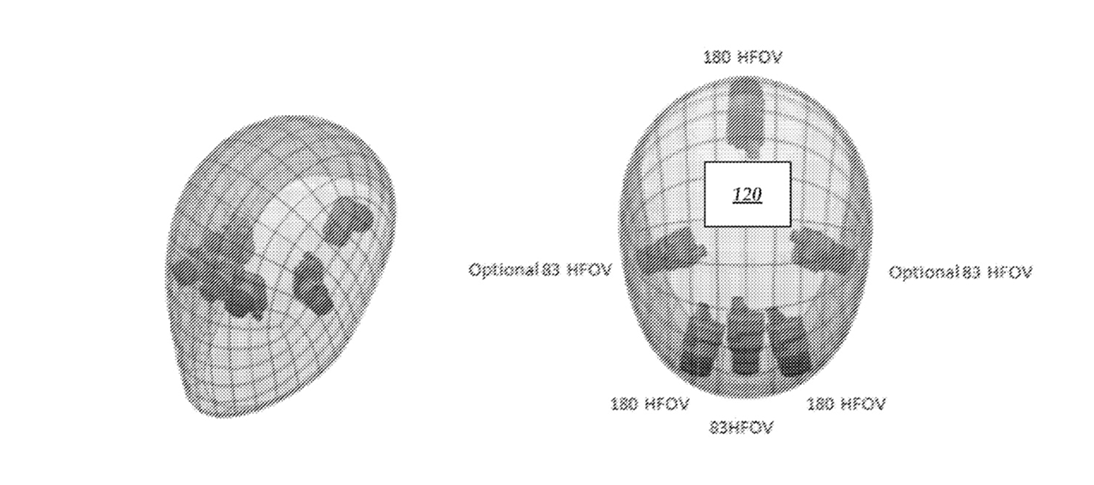
This leap in capability isn't just about better hardware. The integration of advanced AI processing enables robots to extract semantic meaning from raw visual data in real-time, understanding not just where objects are but how they can be manipulated. This fusion of robust sensors with sophisticated perception models enables the fluid, adaptive behavior essential for true labor replacement.
1.1.2. Touch and Tactile
The emergence of viable tactile sensing is another critical breakthrough. New optical tactile sensors transform touch into an imaging problem, enabling robots to detect subtle surface features, pressure distributions, and slip conditions. GelSight's evolution from lab prototype to commercial product exemplifies this shift – their latest sensors provide high-resolution contact mapping in robust, finger-sized packages suitable for industrial deployment.
More fundamentally, distributed tactile arrays are becoming standard features in advanced manipulators. Chinese firm PaXini's DexH13 hand incorporates thousands of integrated sensors, while Meta's ReSkin technology demonstrates how magnetic sensing can enable flexible, durable "electronic skin". These advances finally give robots the fine touch feedback needed for delicate manipulation tasks that were once exclusive to human workers.
1.1.3. Proprioception
Internal awareness – the ability to precisely track joint positions and body configuration – has evolved from a limiting factor into a solved engineering challenge. Modern humanoids integrate high-resolution encoders with distributed inertial measurement units (IMUs), enabling the kind of fluid, coordinated movement that human tasks demand. This proprioceptive foundation proves especially crucial for bipedal robots, where instant balance adjustments must account for changing loads and surfaces.
Video: UBTECH’s New Humanoid Robot, Walker S1
UBTECH's Walker demonstrates how mature this technology has become, with over 40 joints providing continuous force and position feedback. These systems maintain stability through rapid micro-adjustments, matching human-like adaptability in dynamic environments. The miniaturization and cost reduction of these sensors has transformed proprioception from an expensive luxury into a standard feature of commercial platforms.
1.1.4. Force and Torque
Force sensing completes the sensory foundation needed for human-level manipulation. Compact six-axis force/torque (F/T) sensors, once confined to research labs, now enable precise control of interaction forces in industrial settings. Tesla's Optimus uses this capability to manage variable loads during assembly tasks, while newer humanoids incorporate distributed force sensing throughout their structure for more natural human-robot collaboration.
This breakthrough in force perception enables "soft robotics" – machines that can yield appropriately when encountering resistance rather than rigidly following programmed paths. The ability to modulate force output while maintaining precision transforms robots from dangerous industrial equipment into collaborative tools suitable for shared human workspaces.
Advances in sensor technologies – vision, touch, proprioception, and force sensing – finally provides robots with the comprehensive environmental awareness needed to replace human labor. More importantly, these advances have crossed the threshold from research curiosities to robust, commercially viable systems suitable for mass production. This sensory foundation, combined with the actuator and compute breakthroughs we'll explore next, completes the hardware picture needed for general-purpose autonomy.
1.2. Actuator Breakthroughs
The quest for human-like motion has historically forced stark compromises between power, efficiency, and safety. Today's actuator revolution dismantles these trade-offs through innovations across multiple frontiers, enabling the fluid, adaptive movement essential for true labor replacement. This breakthrough spans four critical domains, each marking a decisive advance beyond traditional limitations.
1.2.1. Advances in Electric Actuators
The industry's shift to electric actuation signals more than a technical preference – it unites manufacturing scalability with performance levels once exclusive to hydraulic systems. Tesla's custom actuators for Optimus exemplify this shift: high-torque density motors paired with advanced thermal management enable burst power matching hydraulic systems while maintaining the reliability needed for continuous operation. Even Boston Dynamics, long wedded to hydraulics for dynamic movement, has announced a transition to electric drives, citing superior maintainability and control precision.
Video: Tesla’s Robot Actuators
This electric renaissance stems from breakthroughs in materials and manufacturing. New rare-earth magnetic compositions enable smaller, more powerful motors, while advances in power electronics deliver precise control at higher voltages. The result is actuators that can match human muscle performance while remaining light enough for mobile platforms – a cornerstone capability for viable humanoid deployment.
1.2.2. Mechanical Architecture Innovations
Innovations in mechanical architecture are overcoming the limitations of conventional rotary drives. Apptronik's breakthrough with linear actuators demonstrates how rethinking basic mechanical principles can yield transformative capabilities – their direct-drive approach delivers unprecedented force control while dramatically improving impact resistance.
Video: IMS Systems’ Archimedes Drive Transmission System
Even more revolutionary are approaches that completely reimagine power transmission. IMSystems' Archimedes Drive exemplifies this radical rethinking, eliminating traditional gearing in favor of rolling elements that achieve near-zero backlash with inherent shock tolerance. Chinese firm KGG Robotics’ novel planetary drive system similarly challenges convention by providing high load-bearing capacity and precise motion for humanoid robot joints such as the arms, legs, and hip joints.
These innovations address the durability and reliability demands of industrial deployment, where continuous operation in dynamic environments tests the limits of mechanical design. As these architectures mature and enter mass production, they're establishing the mechanical foundation needed for robots to escape the confines of carefully controlled factory floors.
1.2.3. Materials and Soft Robotics
Advanced materials are reshaping actuator design, pushing beyond incremental improvements to enable entirely new approaches to movement. The integration of carbon fiber composites and specialized metal alloys has transformed the core engineering equation: where traditional actuators demanded strict trade-offs between strength and weight, new materials enable unprecedented power density while enhancing thermal performance. This shift is particularly evident in humanoid platforms, where novel composites enable joint designs that match human biomechanics while maintaining the durability needed for industrial deployment.
Video: Shape-Shifting Soft Robots (MIT Seminar)
Soft robotics represents a paradigm shift from rigid mechanisms to bio-inspired designs. By leveraging elastomer-based artificial muscles and advanced materials, these systems can dynamically alter their mechanical properties like living tissue. Labs at MIT and Stanford have demonstrated capabilities previously impossible with traditional actuators – from delicate object manipulation to adaptive compliance in unstructured environments. While mass production remains a challenge, this technology promises robots that2 don't just move precisely but actively tune their physical characteristics to match task demands.
1.2.4. Elastic and Force-Controlled Actuators
Perhaps most crucial for human-robot collaboration is the mainstream adoption of Series Elastic Actuators (SEAs) and sophisticated force control. By incorporating spring elements and real-time force sensing, modern actuators can yield to unexpected contacts while maintaining precise position control. This inherent compliance enables robots to handle delicate tasks without risking damage or injury.
Video: Series Elastic Actuators (SEA)
Recent breakthroughs in backdrivable actuators have pushed these capabilities further. New mechanical architectures achieve natural compliance through innovative transmission designs that allow external forces to move the robot's joints with minimal resistance. This bidirectional force transparency – where the actuator can both apply precise force and respond fluidly to external forces – transforms how robots interact with their environment. Tasks that once required complex force feedback loops can now leverage the actuator's inherent mechanical properties.
The commercial impact is profound. Tesla's Optimus and Figure's humanoid platforms demonstrate how this sophisticated force control transforms robots from isolated industrial equipment into collaborative tools. Their actuators seamlessly handle both precision tasks and heavy lifting, establishing another milestone in creating machines that can replace human labor across diverse environments. This fusion of power with natural adaptability completes another vital piece of the hardware foundation enabling widespread robotics deployment.
1.3. On-Board Compute
The leap to true robotics demands not just better sensors and actuators, but also advances in edge computing. Where previous generations relied on off-board processing or cloud connectivity, modern humanoids integrate specialized AI accelerators that enable real-time perception and decision-making directly on the platform. This shift from tethered computing to autonomous intelligence marks another crucial milestone toward the birth of practical robotics.
Video: NVIDIA’s Jetson Thor Announcement
NVIDIA's Jetson Thor exemplifies this transformation, delivering teraflops of neural network performance in a form factor suitable for mobile robots. Tesla's approach with Optimus goes further, adapting their self-driving computer architecture to handle the heavy software workloads of a humanoid. These platforms don't just process data – they run sophisticated AI models that enable fluid adaptation to unstructured environments.
The implications extend beyond raw performance. By combining AI accelerators with efficient ARM processors and specialized robotics co-processors, manufacturers can now optimize for the delicate balance between computational power and energy consumption. This enables extended operation on battery power while maintaining the sophisticated capabilities – from real-time SLAM to natural language interaction – that define truly autonomous systems.
These breakthroughs in actuator technology shatter decades of compromises between power, precision, and adaptability. Where previous generations struggled with rigid, unsafe mechanisms unsuited for human environments, today's advances in motors, transmissions, and materials enable fluid movement without sacrificing reliability. The actuator barrier that long confined robotics to demonstrations has finally fallen.
2. Physical AI for Adaptive Intelligence
The leap from rigid automation to true robotics hinges on a revolutionary advance in machine intelligence. Physical AI fuses perception, reasoning, and action into fluid capabilities that match human adaptability, transforming robots from specialized tools into genuine replacements for labor.
2.1. Unified AI Models
The shift from fragmented, single-purpose AI models to unified architectures represents a fundamental breakthrough in robotics. By seamlessly integrating perception, planning, and control, these new systems enable the fluid adaptation to uncertainty that defines human-like intelligence – a decisive break from decades of rigidity.
2.1.1. Multimodality and Partial Inputs
Early robotics treated each sensor stream as an isolated problem, leading to systems that failed when any single input degraded. Modern architectures instead fuse diverse data sources – vision, touch, sound, force – into unified representations that remain robust even with partial information. Projects like DETR3D and BEVFormer pioneered this approach in autonomous vehicles, showing how neural networks could seamlessly combine camera feeds, LIDAR scans, and radar signals.
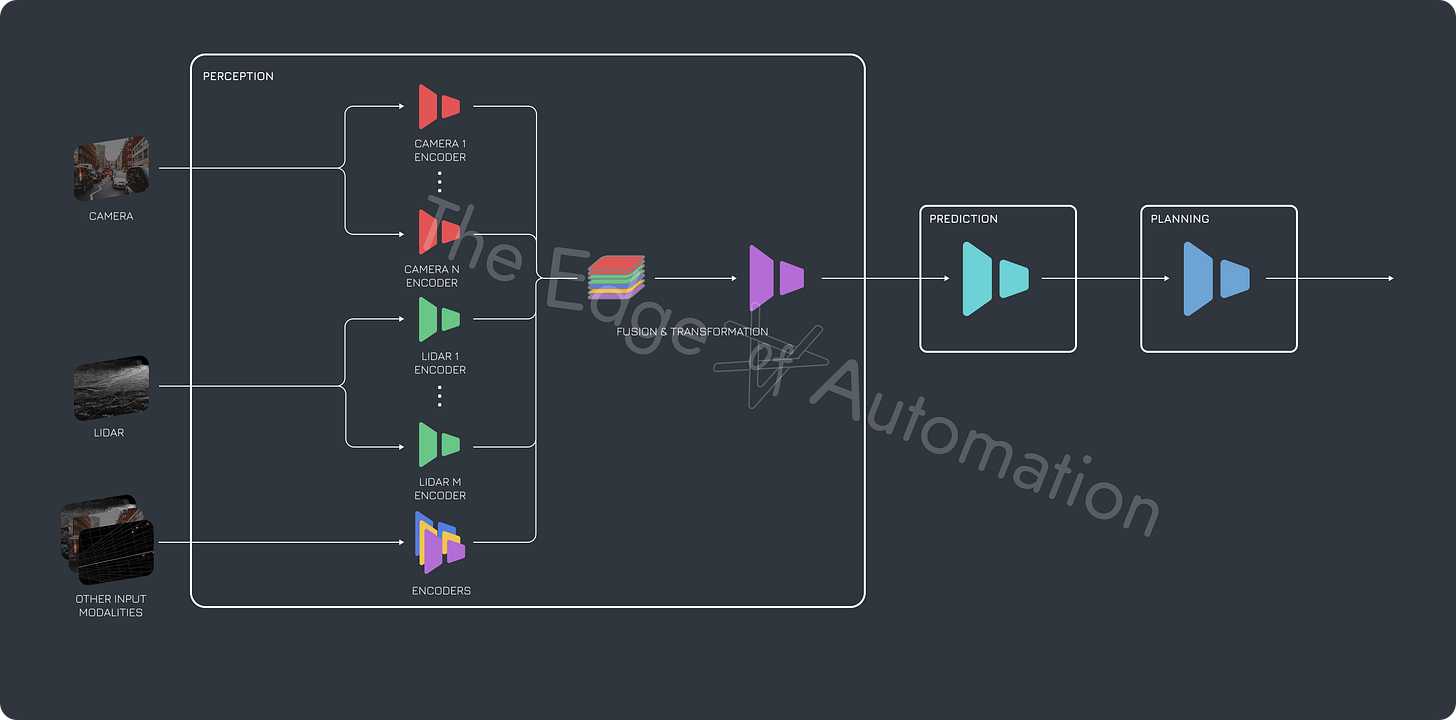
The implications reach far beyond improved perception. By training networks to operate with incomplete or noisy data, robots gain resilience previously impossible with traditional approaches. For example, a humanoid can maintain balance even if a joint sensor fails, or complete a grasping task despite occluded camera views. This adaptability to sensor degradation is a major step toward machines that can handle real-world uncertainty.
2.1.2. Foundation Models
The data requirements for robust multimodal AI would have been prohibitive under manual labeling approaches. Foundation models solve this through self-supervised learning at massive scale. Networks like BEVFusion and ProFusion3D are pre-trained on vast quantities of unlabeled sensor data, learning to predict missing modalities and identify consistent patterns across different inputs. Once pre-trained, these models can be efficiently fine-tuned for specific tasks using minimal additional data.
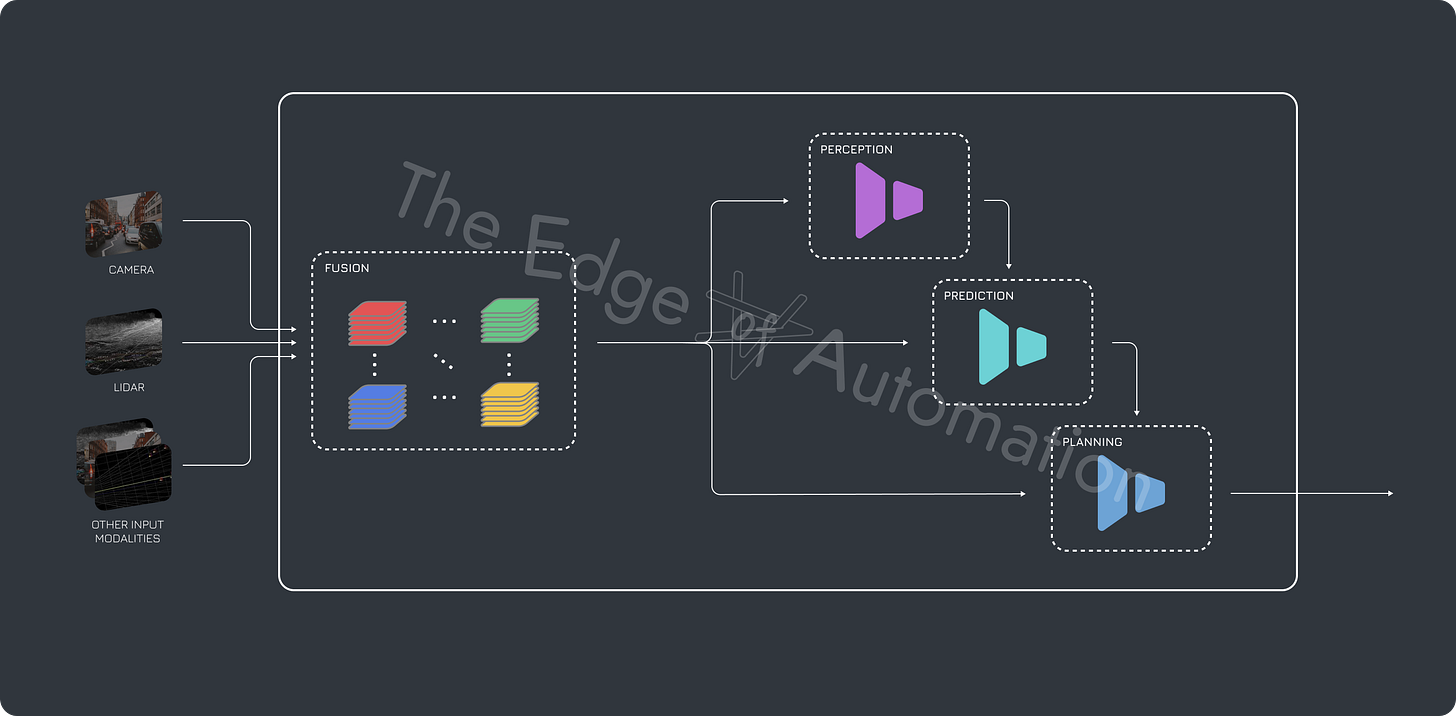
This approach dramatically reduces integration complexity. Rather than maintaining separate codebases for every sensor configuration, robotics teams can deploy a handful of foundational networks that adapt to new hardware through targeted fine-tuning. The economic implications are profound: development cycles shrink from years to months, while reliability improves through exposure to more diverse training scenarios.
2.1.3. Vision-Language-Action Models
The emergence of large language models catalyzed a new paradigm in robotics intelligence. Early systems like SayCan demonstrated how language models could break down complex commands into actionable sequences. More recent architectures like PALM-E and RT-2 go further, directly mapping natural language and visual inputs to motor actions. A robot can now interpret instructions like "sort these items by color" without explicit programming for each step.
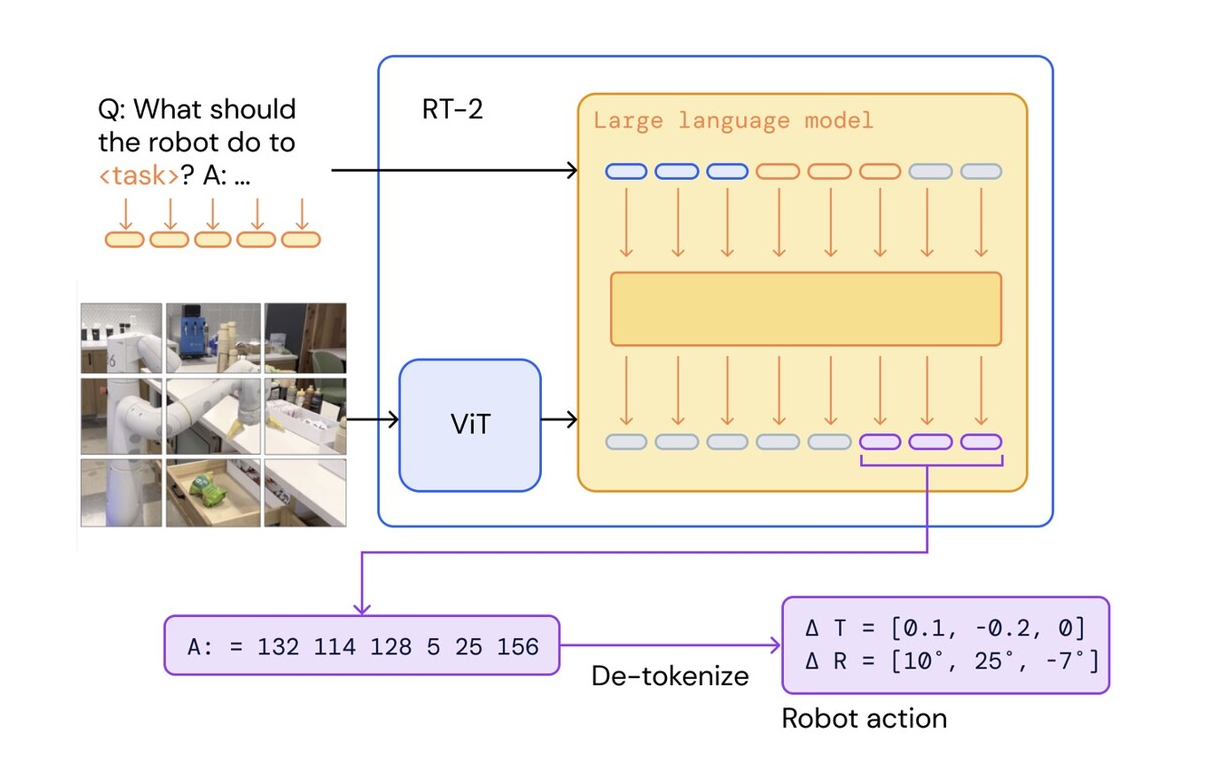
Vision-Language-Action (VLA) models represent a fundamental advance over traditional control hierarchies. By unifying perception, reasoning, and action generation in a single network, they enable fluid adaptation to novel situations. A humanoid equipped with well-designed VLA models can apply learned manipulation skills to unfamiliar objects or recombine known actions to solve new tasks – capabilities that were pipe dreams just a few years ago.
2.1.4. AI-Driven Control and Adaptability
Traditional approaches in low-level control relied on hand-tuned parameters and rigid control laws. Modern systems instead use AI policies trained on motion capture data and physics simulations to coordinate multiple joints simultaneously. Tesla's Optimus exemplifies this shift: its actuator controllers learn from millions of simulated interactions, automatically adjusting to different loads and surface conditions.
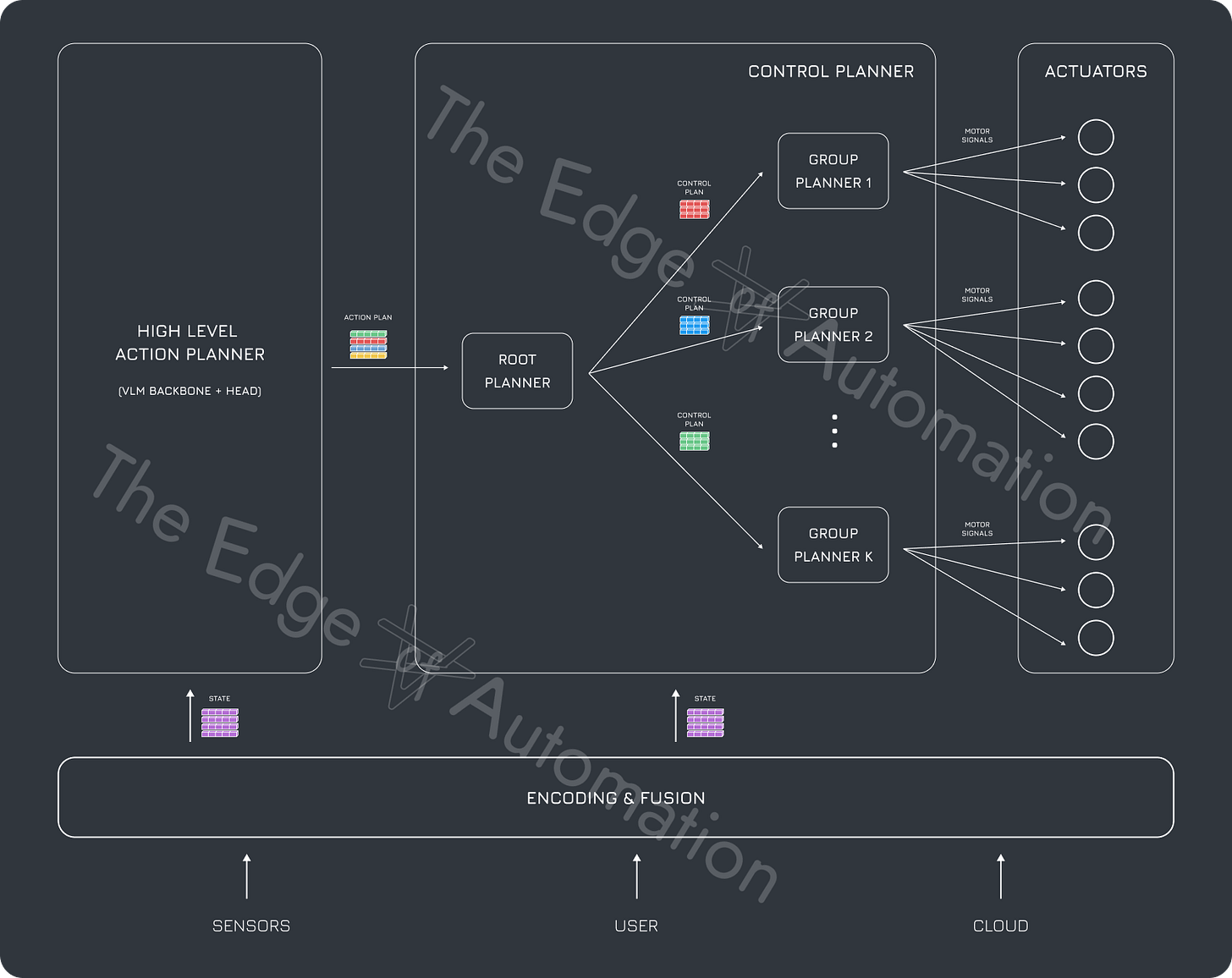
AI-driven control extends to real-time adaptation. Specialized microcontrollers can run local policies that modify joint damping and stiffness on the fly, while higher-level networks coordinate overall motion strategies. The result is robots that move with unprecedented fluidity and resilience, capable of recovering from disturbances that would have crashed earlier systems.
At Quintet AI, we build upon this foundation by offering plug-and-play AI models –from core perception engines to universal control policies, enabling clients to deploy robots at fraction of the time than is traditionally required.
Multimodal fusion, foundation models, VLA architectures, replace the brittle, unintelligent, and firmware-like software systems of traditional robotics. Robots can now perceive, reason about, and interact with their environment through unified intelligence frameworks that improve with experience.
2.2. Reasoning and Memory-Based Models
Even the most sophisticated multimodal models struggle with long-horizon tasks that require strategic planning and continuous adaptation. Reasoning and memory architectures fill this gap, enabling robots to maintain context across long sequences, correct errors mid-task, and learn from experience.
2.2.1. Test-Time Compute
The traditional emphasis on massive training-time computation overlooks a crucial insight: robots need real-time reasoning capabilities to handle dynamic environments. Test-time Compute (TTC) revolutionizes this paradigm by allocating substantial processing power during inference rather than just during training. This approach enables robots to simulate outcomes, verify decisions, and adapt plans on the fly – much like humans mentally rehearse actions before execution.
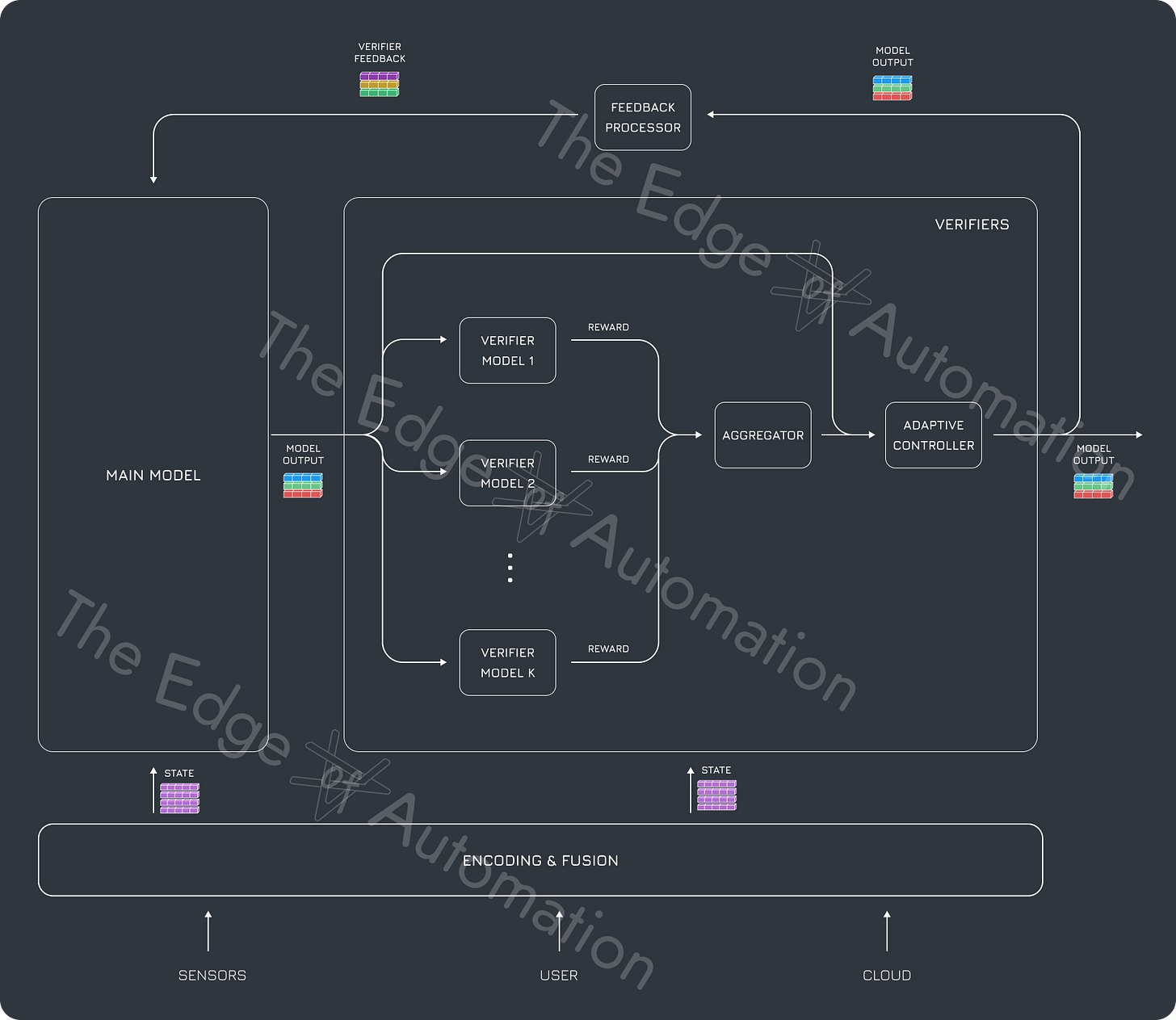
For example, a humanoid stacking boxes might run multiple forward simulations to test different grip positions or sequence variations, using a lightweight verifier model to catch potential failures before they occur. This real-time reasoning capability, achieved with models as small as 1.5B parameters (often at the expense of inference-time latency), shows a fundamental advancement over the traditional "act-then-correct" approaches.
2.2.2. Advanced AI Reasoners
Recent breakthroughs in AI architecture have eliminated the need for iterative verification by enabling sophisticated reasoning in a single forward pass. Models like DeepSeek-R1 demonstrate how careful combinations of supervised fine-tuning and reinforcement learning can produce high-level reasoning capabilities without relying on external verifiers or feedback loops. This architectural innovation adds momentum toward the creation of robots that can plan and execute complex tasks with unprecedented efficiency.
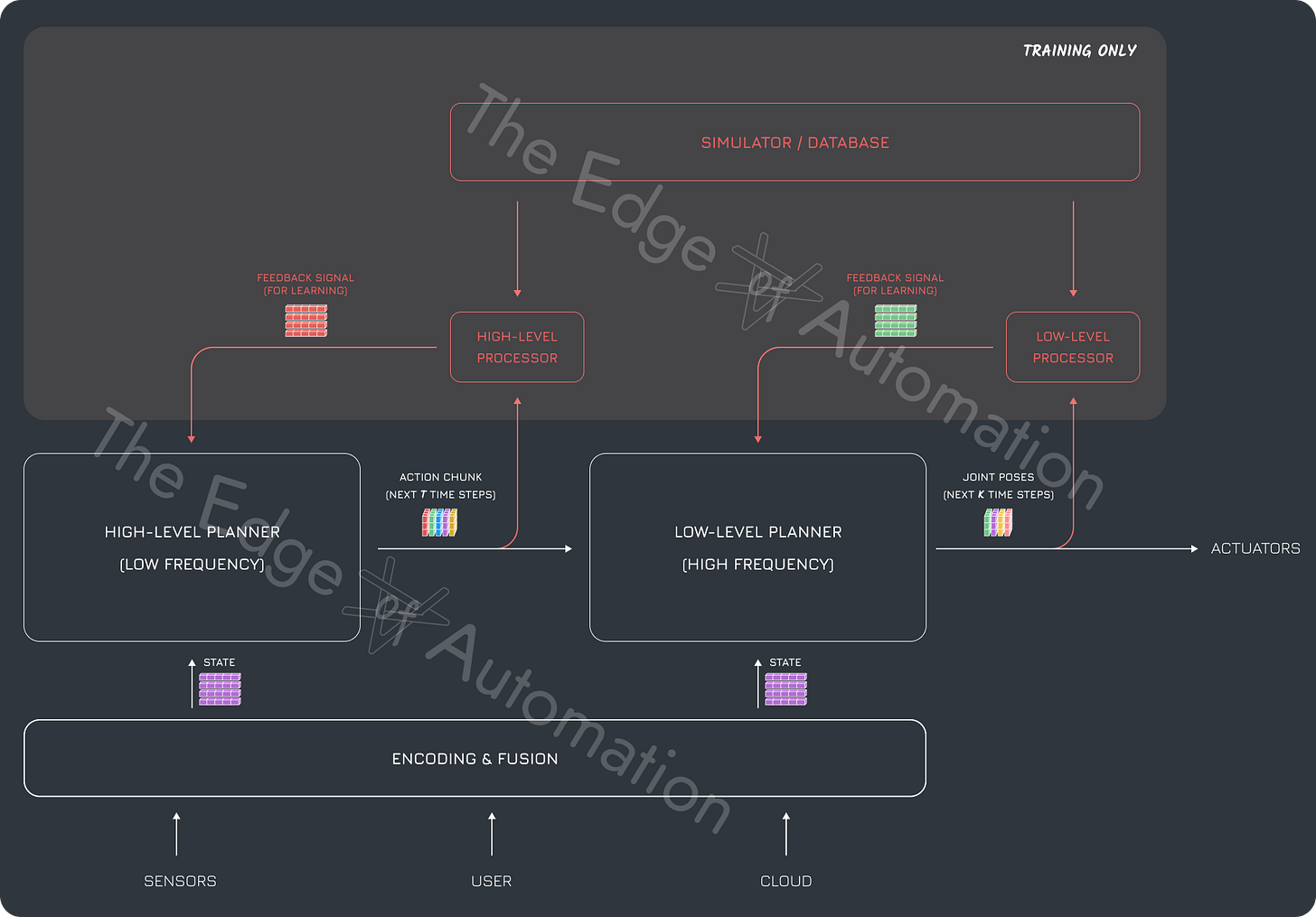
The impact on robotics proves particularly powerful through "action chunking" – where models directly output coordinated sequences of actions divided into achievable subgoals. Stanford's CoT-VLA is an example of such model. Rather than continuously replanning each movement, robots can generate coherent action plans that maintain flexibility while reducing computational overhead. This capability proves crucial for complex manipulation tasks, where fluid coordination between perception, planning, and motor control determines success or failure.
During training, these action chunks are refined against multiple objectives: matching desired robot states, aligning with human demonstrations, and optimizing for task completion. The result is systems that can decompose complex goals into executable sequences while maintaining adaptability to environmental changes. A humanoid tasked with organizing a workspace, for instance, can break down the overall objective into a series of coordinated movements – from clearing surfaces to proper item placement – while smoothly handling interruptions or unexpected obstacles. This breakthrough in planning efficiency, combined with advances in perception and control, marks a significant advancement toward robots that can truly replace human labor.
2.2.3. Chain of Affordances
The Chain-of-Affordances (CoA) framework transforms how robots interact with their environment by treating potential actions as an interconnected network. Each "affordance" – a possible interaction like opening a door or gripping a tool – becomes a building block that can be dynamically combined into complex behaviors, enabling robots to develop new capabilities through experience.
Video: Vision-Language-Action Model with a Chain of Affordances
This flexibility is essential for robots operating in unstructured environments. Rather than failing when encountering unfamiliar situations, CoA-equipped robots can adapt by combining known affordances in novel ways, similar to how humans instinctively adapt familiar skills to new challenges. This adaptability makes robots more effective in real-world applications where conditions frequently change and preset solutions may not suffice.
The most profound advance comes from systems that discover interaction possibilities through direct experience rather than human definition. While fixed industrial arms can rely on prescribed affordances, general purpose mobile manipulators demand more fluid understanding. Modern AI systems break this limitation through end-to-end affordance learning via reinforcement learning and self-supervised exploration. Combined with advanced simulation, this self-discovered knowledge enables intuitive environmental adaptation.
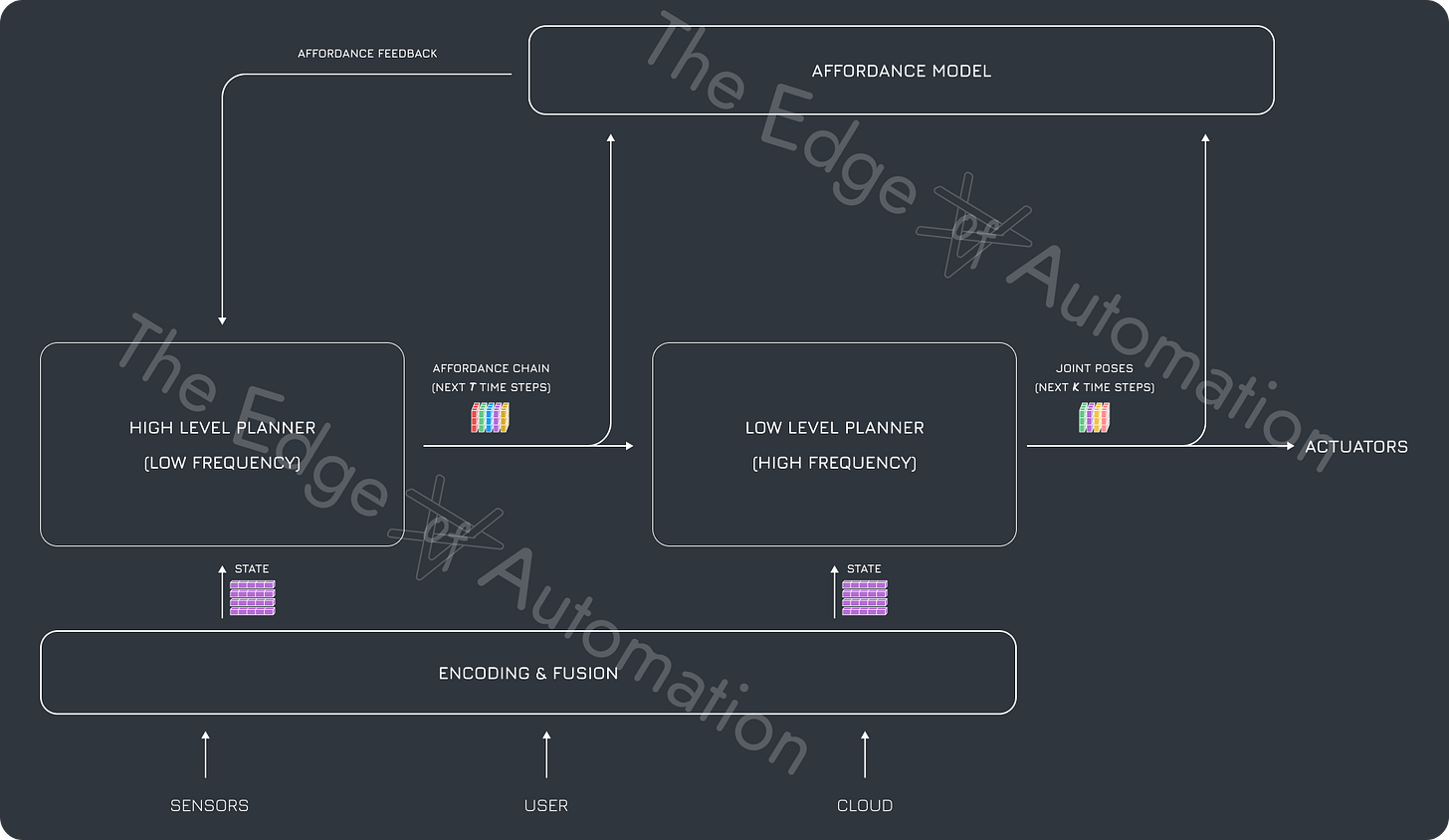
Quintet AI's affordance AI modules enable robots to autonomously chain complex sequences – from object manipulation to navigation to storage tasks – without requiring explicit programming for each step.
The CoA framework enhances human-robot collaboration by making robots more responsive to nuanced tasks. From high-precision surgery to dynamic manufacturing environments, CoA-driven systems are advancing automation toward greater contextual intelligence.
2.2.4. Memory for Long-Horizon Tasks
The final piece of advanced reasoning comes from architectural innovations in robot memory systems. While transformer models excel at processing immediate inputs, they struggle to maintain context across extended operations. New memory architectures solve this through specialized modules that track task progress, environmental changes, and learned experiences.
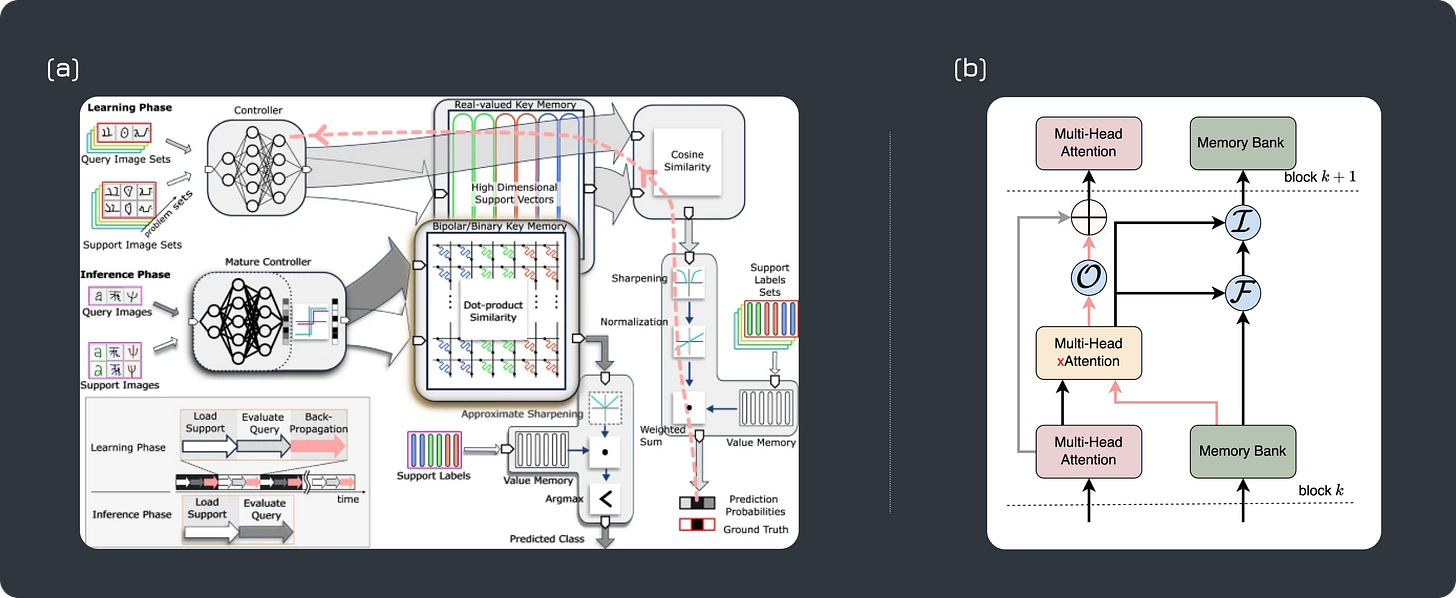
An example of a memory-based model is the Robust High-Dimensional Memory-Augmented Neural Networks (HD-MANN), which improves learning efficiency by integrating external memory modules for high-dimensional embeddings, reducing data needs and training time. Similarly, the Large Memory Model (LM2) enhances Transformer performance in multi-step reasoning and long-context synthesis. With an auxiliary memory module and gated updates, LM2 effectively captures long-term dependencies in sequential data, improving adaptability and retention.
These systems demonstrate how memory-based models enable robots to handle complex sequences without losing track of overall goals or previous actions . A warehouse robot organizing inventory can seamlessly resume after interruptions, remember which areas have been processed, and maintain consistency across shifts – capabilities essential for true automation of human-level work.
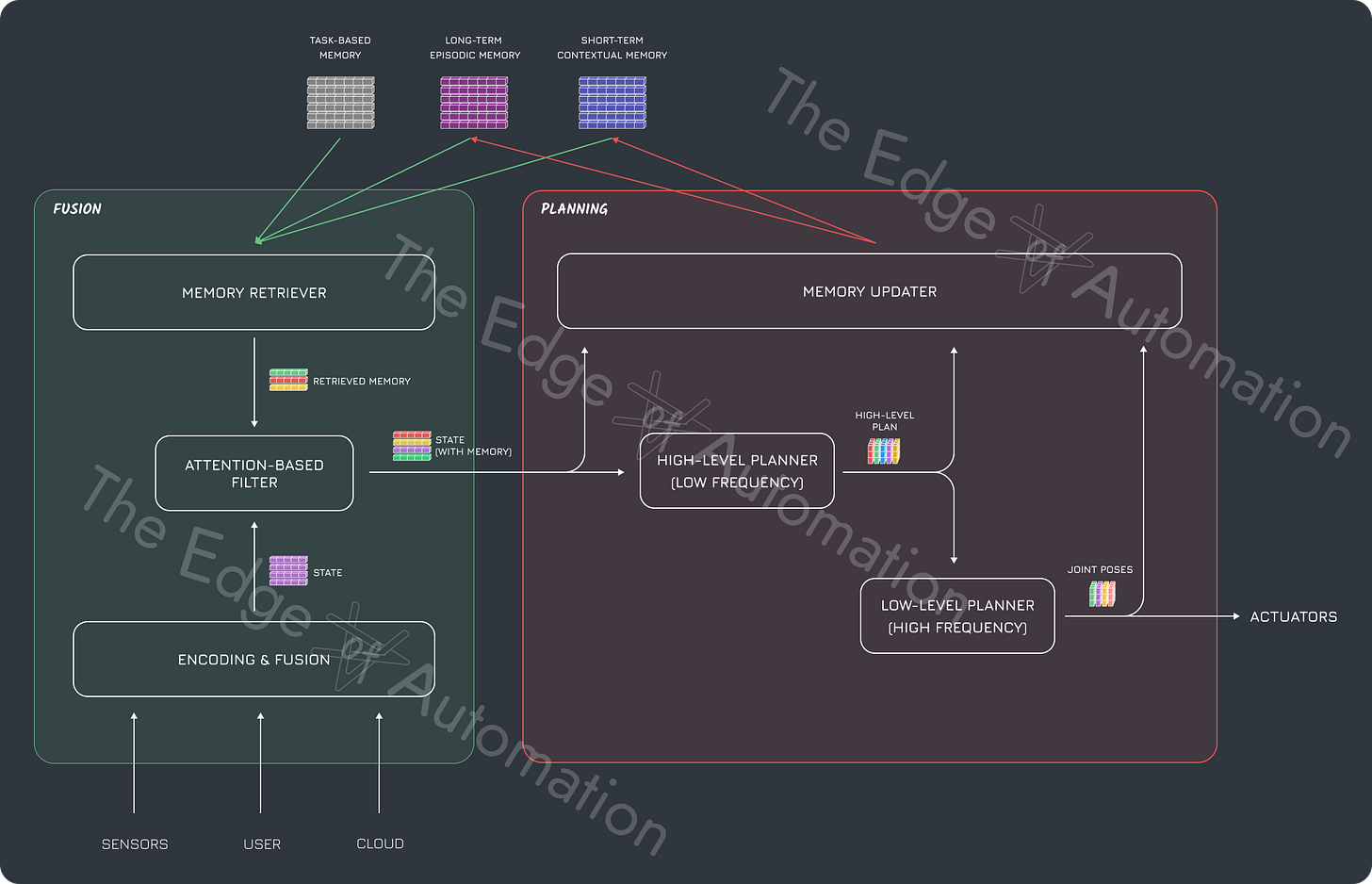
At Quintet AI, our memory-based models enable robots to handle extended sequences without losing track of subtle state changes or incomplete steps. Our latest innovation, a model that "learns to remember" during inference, has dramatically improved the reliability of humanoids and factory robots in multi-stage tasks like inventory management and assembly.
Reasoning and memory architectures transform robots from reactive machines into genuine problem-solvers. By unifying advanced planning, chain-of-affordances, and dynamic memory management, today's robots can maintain awareness across complex tasks while adapting to novel situations. This leap in cognitive architecture, combined with advances in perception and control, puts us firmly on the path toward machines that can truly replace human labor.
2.3. Mapping Human Activities
The leap from controlled automation to true robotics demands machines that can replicate the nuanced physical intelligence humans take for granted. Beyond perception and reasoning, robots must inherit our embodied knowledge – the countless micro-adjustments and learned behaviors that make tasks like screwing a bolt or folding fabric appear effortless. This capability to map and reproduce human activities is an essential step toward true autonomy.
2.3.1. Imitation Learning
Imitation learning represents a fundamental breakthrough in how robots acquire human-like capabilities. Rather than programming every possible movement or crafting complex reward functions, modern systems can learn directly from human demonstrations – whether through teleoperation, motion capture, or direct observation. Tesla's approach with Optimus exemplifies this paradigm: operators demonstrate assembly tasks through natural movements, building a rich dataset that trains increasingly sophisticated control policies.
Video: Imitation Learning Example (Stanford HumanPLUS)
The implications extend far beyond simple mimicry. Through techniques like inverse reinforcement learning, robots extract the underlying principles driving human choices – why we adjust grip pressure when handling delicate objects or modify our approach angle when navigating cluttered spaces. This deeper understanding enables generalization to novel situations, a crucial capability for real-world deployment. A robot that learns to grasp cups through demonstration can apply those principles to unfamiliar containers, while systems trained on basic assembly tasks can adapt their learned skills to new product variants.
This breakthrough in skill acquisition transforms how we deploy robots in unstructured environments. Tasks that once required months of careful programming – from precision electronics assembly to handling soft materials like clothing – can now be taught through natural demonstration. More importantly, these learned behaviors can be decomposed into reusable primitives, enabling robots to combine and adapt their skills for novel challenges. The result is machines that don't just replicate specific actions but develop genuine physical intelligence, marking another crucial step toward true labor replacement.
2.3.2. Network of Task-Specific Models
The true power of Physical AI emerges when specialized task-specific models combine into comprehensive robot capabilities. Through careful orchestration, systems can wire together distinct skills – from bipedal locomotion to dexterous manipulation – creating adaptable sequences that handle real-world complexity without explicit programming for every scenario. This enables smooth transitions between tasks while maintaining coherent behavior over extended operations, even in dynamic environments where conditions constantly shift.
The architecture typically employs multi-level planning, where high-level models decompose complex objectives into actionable sequences while specialized planners handle specific tasks. A warehouse robot unloading trucks might seamlessly transition between walking, lifting, and precise placement – each action handled by dedicated models that convert sensory inputs and internal state into specific motor commands. This hierarchical approach enables robots to tackle sophisticated workflows that would overwhelm monolithic systems, while maintaining the precision and reliability that industrial deployment demands. The separation of planning levels also allows for rapid adaptation when unexpected obstacles or changes arise.
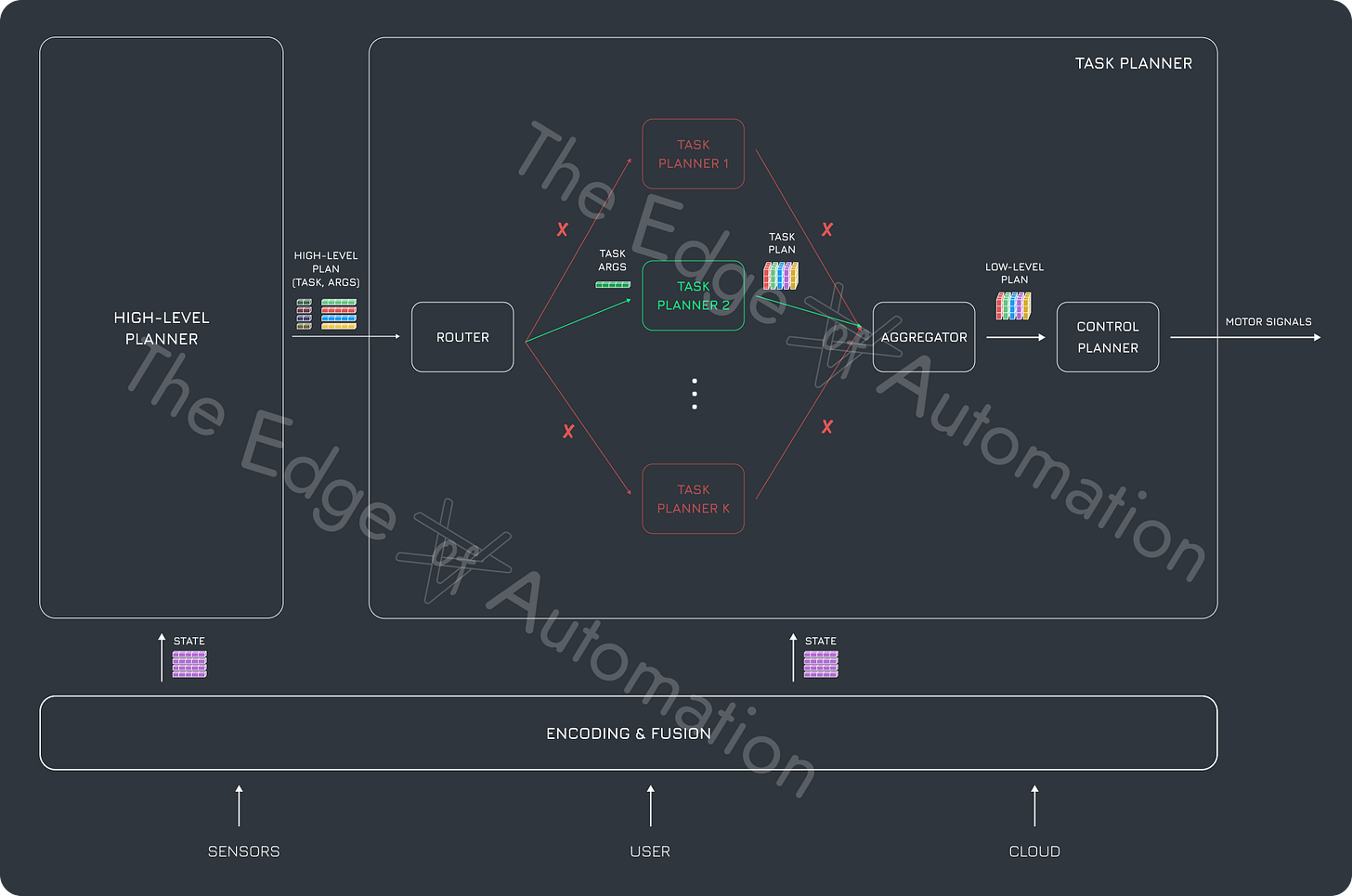
Quintet AI provides a suite of over 100 task-specific AI models that can be either orchestrated by a powerful central planner or integrated into clients' existing systems on a plug-and-play basis. These models have demonstrated success in executing complex, long-horizon tasks for humanoids, drone swarms, and factory mobile manipulators.
Most crucially, these networked models can undergo end-to-end optimization once their individual components prove reliable. This holistic training mitigates sharp corners and develops fluid transitions between related actions like walking and running, or different gripping strategies for varied objects and surfaces. Rather than remaining trapped in isolated competencies, robots build task networks that adapt and improve through continued operation. The system learns to anticipate and smoothly handle edge cases where multiple skills must blend together, creating truly robust automation capable of operating in unstructured environments.
This combination of imitation learning and modular skill networks accelerates robot deployment while improving reliability. Rather than struggling to handle every edge case through explicit programming, modern systems learn directly from human expertise while building a growing library of reusable capabilities. The result is robots that can tackle real-world tasks with unprecedented adaptability, marking another crucial step toward the birth of a true robotics industry.
2.4. Simulation, Data, and World Models
The leap from prototype to commercial deployment demands exhaustive testing across countless scenarios – a process that would be prohibitively expensive and dangerous with physical robots alone. Advanced simulation has emerged as the critical accelerant, enabling AI models to explore, fail, and improve through billions of virtual trials. This capability to rapidly iterate in synthetic environments, combined with increasingly sophisticated world models, is finally unlocking the path to robust, deployable robotics at scale.
2.4.1. Video Generation
Recent breakthroughs in video synthesis have revolutionized simulation. Where early systems struggled with basic physical consistency, new architectures can maintain coherent object interactions across extended sequences. OpenAI's Sora marked a turning point, demonstrating AI's growing grasp of spatiotemporal dynamics. Industry leaders quickly followed: Google's Veo2 introduced interactive scene manipulation, while Meta's VideoJam perfected stable motion through advanced optical flow modeling.
These advances directly enable more realistic robotics AI training. Generated sequences now capture subtle physics – how materials deform, how surfaces interact, how objects respond to forces. This fidelity is crucial for developing robots that can handle real-world complexity. Video generation has evolved from a technical curiosity into an essential tool for bootstrapping physical AI.
2.4.2. Simulating Missing Sensor Data
Physical robots integrate multiple sensor streams – vision, touch, force, proprioception – that are challenging to simulate comprehensively. New "sensor hallucination" techniques bridge this gap by inferring missing modalities from available data. NVIDIA's sensor fusion framework calibrates sensors across multiple modalities. At Quintet AI, we employ large self-supervised models to approximate complete sensor suites from partial observations, such as generating realistic touch feedback from visual inputs alone.
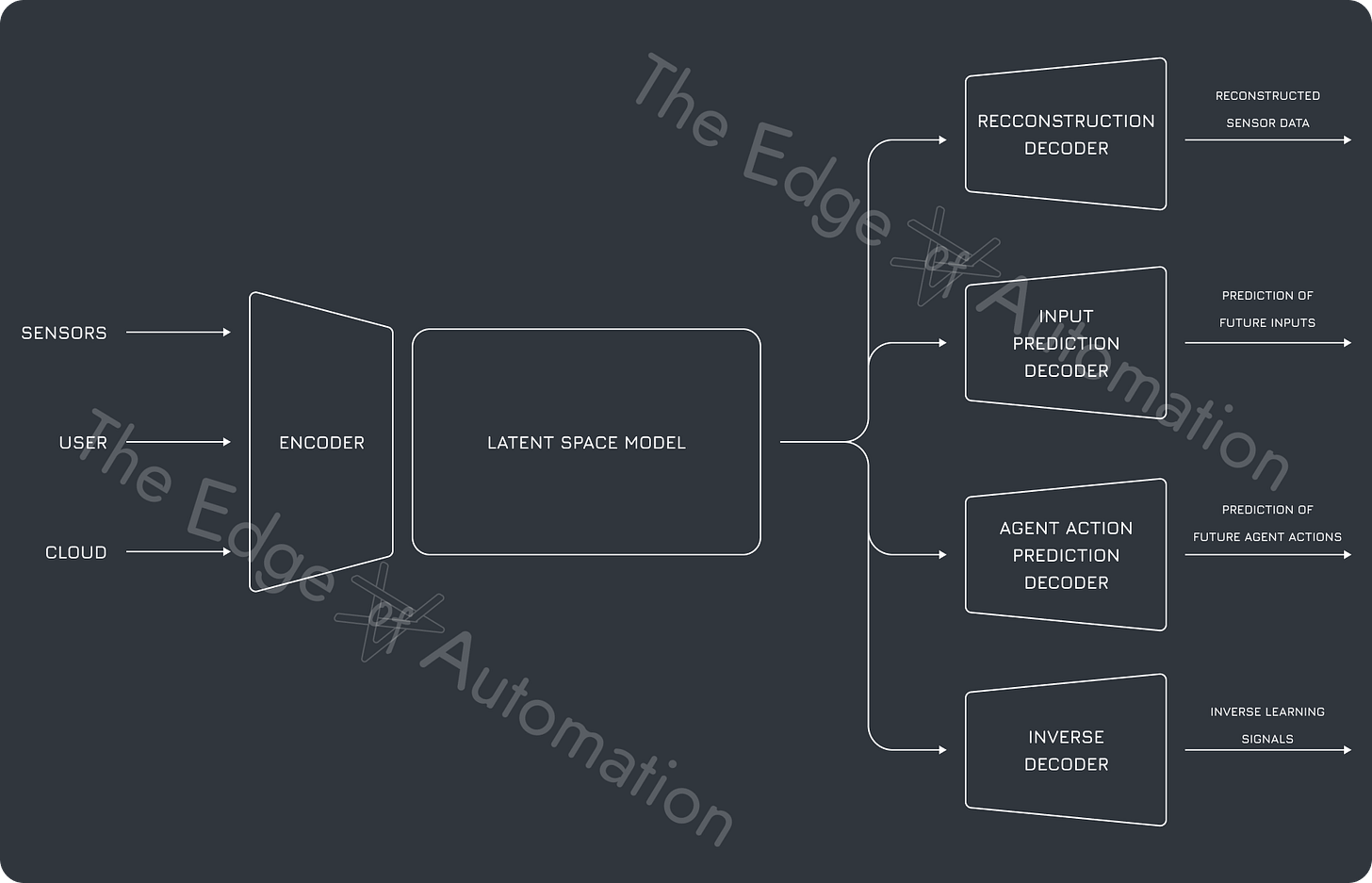
This breakthrough enables two critical capabilities that accelerate commercial deployment: robust fallback systems when physical sensors degrade or fail, and an abundance of synthetic training data. Rather than spending months gathering real-world examples, teams can now generate diverse, accurate sensor logs that capture the full spectrum of operating conditions. This shift from physical to synthetic data collection has compressed development cycles from years to months, marking another crucial step toward viable industrial robotics.
2.4.3. The Rise of Simulators
A new generation of simulators has emerged, combining photorealistic rendering, physics-based modeling, and generative AI to create virtual training environments for robotics. Recent breakthroughs like PhysDreamer demonstrate how diffusion models can infer material properties directly from video, enabling accurate simulation of complex physical interactions. PhyScene pushes this further by generating interactive 3D environments from text descriptions while maintaining physical constraints. Sim Anything integrates physics simulation with advanced rendering to capture realistic object interactions over time.
Video: Physically Interactable 3D Scene Synthesis for Embodied AI (PhyScene)
Major industry players are investing heavily in comprehensive simulation platforms, recognizing their crucial role in scaling robotics deployment. Carnegie Mellon's Genesis project offers an end-to-end environment specifically optimized for robotics development, while NVIDIA's Cosmos platform aims to integrate with their broader Omniverse ecosystem. These platforms enable safe, rapid iteration that would be impossible with physical prototypes alone. Teams can stress-test robots against rare edge cases, validate safety protocols, and refine control policies without risking hardware damage.
The implications extend far beyond simple testing. By generating vast quantities of synthetic training data, these simulators accelerate the development of robust Physical AI models that can generalize across diverse environments. Though simulator technology remains in its early stages, it is steadily advancing toward enabling adaptable intelligence through millions of hours of virtual interactions. This capability to rapidly iterate in synthetic environments while maintaining physical accuracy is another essential milestone in bridging the gap between prototype and mass deployment.
2.4.4. World Models and Robotics
World models represent a fundamental shift in robotics development, transcending basic physics engines to create comprehensive simulations of physical reality. These sophisticated platforms combine advanced material properties, realistic physics, and probabilistic scenario generation to replicate the complexities robots encounter in real environments. Early frameworks like SCENIC demonstrated the potential by introducing probabilistic variations in autonomous driving scenarios. Today's world models go further, enabling simulation of everything from material deformations to complex multi-object interactions across vast ranges of environmental conditions.
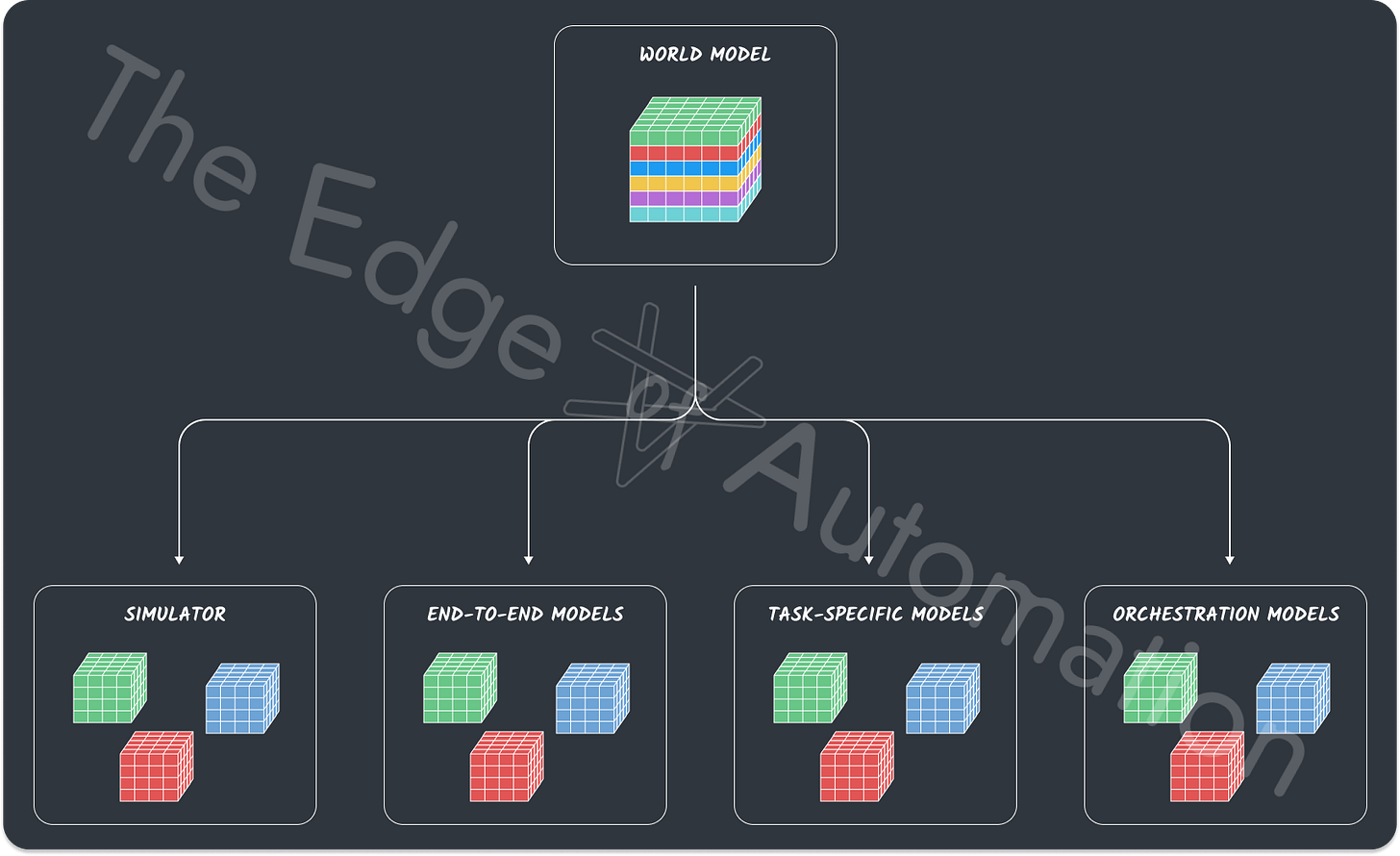
This breakthrough transforms how we develop Physical AI by enabling extensive pretraining on synthetic data that would be impossible to gather in the real world. Rather than waiting months to collect limited real-world examples, teams can generate billions of variations spanning countless edge cases and failure modes. A robot learning to manipulate delicate electronics can experience thousands of different component configurations, lighting conditions, and failure scenarios without risking actual hardware. This comprehensive synthetic training creates robust models that arrive in the real world with built-in adaptability to novel situations.
At Quintet AI, we use robotics world models to generate synthetic data that represent physical environments. These models provide robust, generalizable simulations of the real world, offering an unprecedented toolset for AI development.
The increasing fidelity of world models is pushing robotics toward a simulation-first development paradigm. Models pretrained on vast synthetic datasets now require only minimal real-world fine-tuning, while their exposure to diverse virtual scenarios yields better generalization than systems trained purely on physical data. This shift is particularly powerful for reinforcement learning, where agents can safely explore risky strategies and accumulate lifetimes of experience in accelerated virtual environments. The economics of robotics development are fundamentally changing – what once required years of careful physical testing can now be largely accomplished through sophisticated simulation.
2.5. Reinforcement Learning
Reinforcement learning (RL) transforms robots from pre-programmed machines into systems that evolve through experience. When combined with advanced simulation, RL enables robots to accumulate thousands of hours of virtual practice before touching physical hardware. This capability – training machines through trial and error at massive scale – separates modern Physical AI from traditional approaches that relied on rule-based planning.
2.5.1. RL Agents in Robotics
The core innovation of RL lies in its ability to discover optimal strategies through guided exploration. Rather than explicitly programming each movement, engineers define reward structures that encourage desired outcomes. A humanoid learns to walk by maximizing stability and forward progress; a manipulator masters precise assembly by optimizing successful completions. The agent continuously refines its approach through countless attempts, developing increasingly sophisticated behaviors that would be impossible to code by hand.
Video: RL Agent for UniTree G1 Humanoid
Modern simulators accelerate this process by running billions of parallel training scenarios. Robots can safely experiment with risky maneuvers, learn from catastrophic failures, and validate new capabilities without risking hardware damage. Once policies demonstrate competence in simulation and (often) undergo fine-tuning with real-world data, they transfer to physical robots through carefully designed adaptation layers that bridge the reality gap. This simulation-to-reality pipeline has slashed development cycles from years to months
2.5.2. Hierarchical and Multi-Agent Planning
Complex tasks demand more than single monolithic policies. Hierarchical RL (HRL) decomposes challenges into manageable layers: high-level planners that coordinate strategy, mid-level controllers handling motion sequences, and low-level systems managing individual joints. This separation mirrors human cognition, where abstract goals naturally cascade into concrete motor actions.
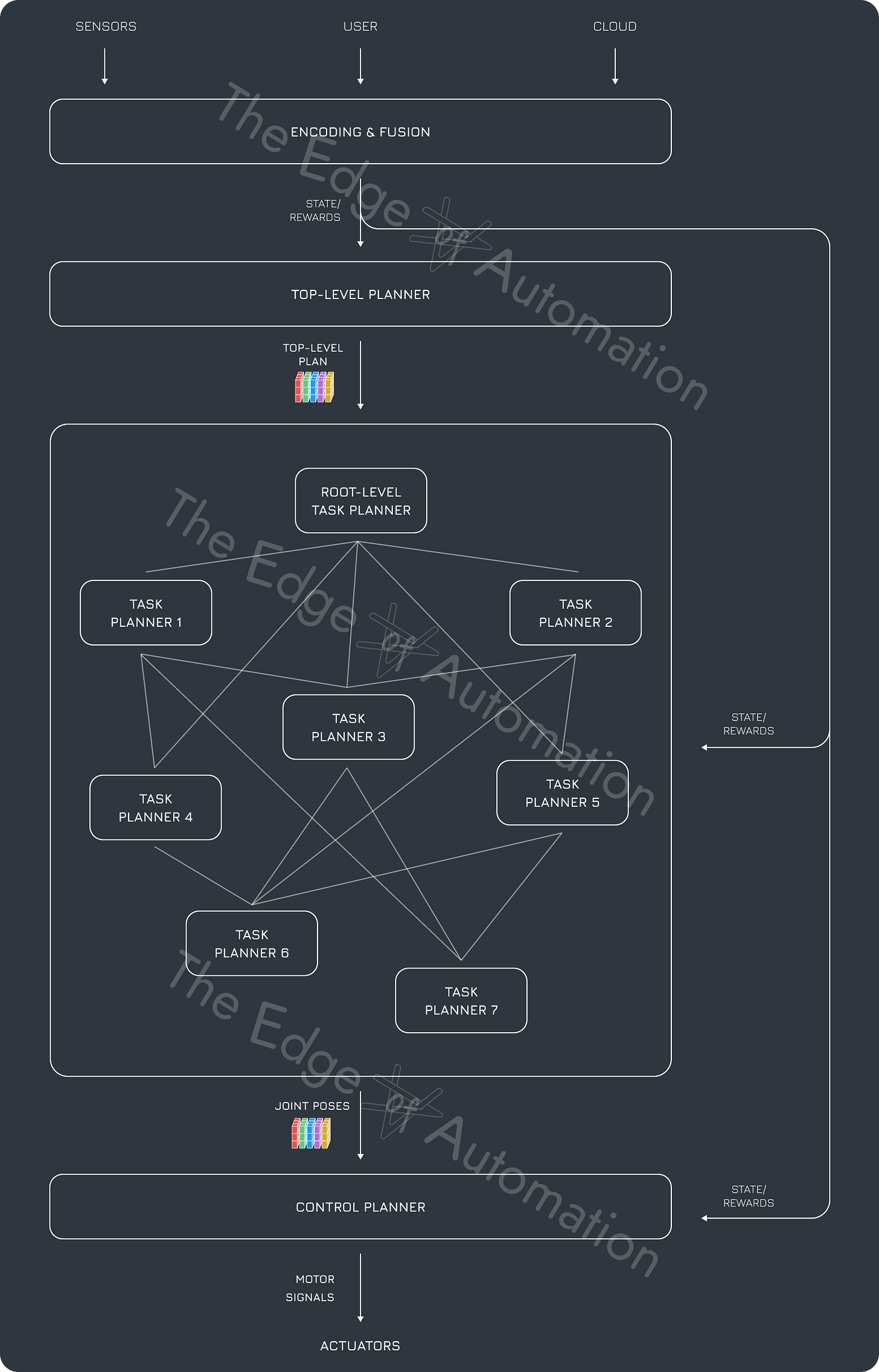
In warehouse environments, this hierarchical approach enables sophisticated automation. A high-level planner might optimize overall item distribution and traffic flow, while mid-level controllers coordinate specific robot movements, and low-level systems handle precise gripper control and obstacle avoidance. Each layer operates semi-independently yet remains coordinated through shared reward structures, creating fluid and adaptable behavior.
Multi-Agent RL (MARL) amplifies these capabilities further. Swarms of mobile robots learn to distribute tasks dynamically, adapting their collective behavior to changing conditions. Assembly lines coordinate multiple robots that synchronize their movements to handle shared workpieces, much like a team of humans collaborating on complex tasks. These frameworks create emergent behaviors – patterns of cooperation and adaptation that would be impossible to program explicitly – far surpassing what any centralized control system could orchestrate.
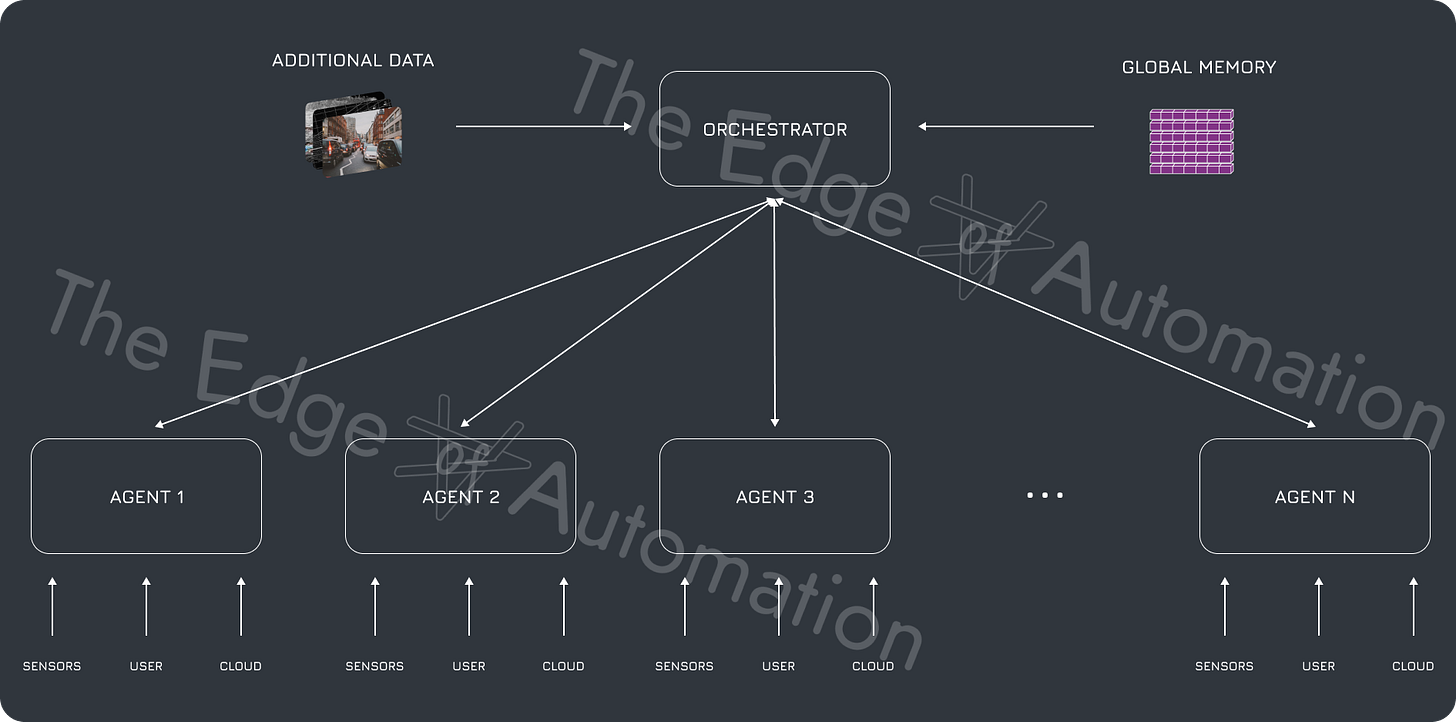
The true power of hierarchical and multi-agent RL emerges in real-world deployment, where robots must handle unpredictable scenarios and shifting objectives. A fleet of warehouse robots can autonomously redistribute tasks when one unit requires maintenance, and assembly systems can smoothly adjust their coordination patterns when product specifications change. This adaptability, achieved through layered learning and collective intelligence, is essential for the creation of robots that can truly replace human labor.
2.5.3. Adaptability to Unseen Scenarios
Reinforcement learning offers unique advantages for developing robots that can handle novel situations gracefully. By combining massive synthetic datasets from simulators with novel architectures, RL creates a powerful feedback loop where robots continuously generate their own training data through environmental interaction. This approach proves especially potent when dealing with real-world complexity, as the machine's direct experience helps it discover robust strategies that traditional supervised learning lacks.
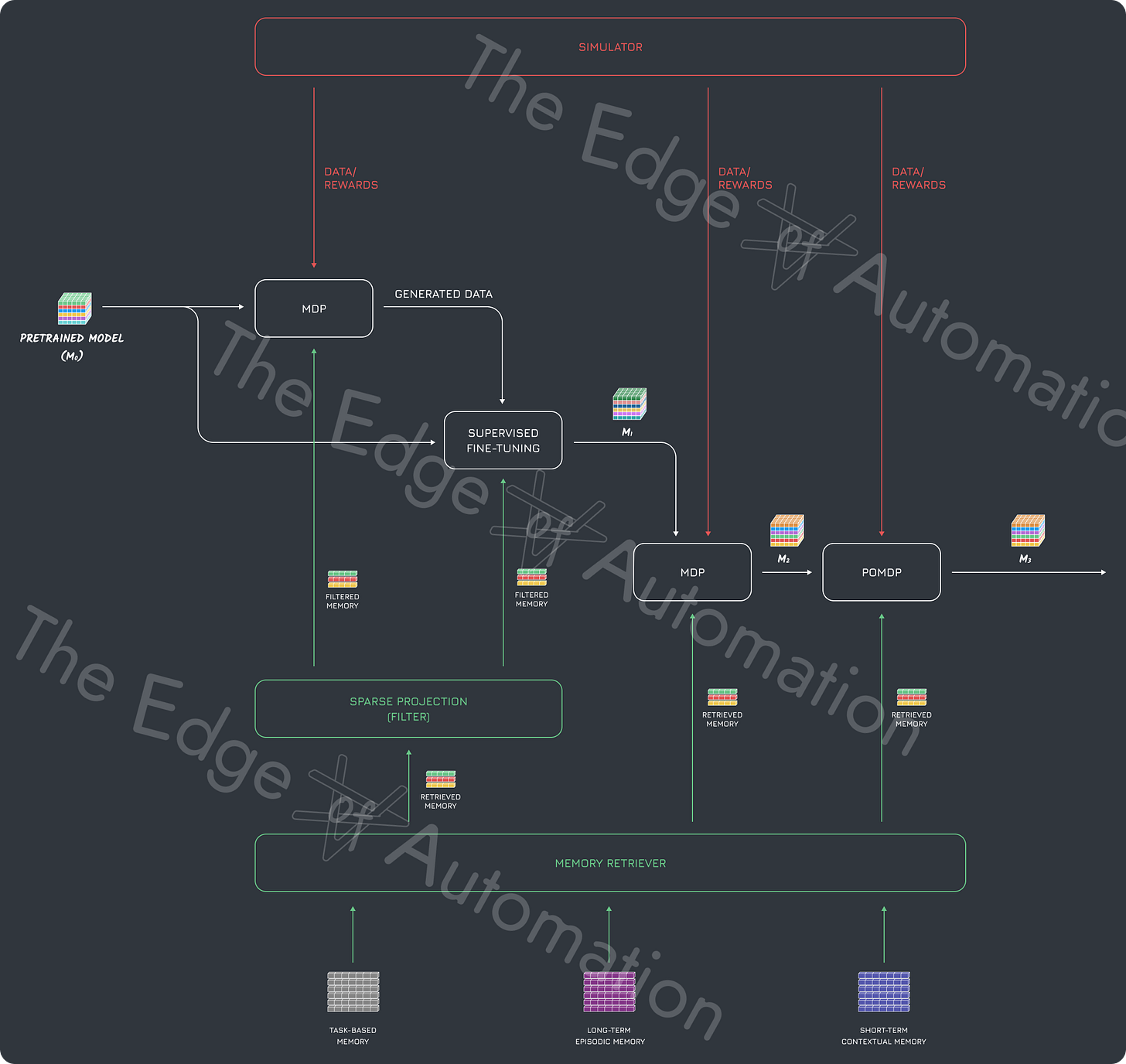
Multi-agent training provides another crucial pathway to generalization. When multiple RL agents interact, they naturally create diverse scenarios that challenge each other's assumptions. This dynamic can prevent models from overfitting to specific patterns, as strategies that work against one agent may fail against another. More sophisticated approaches like Partially Observable Markov Decision Processes (POMDPs) explicitly encourage policies that perform well with incomplete information – a crucial capability for robots that frequently encounter unfamiliar environments with imperfect sensor data.
The combination of RL techniques with advanced world models creates a particularly powerful synergy. As simulators generate increasingly realistic training environments, RL agents can accumulate vast experience across diverse scenarios. The policies they discover often exhibit surprising adaptability, finding clever ways to handle edge cases that designers never explicitly considered. This capability to extrapolate beyond training distributions, combined with the continuous generation of high-quality synthetic data, establishes a virtuous cycle that accelerates the development of truly adaptable robotics systems.
2.5.4. RL Simulators
Reinforcement learning now plays a role not only in training robots but also in creating the environments used to train them. "Adversarial" simulation agents continuously generate challenging scenarios, probing the edges of a robot's capabilities. These agents learn to identify and exploit weaknesses in robot policies, creating increasingly complex test cases that drive rapid improvement. For instance, a simulator might gradually introduce more difficult object arrangements in a pick-and-place task, or generate increasingly unstable terrain patterns for locomotion training.
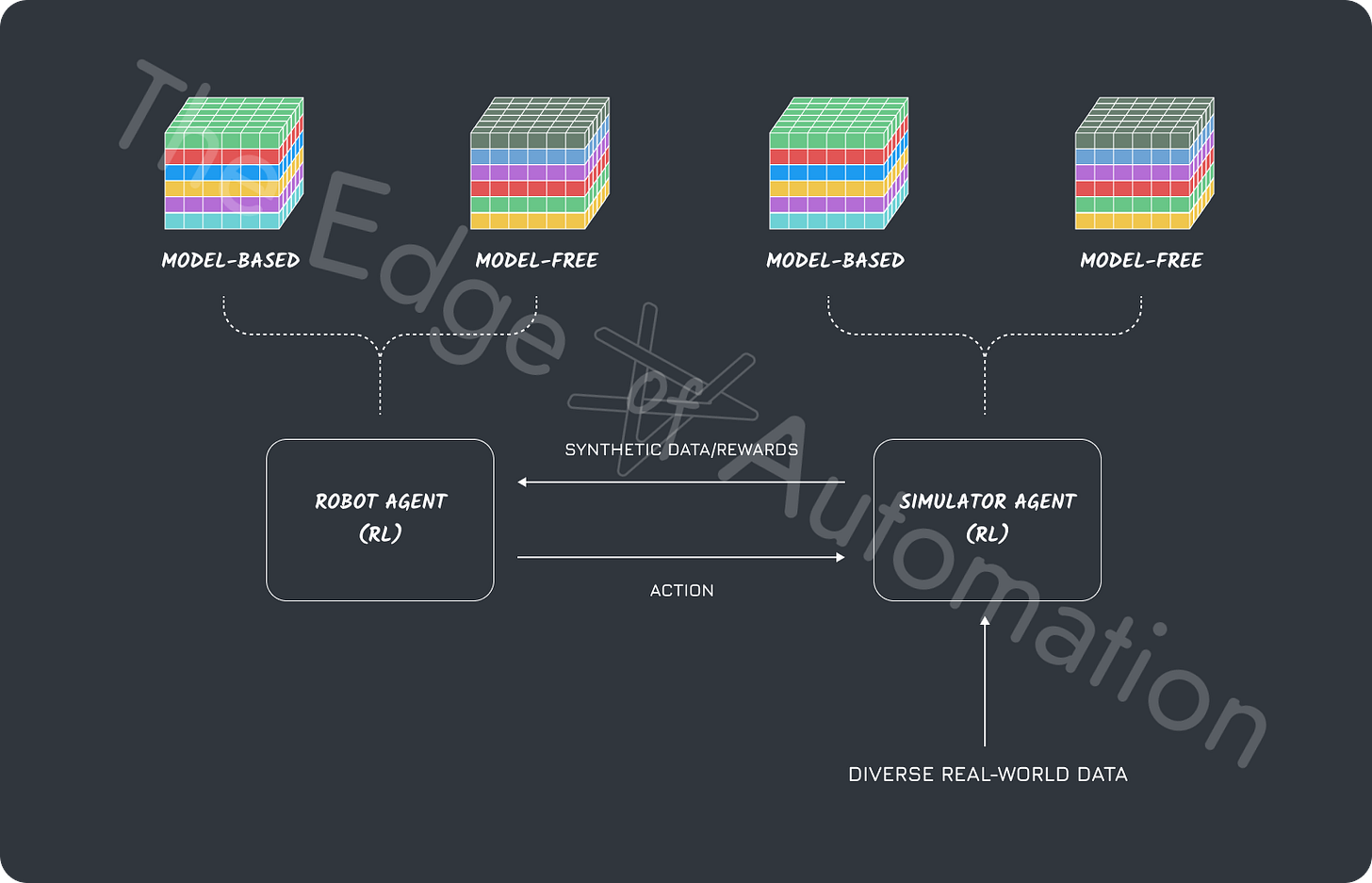
This approach transcends traditional simulation methods that relied on hand-crafted scenarios and rigid physics models. Modern RL-driven simulators can automatically adjust physical parameters – friction coefficients, material properties, lighting conditions – to create robust training regimes. At Quintet AI, we pioneer self-improving simulation loops that systematically expand scenario complexity while maintaining physical realism. The system learns which parameters most effectively challenge current robot capabilities, creating a form of automated curriculum that accelerates development.
The implications extend beyond individual training sessions. As simulators become more sophisticated, they begin capturing subtle real-world phenomena that were previously impossible to model. A simulator might learn to replicate the way different materials deform under pressure, or how surface textures affect grip stability. This growing fidelity, driven by the continuous feedback loop between robot performance and simulator refinement, is rapidly closing the reality gap that historically limited sim-to-real transfer in robotics.
2.5.5. Path to Self-Evolving Physical AI
As simulators, RL agents, and physical robots become more tightly integrated, a new paradigm emerges: self-evolving Physical AI. Robots share experiences across a fleet, feeding real-world data back into training simulations and collectively improving their capabilities. This continuous learning loop means deployed systems grow more capable over time, rather than remaining static like traditional automation.
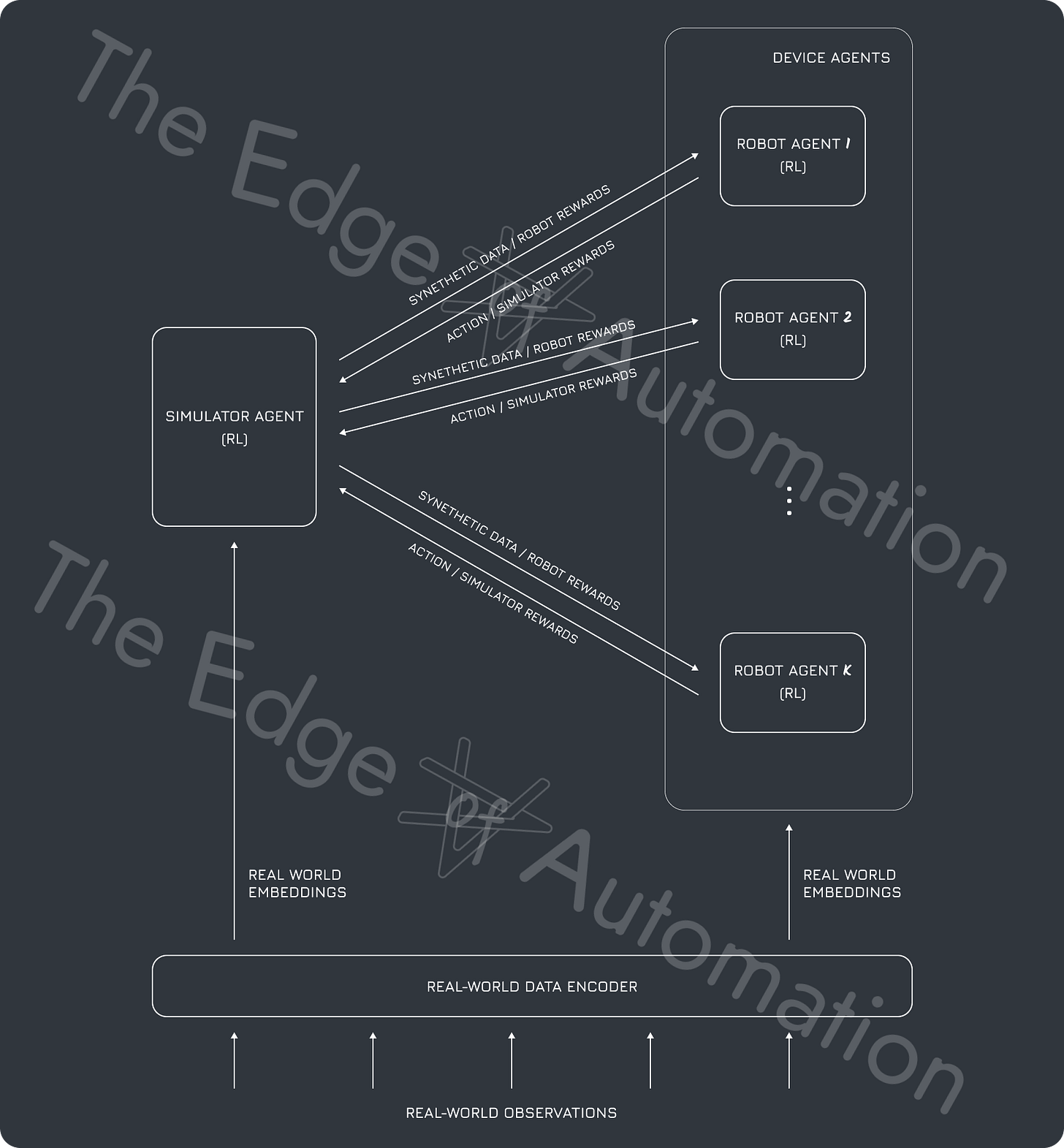
The implications are profound. Once an initial policy proves viable, it becomes a foundation for perpetual refinement as conditions change – whether those changes involve new products, altered facility layouts, or shifting business demands. This ability to adapt and improve through experience, more than any single technical capability, highlights RL's essential contribution to the birth of true robotics.
2.6. Meta-Learning
Meta-learning represents a crucial leap beyond traditional reinforcement learning, enabling robots to adapt to novel situations with minimal data. Rather than requiring extensive retraining for each new task, meta-learned systems develop a form of "learning to learn" – acquiring fundamental skills that transfer rapidly to unfamiliar challenges. This capability proves essential for deploying robots in dynamic environments where tasks and conditions constantly evolve.
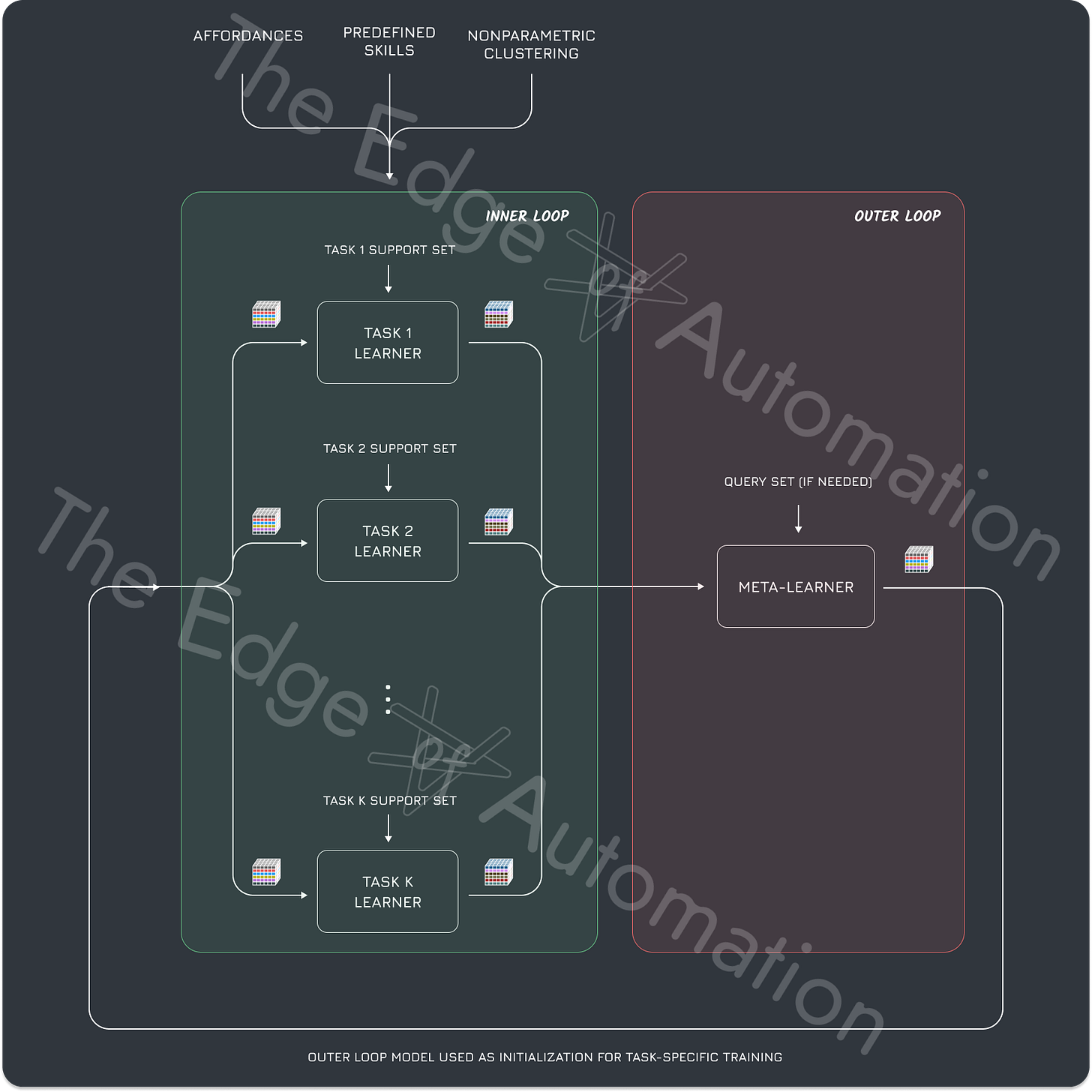
The technical architecture typically involves nested optimization loops: an inner loop that learns task-optimized parameters, and an outer loop that discovers generalizable parameters from them. Advanced techniques like Almost No Inner Loop (ANIL), Moreau Envelope Meta-Reinforcement Learning (MEMRL), and Reptile have made this process computationally tractable even for highly complex models. By avoiding expensive second-derivative calculations while maintaining strong transfer performance, these methods bring meta-learning from theoretical concept to practical deployment.
For the emerging robotics industry, meta-learning bridges a critical gap between specialized and general-purpose automation. A single meta-trained robot can rapidly adapt to diverse applications – from precision electronics assembly to retail merchandising – after seeing just a handful of demonstrations. This flexibility transforms the economics of automation, enabling robots to handle the constant task variations that once made human labor irreplaceable. As meta-learning continues to mature, we're approaching systems that can seamlessly pivot between domains without the burdensome reprogramming that plagued earlier automation attempts.
2.7. Cloud-Edge Coordination
Advanced demands a careful balance between local processing and cloud intelligence. Edge computing handles critical real-time decisions – a robot must instantly react to falling objects or unstable loads without waiting for remote input. Modern AI accelerators enable sophisticated neural networks to run directly on-board, giving robots the low-latency intelligence needed for dynamic environments. Figure's humanoids demonstrate this capability by processing complex manipulation tasks entirely on local hardware, maintaining fluid motion even with intermittent connectivity.
Video: Figure AI Helix (On-Board VLM)
Yet the cloud remains indispensable for higher-order coordination and learning. When multiple robots operate in shared spaces – whether a busy warehouse or assembly line – centralized systems can optimize global behavior by analyzing aggregate data and dispatching refined strategies. The cloud also enables fleet-wide learning, where insights from individual robots improve the capabilities of the entire network. Tesla's approach with Optimus exemplifies this synergy: robots operate autonomously using local models while continuously sharing experience data that enhances collective performance.
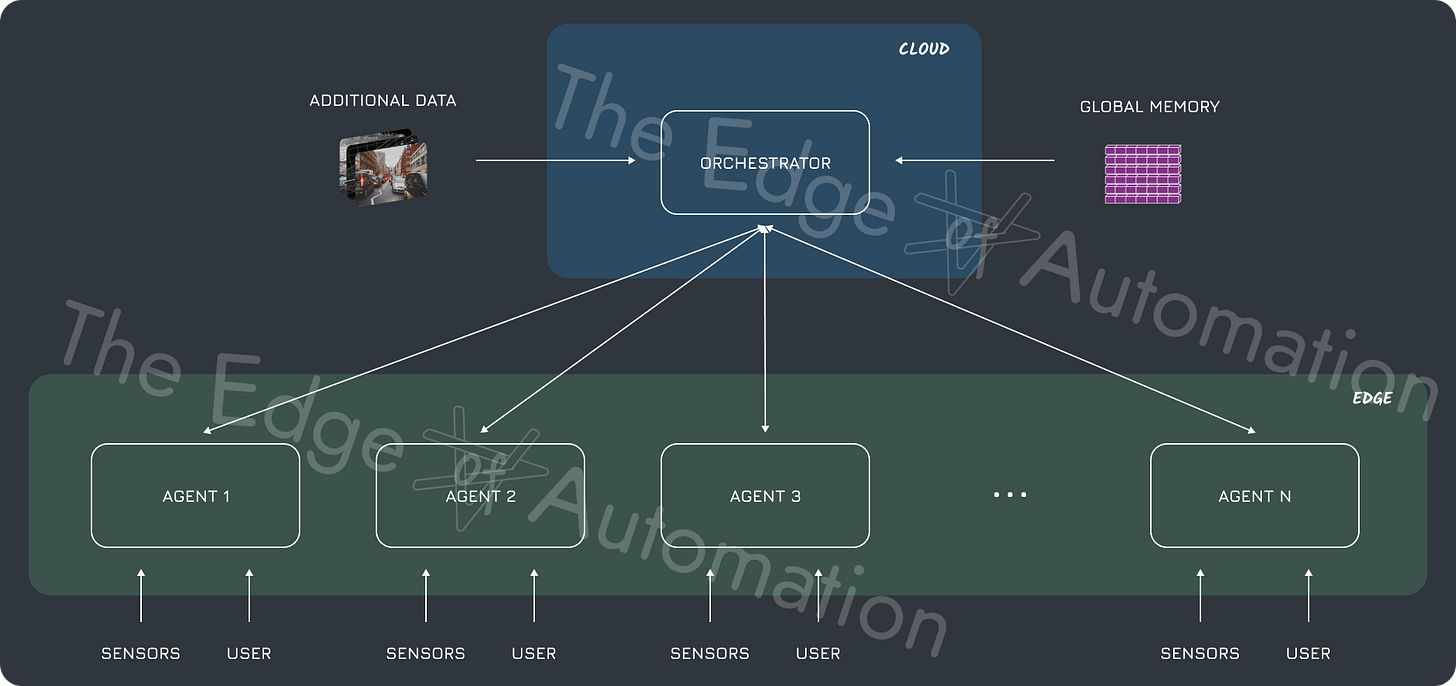
Recent advances in model compression and specialized hardware have transformed this coordination. Edge devices can now run sophisticated local policies without draining batteries, while cloud infrastructure handles massively parallel optimization with minimal latency. More importantly, robots no longer fail when connectivity drops – they continue operating independently using cached models and local intelligence, then sync and update once connections resume. This resilience is instrumental in enabling practical deployment at scale.
The true power lies in fusion rather than compromise. Cloud-edge coordination creates systems that combine the immediate responsiveness of local processing with the strategic intelligence of networked computation. As these architectures mature, they enable robot fleets that are both individually capable and collectively adaptive – a cornerstone capability for the emerging age of widespread automation.
From multimodal AI to cloud-edge orchestration, we're witnessing a departure from traditional automation. The powerful combination of foundation models, reasoning architectures, and learning frameworks has finally unlocked the fluid, generalizable intelligence that robotics has long pursued, arriving precisely as market forces create unprecedented demand for automated labor.
3. Industry Readiness
For decades, robotics remained trapped in a fragmented landscape of research projects, never coalescing into a true industry. Today, this deadlock is breaking as elite talent floods in from AI labs and tech giants, while desperate capital pours in from powers that see automation as existential. This unprecedented alignment of talent, technology, and strategic imperatives leads to the birth of the first real robotics industry that will finally lift civilization’s age-long dependency on human physical labor.
3.1. New Blood, New Talent
Tesla's 2021 Optimus announcement marked more than another corporate robotics initiative – it catalyzed a fundamental shift in how the industry approaches automation. Unlike previous projects trapped in perpetual R&D cycles, Tesla's vision centered on immediate commercial deployment within their own factories. This product-first mindset triggered a cascade of changes across the robotics landscape, drawing world-class talent from AI labs and consumer electronics giants into new ventures focused on viable deployment rather than mere demonstration.
Video: Elon Musk announces Tesla Optimus (2021)
This influx of entrepreneurial leadership brings crucial capabilities that the field previously lacked. Companies like Figure and Apptronik combine sophisticated engineering with practical business acumen, breaking from the academic mindset that kept robotics perpetually in prototype phase. As seasoned executives and engineers migrate from established tech giants, they bring proven methodologies for scaling complex technologies. Their expertise in AI, hardware, supply chain management creates a multiplier effect, transforming isolated research projects into commercially viable platforms.
3.2. Early Signs of Hardware Standardization
Robotics is finally breaking free from its fragmented past as manufacturers converge on standardized architectures. Leading humanoid makers have aligned on crucial design choices: high-torque electric motors with harmonic drive gearboxes for joints, EtherCAT protocols for real-time communication, and integrated sensor suites combining cameras with depth sensors. This agreement represents growing consensus on optimal solutions for general-purpose robotics. As suppliers scale production of these core components, they're establishing the supply chains needed for mass manufacturing.
Formal standardization efforts have emerged to accelerate this transformation. In 2024, China's Ministry of Industry and Information Technology formed a dedicated technical committee for humanoid standardization, engaging manufacturers and researchers to define specifications for hardware interfaces, safety requirements, and use-case standards across industrial and consumer applications. This initiative, backed by China's unmatched manufacturing infrastructure, promises to create reference designs that new projects can adopt rather than starting from scratch – similar to how standardized motherboards accelerated the personal computer revolution.
Video: BMW and Figure Partnership
Cross-industry partnerships further reinforce these standards. Major automotive manufacturers have begun collaborating with robot makers, bringing decades of experience in safety protocols and quality control to robot design. These relationships expose robotics companies to established industrial practices, from standardized maintenance procedures to rigorous testing requirements. More importantly, they create pressure for compatibility with existing factory systems, from standard tool interfaces to common communication protocols.
This standardization wave represents a progress toward the first true robotics industry. Just as the personal computer industry exploded once hardware components became standardized and interoperable, robotics is approaching a similar inflection point. The emergence of common architectures and components will dramatically lower development barriers while improving reliability through proven designs. As these standards mature and supply chains scale, they'll accelerate the transition from isolated prototypes to mass-produced platforms capable of transforming global manufacturing.
3.3. Shadows of a New Software Ecosystem
The robotics software landscape is undergoing a systemic transformation, evolving from fragmented solutions toward cohesive AI-driven frameworks. This transformation is a departure from historical practices where teams crafted specialized code for every sensor and actuator, leaving robots brittle and trapped in endless integration cycles. The emergence of foundation models, pre-trained on vast datasets spanning real and synthetic experiences, is dissolving these constraints by providing adaptable cognitive backbones that can be efficiently tuned for diverse applications.
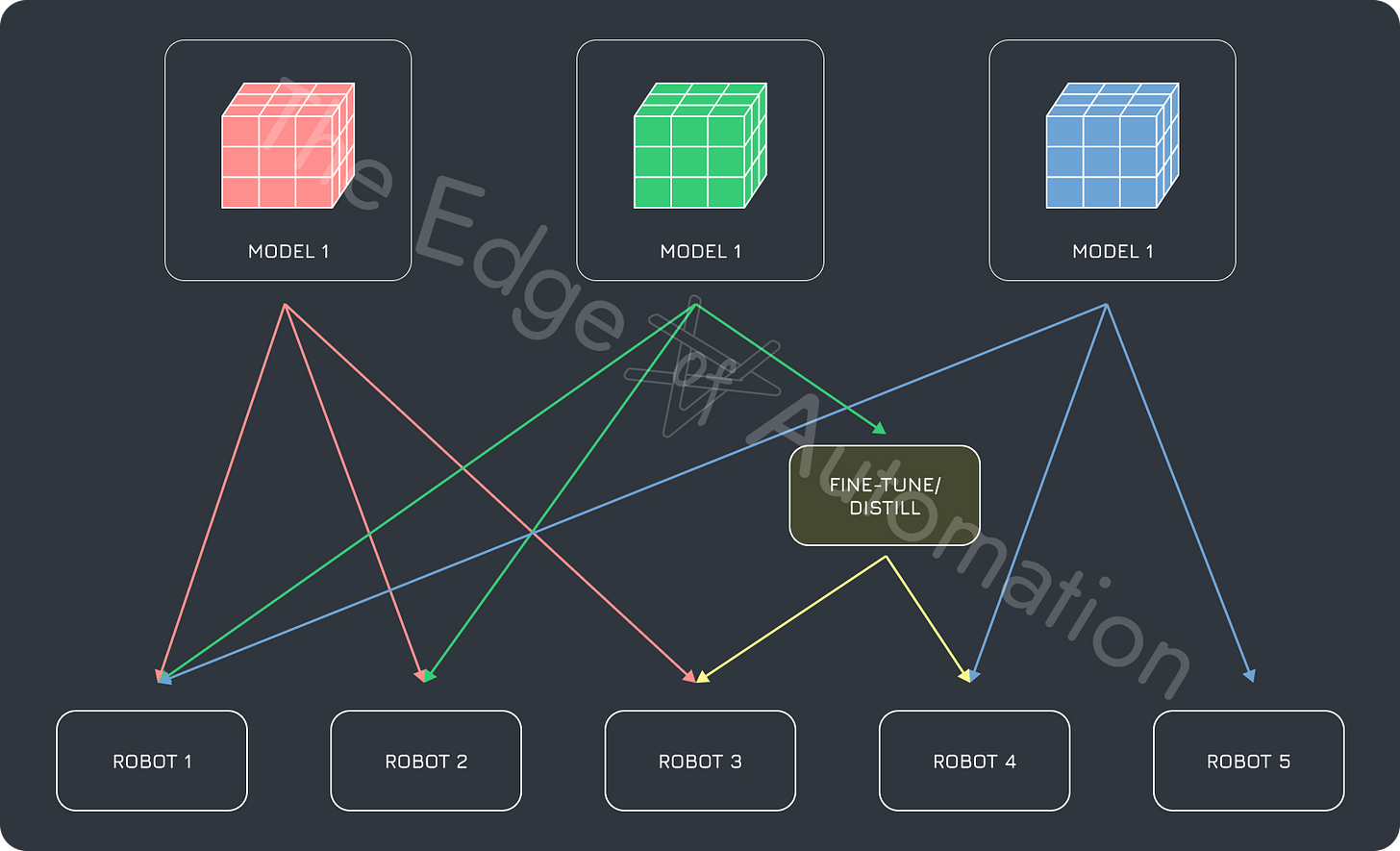
Modern robotics platforms leverage this architectural revolution to dramatically simplify system complexity. Rather than maintaining constellations of narrowly-focused perception and control modules, teams can deploy unified multimodal models that seamlessly fuse vision, tactile, and linguistic inputs. These models don't just process sensor data – they enable fluid reasoning about physical interactions, from interpreting scene geometry to planning complex manipulation sequences. The result is more resilient systems that can adapt to novel situations without requiring extensive reprogramming.
Video: Simulating Robots and Soft Interactions with the World
The emergence of sophisticated simulation environments has transformed development practices. Modern platforms combine physics-accurate modeling with procedural generation, enabling robots to accumulate thousands of hours of virtual experience before touching physical hardware. This capability to rapidly iterate in synthetic environments, combined with robust reinforcement learning pipelines, has compressed development cycles from years to months while improving system reliability.
While still emerging, the foundations of a true software ecosystem are becoming visible through cloud-based infrastructure for model training, deployment, and fleet management. As these platforms mature and standards emerge, they're creating pathways for robots from different manufacturers to share capabilities through compatible APIs and model architectures. This shift toward shared platforms, more than any single technical breakthrough, signals robotics' evolution from isolated achievements into a coordinated industry capable of scaling to meet civilization's mounting automation imperatives.
3.4. Desperation-Fueled Capital Flow
The current surge of investment in robotics is different from previous hype cycles. Unlike the speculative frenzies of the past, we now witness the methodical deployment of capital by powerful entities that see automation as an existential imperative. The prospect of robots substituting human labor transcends mere technological advancement – it threatens to shatter one of civilization's oldest constraints: the scarcity of physical labor. For those who have amassed generational wealth through existing power structures, this looming transformation compels swift and decisive action.
The capital sources reflect this strategic urgency. Old-money dynasties and established institutions – particularly from Asia, Middle East, and Europe – are mobilizing unprecedented resources. These aren't entrepreneurs seeking the next unicorn but custodians of generational wealth who recognize robotics as a force that could fundamentally reshape their power base. Their deep connections to banking systems and governmental bodies enable them to marshal investment flows far greater than previous automation initiatives. The public announcements of robotics breakthroughs by influential figures serve as carefully calculated signals to power structures worldwide: embrace this transformation or face extinction.
This "desperation-fueled" capital creates a powerful accelerant for the emerging robotics industry. Unlike the boom-bust cycles of autonomous vehicles or consumer robotics, this investment wave shows remarkable patience. The stakes transcend quarterly returns – these backers understand that whoever masters Physical AI will control the future of global manufacturing. This alignment of deep pockets with long-term strategic imperatives provides the sustained funding needed to bridge the gap between prototype and mass deployment, bringing humanity's first true robotics industry closer to reality.
Tesla's 2021 Optimus announcement may have catalyzed the shift, but it took the convergence of entrepreneurial talent, emerging standards, AI-driven platforms, and strategic capital to transform robotics into a commercial possibility. Today's landscape marches inexorably toward shared platforms and standardized practices, while war chests of desperate investment ensure these machines will find real-world deployment.
Yet this alignment of industry forces represents more than technological maturation – it reflects broader geopolitical imperatives reshaping global power structures. As we'll explore next, the race for general-purpose mobile manipulation has become a crucial battleground in the struggle for technological dominance.
4. Geopolitical Alignment
The robotics revolution transcends technological innovation – it's fundamentally driven by the dissolution of post-Cold War unipolarity. As regional powers consolidate their spheres of influence, automated manufacturing has evolved from an efficiency tool into a cornerstone of modern sovereignty. Nations are pursuing robotics not just for economic advantage, but also for survival in a new reality where technological capability determines power.
4.1. The Return of Empires
The global order is fracturing into distinct power spheres, each racing to secure automated manufacturing capabilities as a foundation of modern sovereignty. This isn't merely a shift in international relations – it's a fundamental restructuring of how nations project power and maintain industrial capacity. The brief unipolar moment of American dominance is giving way to a multipolar landscape where technological self-sufficiency, particularly in Physical AI and robotics, determines a nation's position in the emerging hierarchy.
Two primary hubs have emerged as the decisive players in this realignment: the United States and China. Each commands vast markets, sophisticated tech ecosystems, and deep capital reserves needed to develop and deploy advanced robotics at scale. Their competition has transformed robotics from a technological pursuit into a strategic imperative, as each power bloc seeks to secure its manufacturing base through automation.
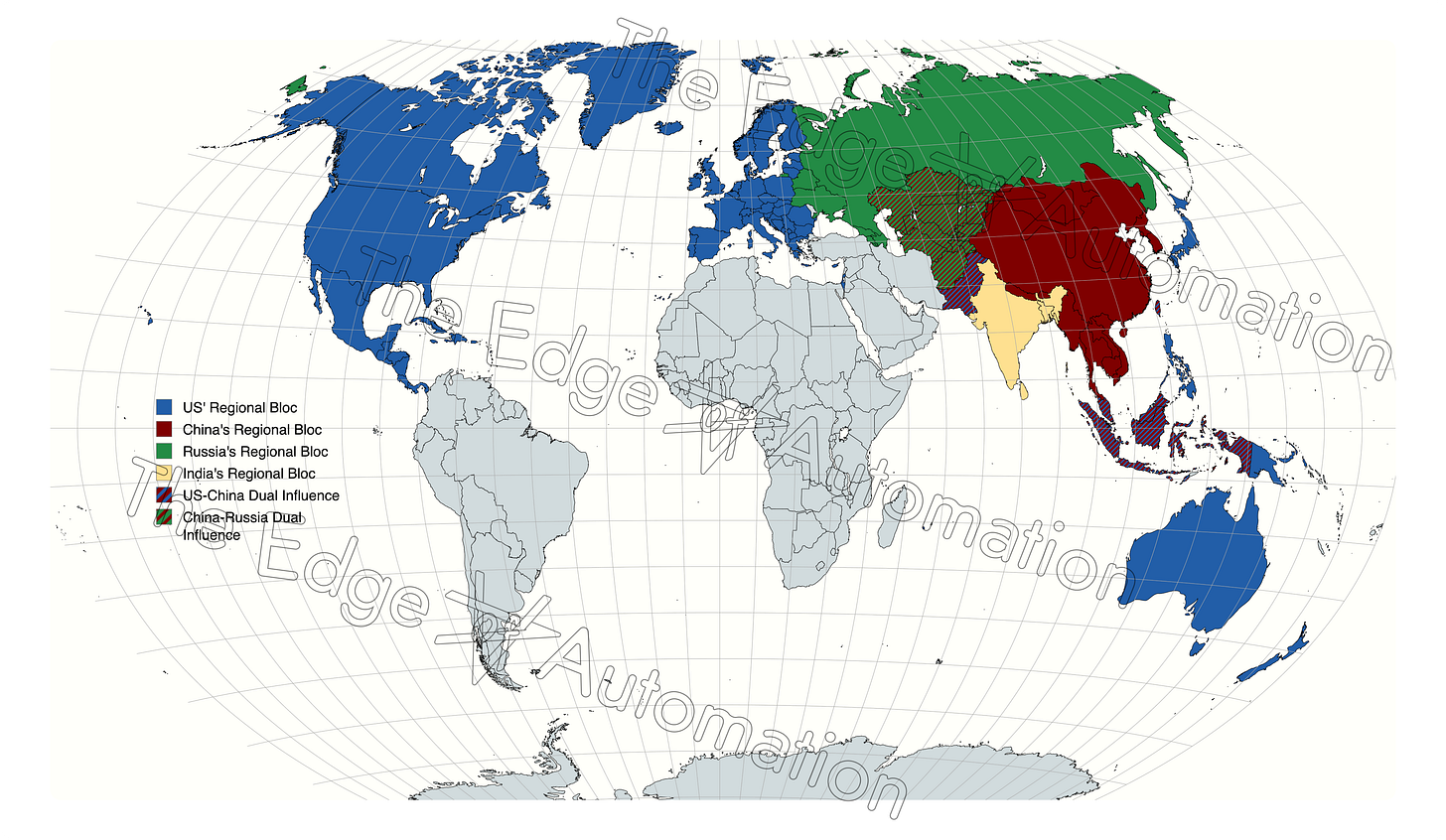
This new multipolar reality creates unprecedented momentum for robotics development. Unlike previous waves of automation driven by efficiency or cost savings, today's investments flow from existential necessity. Nations that fail to establish robust automated manufacturing capabilities risk becoming dependent vassals in a world where physical labor – historically the foundation of industrial power – can be replicated through machines.
The implications extend far beyond individual robots or factories. As regional powers consolidate their spheres of influence, they're racing to build not just individual machines but entire automated industrial ecosystems. This competition has unleashed massive capital flows and policy support that were unthinkable during the unipolar era. Robotics has evolved from an optional innovation into a cornerstone of modern statecraft – a transformation that ensures sustained investment and deployment at a scale previously impossible.
4.2. Decoupling Supply Chains
Global supply chains are undergoing a fundamental restructuring that makes robotics not just desirable but imperative. The era of seamless globalization – where manufacturing naturally flowed to lowest-cost regions – is giving way to a new paradigm defined by strategic autonomy and technological sovereignty. This shift creates unprecedented demand for automation that can sustain domestic manufacturing in developed economies while enabling China to overcome its demographic constraints.
For Western economies, the reshoring imperative exposes a harsh reality: rebuilding domestic manufacturing requires solving the fundamental labor equation. The era of offshoring to low-wage regions is ending, yet developed nations lack the workforce skilled enoough to sustain large-scale production. This forces a decisive pivot toward automation – not as an optional efficiency gain, but as the only viable path to industrial sovereignty. The resulting wave of investment in humanoid robotics isn't driven by incremental cost savings, but by the existential need to maintain manufacturing capability in a fragmenting global order.
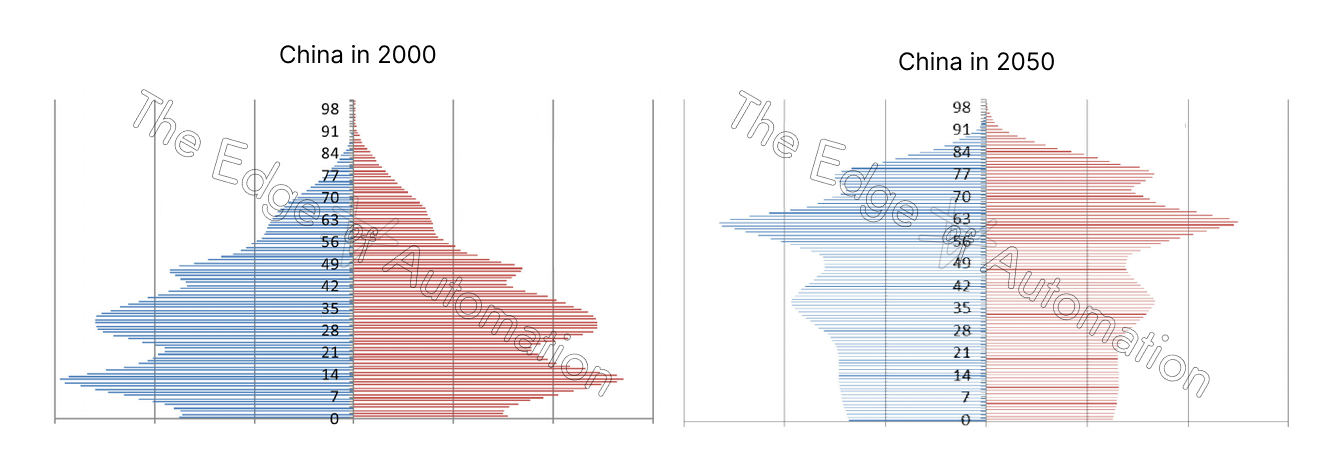
China faces its own existential pressure toward automation. The demographic cliff from decades of population control threatens its manufacturing dominance precisely as global supply chains fragment. Beijing's response has been decisive: unprecedented investment in robotics and automation to maintain industrial capability even as its workforce shrinks. This isn't merely modernization – it's a strategic imperative to prevent critical industries from developing external dependencies.
These fundamental forces – Western reshoring and China's demographic crisis – create unstoppable momentum for robotics deployment. Traditional industrial automation proves insufficient; the complexity of human tasks demands machines with unprecedented adaptability. This pressure drives investment in true robotics: platforms that can handle the fluid, unstructured work that has historically required human labor. Unlike previous waves of automation focused on narrow efficiency gains, this transformation stems from nations and power structures fighting for survival in a fragmenting global order.
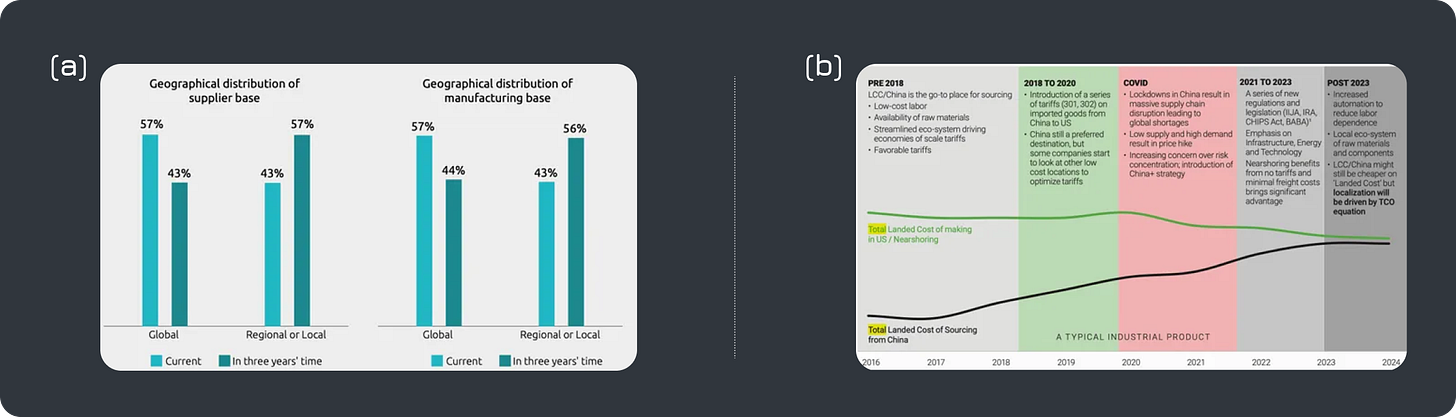
As these dynamics intensify, they're forging the first true robotics industry. The desperate need for automated manufacturing capability ensures sustained capital flow and rapid deployment at scale. More fundamentally, it guarantees that robotics will evolve beyond isolated research projects into standardized, mass-produced platforms capable of reshaping global production networks. The age of automation isn't just coming – it's being dragged into existence by the systemic forces transforming our world order.
4.3. Race for Technological Supremacy
The robotics race has transcended traditional commercial competition to become a proxy battleground in the struggle for global technological supremacy. Nations now view mastery of Physical AI and automation not as mere economic advantages, but as cornerstones of modern power projection. This shift transforms robotics from an academic pursuit into a strategic imperative that shapes military doctrine, industrial policy, and international relations.
China's aggressive investment in robotics exemplifies this new reality. The latest Five-Year Plan explicitly prioritizes humanoid development and automated manufacturing, backed by massive state investment and coordinated industrial policy. This isn't just about economic efficiency – it's a calculated bid to secure technological independence while the demographic dividend fades. Heavy investments by Xiaomi and UniTree represent more than corporate success; they're instruments of national strategy in the race for automation supremacy.
Video: China Unveils First Humanoid Robot Training Base in Shanghai
The United States has responded by mobilizing its own technological arsenal. DARPA's continued investment in advanced robotics projects signals the Pentagon's recognition that tomorrow's conflicts will hinge on autonomous capabilities. The National Security Commission on Artificial Intelligence has explicitly warned that falling behind in robotics threatens America's strategic position. This elevation of robotics to national security priority has unleashed unprecedented resources, from direct funding of research to export controls targeting critical components.
This competition creates powerful accelerants for commercial robotics. When Tesla demonstrates new manipulation capabilities or UniTree showcases advanced mobility, these aren't mere technical achievements – they're signals that reshape national priorities and corporate strategies worldwide. The need to maintain technological parity drives rapid cross-pollination of innovations, as countries rush to match or exceed their rivals' capabilities.
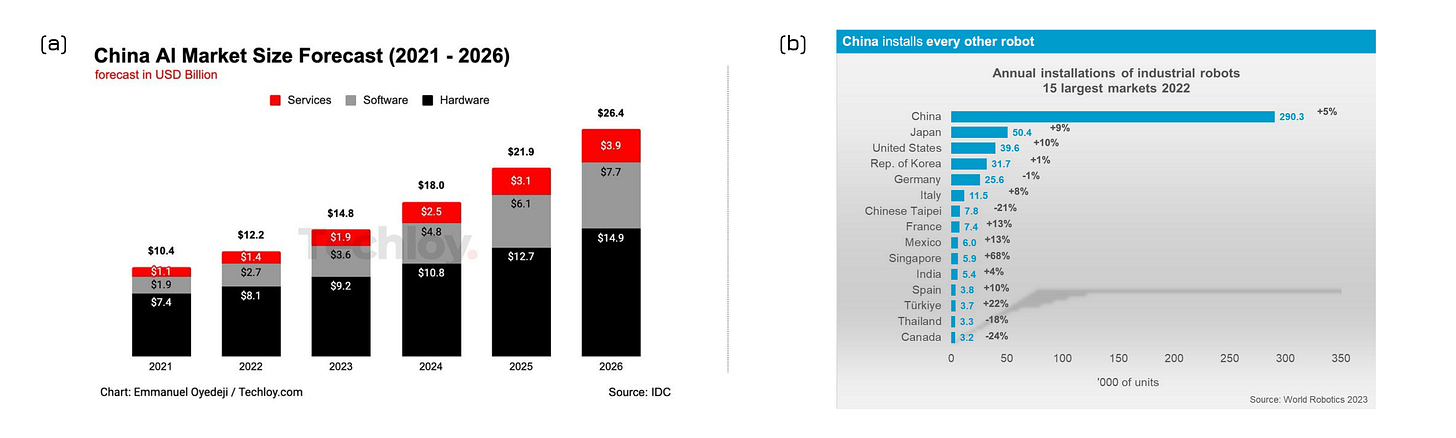
Military applications particularly accelerate this dynamic. Defense establishments worldwide increasingly view autonomous systems as force multipliers that could decide future conflicts. This drives investment in crucial areas like bipedal locomotion and swarm coordination – capabilities that directly benefit commercial robotics development. The Russia-Ukraine War has only intensified this focus, demonstrating how even basic autonomous systems can dramatically impact modern warfare.
Video: DARPA’s Drone Swarm Demonstration
Most significantly, this strategic competition has fundamentally altered investment horizons. Unlike commercial robotics initiatives that must show quick returns, national programs can sustain long-term development toward crucial capabilities. This patience, combined with substantial capital, enables breakthrough advances that would be impossible under normal market constraints. The result is an unprecedented acceleration of robotics technology, driven not by market forces but by the existential imperative of maintaining technological supremacy.
This elevation of robotics to strategic imperative ensures sustained momentum toward mass deployment. No major power can afford to lag in automation capability, as falling behind threatens both economic competitiveness and national security. The result is a self-reinforcing cycle where each breakthrough intensifies pressure for further advancement, compressing development timelines that once stretched decades into mere years.
These geopolitical imperatives transform robotics into strategic necessity. As nations race to secure automated manufacturing capabilities in a decoupling global order, they create unstoppable momentum toward widespread adoption. This isn't merely about building better robots but also about establishing the industrial foundation that will define power in coming decades. When survival rather than profit drives automation, the pace of adoption becomes relentless and irreversible.
V. Anatomy of the Emerging Industry
The robotics revolution isn't just another technological wave – it represents the dismantling of humanity's oldest constraint: the scarcity of physical labor. As artificial intelligence fuses with advanced hardware, we're witnessing the birth of an industry that will fundamentally reshape civilization. This transformation brings together foundational robots, AI models, cloud services, and desperate capital in ways that transcend traditional automation.
This section maps the products, players, and power dynamics defining this nascent industry. From vertically-integrated humanoid makers to specialized component suppliers, from established manufacturers to ambitious startups, these forces are converging to create the first true robotics ecosystem – one that promises to rewrite the rules of human work forever.
1. Products on Horizon
Soon, robots will no longer confined to research labs and promotional videos. A new wave of commercial products is emerging – from adaptable humanoid platforms to the sophisticated cloud systems that manage them. Unlike the specialized industrial arms or autonomous vehicles of past decades, these offerings aim squarely at replacing human labor across diverse environments. What makes this moment unique isn't just technological readiness, but the simultaneous maturation of viable hardware, practical AI architectures, and robust infrastructure that can support widespread deployment.
1.1. Foundational Robot Hardware
At the heart of this revolution are machines designed to operate in human environments without extensive modification. While traditional automation required carefully engineered spaces with safety fences and precise product positioning, the new generation of robots brings unprecedented adaptability. Humanoid platforms lead this charge – their anthropomorphic design isn't about aesthetics but compatibility. When everything from door handles to tool belts is built for human proportions, matching those dimensions enables seamless integration into existing workflows.
Video: Sanctuary AI Phoenix Gen 7
This shift extends beyond humanoids to a broader ecosystem of flexible platforms. Mobile manipulators combine wheeled or legged bases with dexterous arms, enabling fluid transitions between tasks like heavy lifting and precision assembly. Advanced drones evolve past simple surveillance or delivery to tackle complex maintenance and inspection duties. What unites these diverse forms is a crucial milestone: sufficient perception, dexterity, and onboard intelligence to handle real-world complexity with minimal oversight.
Video: Omron Mobile Manipulator
Component standardization accelerates this hardware revolution. Rather than each manufacturer building custom actuators and sensors, emerging supply chains offer tested, compatible modules that accelerate development while driving down costs. Early units may carry premium price tags and require frequent maintenance, but their presence in factories and warehouses creates a powerful feedback loop: successful deployments drive further adoption, which funds reliability improvements and cost reductions, broadening the market for the next generation of machines.
The era of research prototypes and isolated demos is ending. These foundational platforms – whether humanoid, mobile manipulator, or specialized drone – represent the first wave of commercially viable robots capable of truly replacing human labor. Their emergence signals a fundamental departure from decades of limited automation, setting the stage for a transformation that will reshape every industry touched by physical work.
1.2. Standardized Hardware Components
The robotics industry is finally breaking free from its fragmented past through emerging hardware standardization. Where previous generations required custom components for every joint and sensor, manufacturers are converging on common architectures that enable economies of scale. This shift mirrors the early personal computer revolution, where standardized motherboards and CPUs catalyzed an explosion of innovation.
Companies like Moog and Harmonic Drive specialize in high-performance actuators, while sensor manufacturers like Omron and Keyence produce plug-and-play modules for vision, touch, and force sensing. This implies a significant momentum toward creating a marketplace where robot builders can select from an expanding catalog of proven components rather than reinventing core subsystems for every machine they develop. This trend promotes efficiency and accelerates innovation in robotics development.
Video: Modular Actuators (HEBI Robotics)
China leads this standardization wave, leveraging its unmatched manufacturing infrastructure and deep engineering talent pool. Government-backed committees coordinate technical standards across the industry, from servo connectors to multi-axis force sensors. Chinese suppliers now offer entire "robot bodies" – assembled torsos, arms, and legs – allowing buyers to focus on AI and application-specific customization rather than fundamental hardware engineering.
This modularization extends to "generalized body parts" – integrated systems that combine motors, sensors, and quick-attach interfaces into plug-and-play limbs. As these modules grow more sophisticated, embedded controllers enable seamless integration with any robot's AI layer. The line between "robot builder" and "robot integrator" blurs, accelerating innovation by letting companies focus on software and specialized applications rather than basic hardware challenges.
1.3. Physical AI Models
Physical AI represents the "brain" that transforms mechanical platforms into genuine replacements for human labor. Unlike traditional automation that relies on rigid scripts, these models enable robots to perceive environments, reason about tasks, and execute complex actions with human-like adaptability. This leap from programmed routines to fluid intelligence marks a decisive break from decades of limited automation.
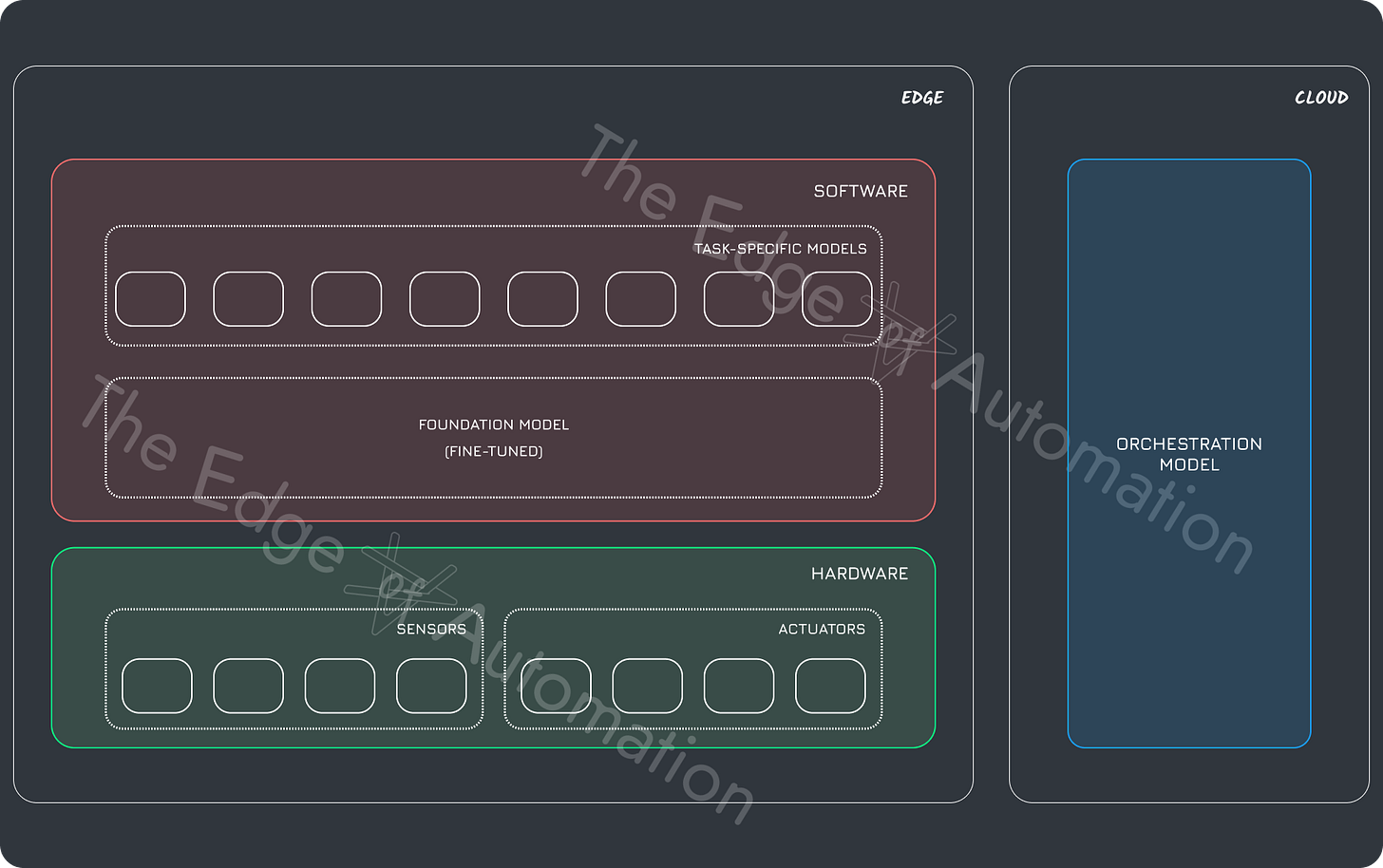
1.3.1. Foundation Models
Foundation models establish the bedrock of autonomy through massive pre-training on diverse physical interactions. These models unify vision, planning, and manipulation into cohesive architectures that can be efficiently fine-tuned for specific deployments. Similar to how large language models revolutionized natural language processing, robotics foundation models enable transfer learning and few-shot adaptation that were impossible with traditional approaches.
The implications extend beyond individual capabilities. A warehouse robot equipped with a robust foundation model can learn new pick-and-place tasks from minimal demonstrations, while maintaining core competencies in navigation and collision avoidance. This dramatic reduction in training overhead transforms the economics of deployment, enabling robots to handle novel scenarios without extensive reprogramming.
1.3.2. Task-Specific Models
While foundation models provide broad capabilities, task-specific models deliver the specialized intelligence needed for particular domains. These models augment foundation layers with deep expertise in areas like precision assembly, fabric manipulation, or tool handling. A semiconductor manufacturing model might incorporate advanced visual inspection and micron-level positioning, while a construction model could focus on structural analysis and material handling.
This layered architecture – foundation models enhanced by specialized skills – creates unprecedented flexibility. Robots can rapidly switch between tasks by loading different skill modules while maintaining core competencies. As these specialized models proliferate, they establish a growing library of capabilities that accelerates deployment across diverse industries.
1.3.3. Orchestration Models
Orchestration models complete the intelligence hierarchy by coordinating multiple robots and optimizing system-wide performance. Operating from edge or cloud infrastructure, these models translate high-level directives into coordinated actions across entire fleets. A factory orchestrator might optimize workflow by dynamically reassigning robots based on throughput bottlenecks, while a warehouse system could coordinate dozens of robots to maximize picking efficiency.
This capability to manage complex multi-robot operations foreshadows the transition toward Level 4 and 5 autonomy. As standardized platforms emerge and robot populations grow, orchestration models will evolve to handle increasingly sophisticated coordination – from factory-wide production optimization to city-scale automation of services and infrastructure.
These three model types – foundation, task-specific, and orchestration – establish the cognitive foundation needed for true robotics. By enabling machines to perceive, reason, and adapt with human-like flexibility, Physical AI bridges the final gap between mechanical capability and genuine labor replacement. This breakthrough in machine intelligence, combined with advances in hardware and unprecedented market demand, drives the industry inexorably toward mass deployment of truly capable robots.
1.4. Physical AI Services
The sophistication of modern robotics demands more than just models and hardware – it requires a comprehensive ecosystem of software services to bridge the gap between raw capabilities and real-world deployment. From training pipelines to operational monitoring, these "Physical AI Services" form the connective tissue that enables robots to move beyond controlled demonstrations into continuous, reliable operation.
1.4.1. Training and Fine-Tuning
While foundation models provide broad capabilities, most deployments require precise fine-tuning for specific tasks and environments. A humanoid sorting produce needs different sensitivities than one welding car frames. Training services fill this crucial gap by providing sophisticated pipelines that combine real-world data collection, synthetic simulation, and incremental reinforcement learning.
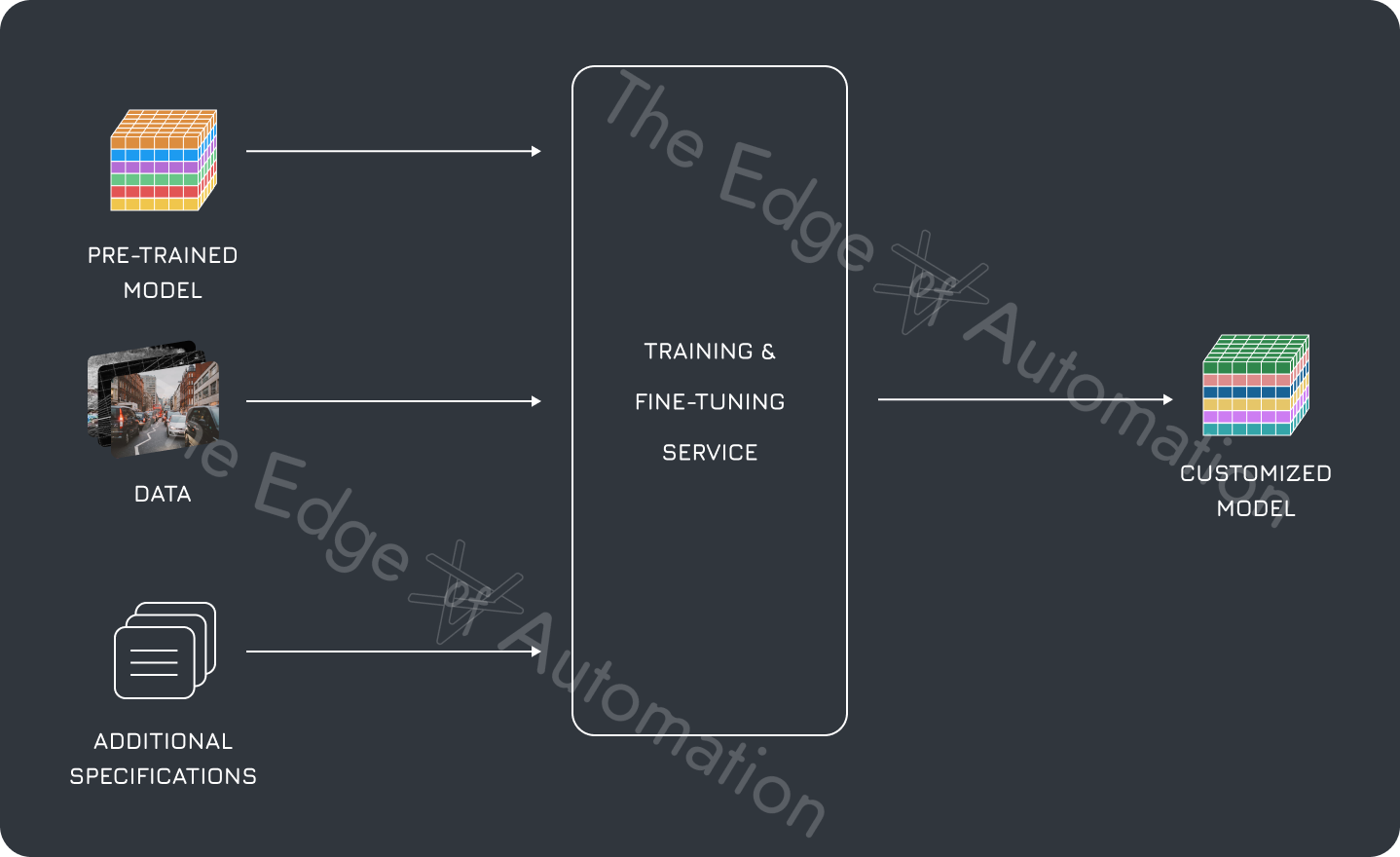
This dependence on specialized training mirrors the early autonomous vehicle industry, where every self-driving stack needed custom datasets for local road conditions. The key difference is scale – robots face nearly infinite variations in physical tasks, from delicate electronics assembly to heavy material handling. Early deployments will rely heavily on continuous cycles of deployment, data gathering, and refinement before foundation models become truly universal.
1.4.2. Model Management and Testing
The complexity of robotics AI – with multiple foundation models, task-specific skills, and orchestration engines – demands sophisticated management platforms. These services handle version control, compatibility validation, and performance benchmarking across dynamic conditions. Think of it as DevOps for Physical AI, where updates affect not just software but real-world physical interactions.
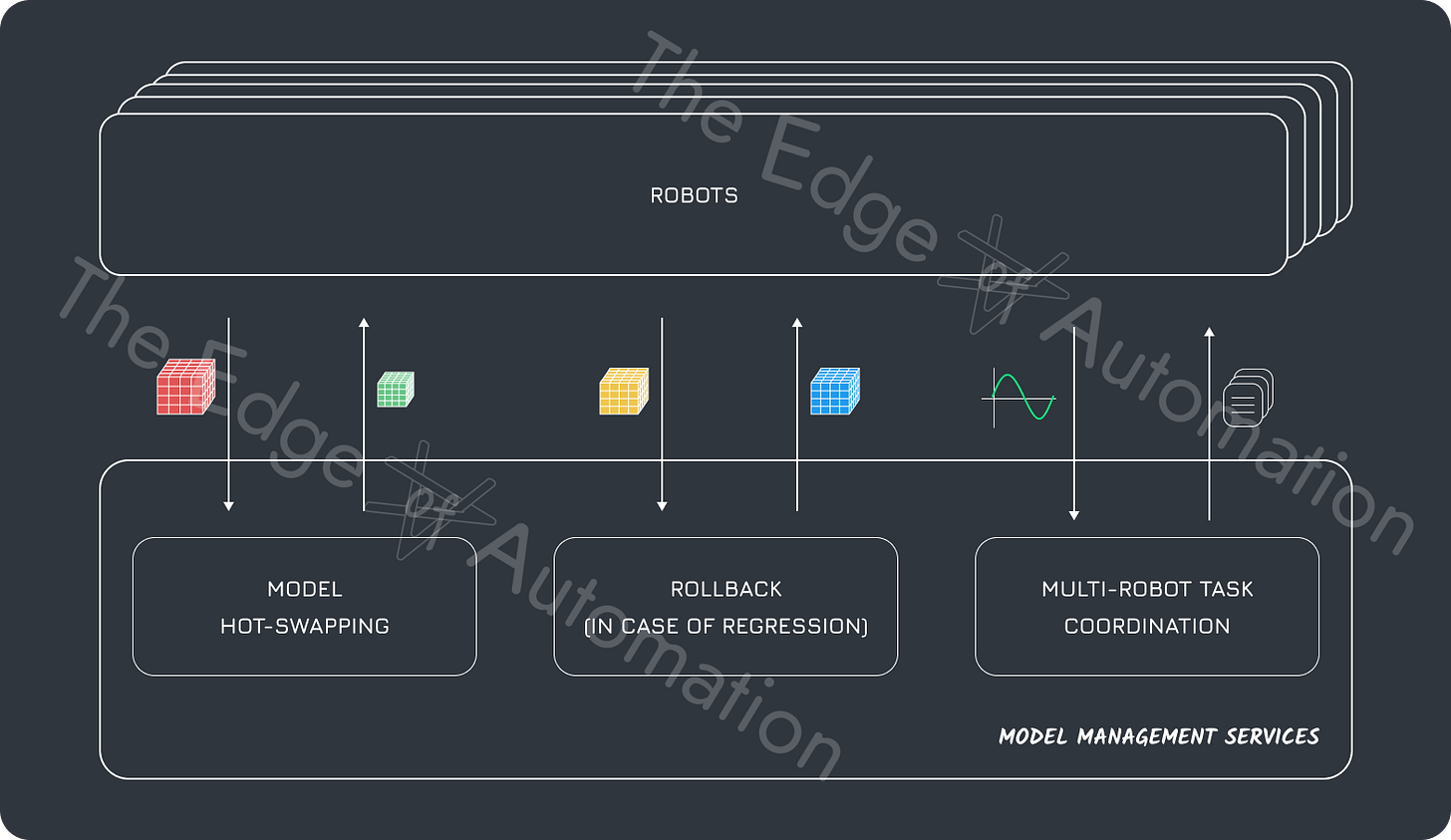
These platforms enable crucial capabilities like hot-swapping between heavy and light models based on computational constraints, or rapid rollback if a model update causes unexpected behavior. Rigorous testing protocols leverage both real-world pilots and high-fidelity simulations to validate changes before deployment, ensuring that robots maintain safety and reliability even as their capabilities evolve.
1.4.3. Observability and Operational Excellence
The transition from prototype to production demands comprehensive observability – the ability to monitor, analyze, and optimize robot performance in real-time. Modern platforms provide unified dashboards for tracking everything from sensor health to task completion rates, enabling rapid detection of emerging issues before they cause failures.
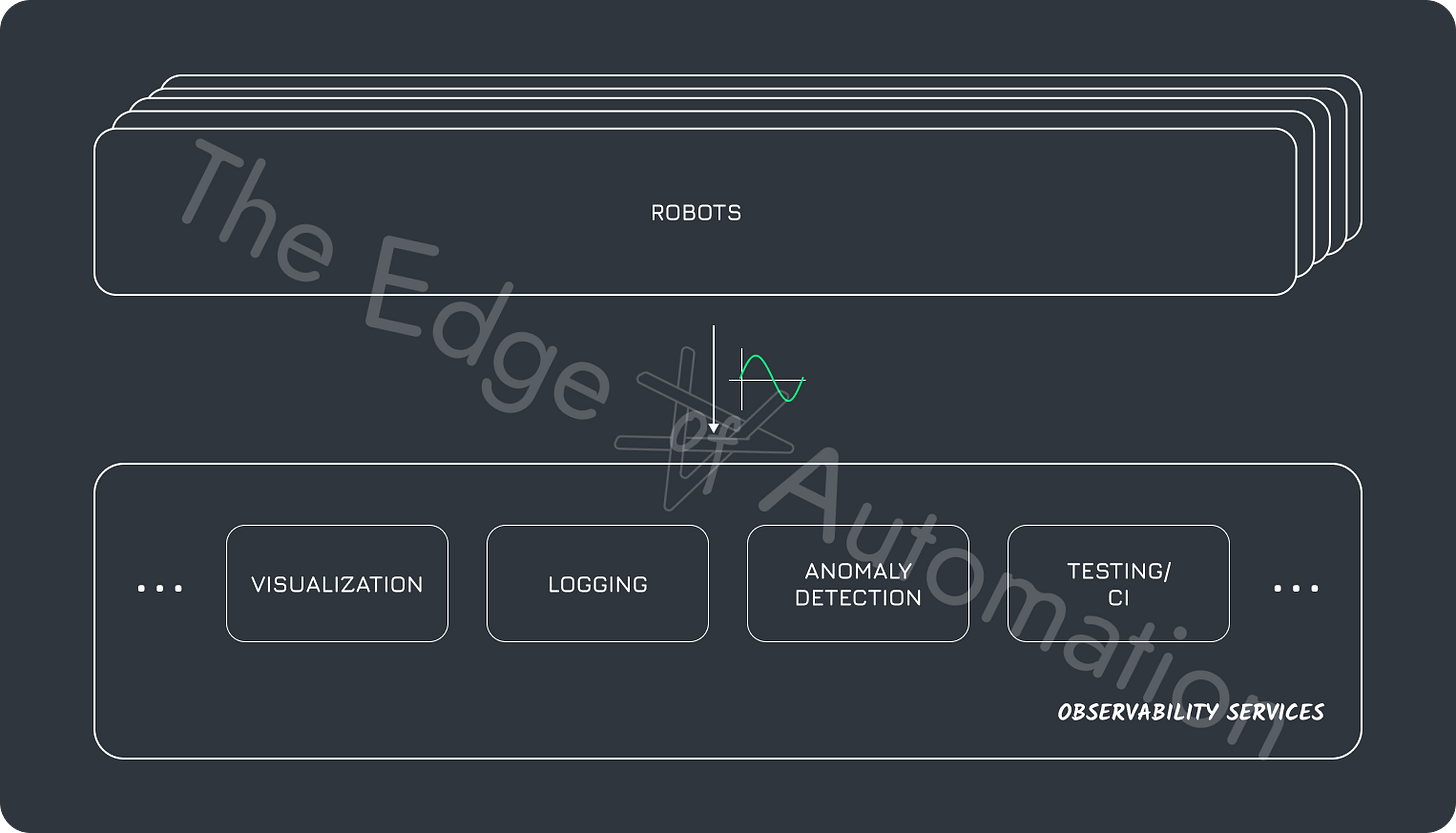
By aggregating data across entire fleets, these systems enable predictive maintenance and continuous improvement. Operators can identify patterns like degrading actuators or drifting model performance, then take corrective action before critical failures occur. This level of operational insight proves essential for building trust in autonomous systems, particularly in high-stakes manufacturing environments.
1.4.4. Simulation and Synthetic Data
The path to widespread robotics deployment runs through simulation. Physical robots must handle countless edge cases in messy, dynamic environments – a challenge that can't be solved through real-world data collection alone. Modern simulation platforms bridge this gap by generating vast quantities of synthetic training data, enabling robots to accumulate thousands of hours of virtual experience before touching physical hardware.
These aren't simple physics engines rendering basic scenes. Next-generation simulators integrate ray tracing, advanced material modeling, and real-time physics to capture the subtle complexities robots encounter: how fabric deforms under tension, how surface textures affect grip stability, how objects interact under varying lighting conditions. This fidelity proves crucial – even minor discrepancies between simulated and real-world behavior can cascade into catastrophic failures during deployment.
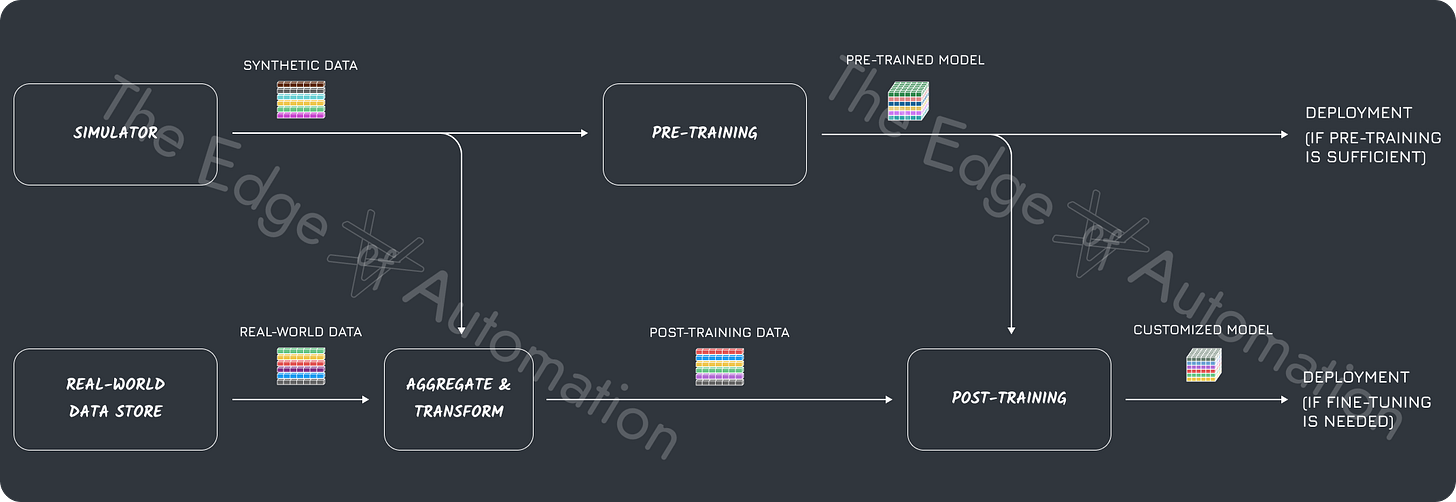
The real power lies in scale. While gathering physical training data might yield thousands of examples, simulators can spawn millions of parallel scenarios, systematically exploring edge cases that might occur once in a decade of real operation. A robot learning to handle delicate electronics can practice recovering from drops, adapting to bent pins, or managing unexpected component failures, all without risking actual hardware. This virtual experience translates into dramatically improved real-world performance and reliability.
The simulation ecosystem is evolving into a cornerstone product category for robotics deployment. Cloud-based platforms seamlessly integrate with training pipelines, enabling teams to fine-tune models on custom environments or validate safety protocols before live testing. Advanced data management systems create feedback loops between physical robots and virtual training, using real-world logs to continuously refine simulation accuracy. As large language and vision models mature, they'll enhance scenario generation further – creating hyper-realistic training environments that mirror specific facilities with uncanny precision.
This simulation infrastructure transforms how we develop and deploy robots. By enabling safe, rapid iteration at massive scale, it accelerates the path from prototype to production. More fundamentally, it provides the vast quantities of structured experience needed to create truly adaptable machines – a crucial step toward widespread automation.
Physical AI services establish the operational foundation needed for widespread robotics deployment. By providing the tools and infrastructure for managing intelligent machines at scale, they transform robotics from isolated demonstrations into a true industry capable of replacing human labor across diverse environments.
1.5. Virtual Private Cloud
The complexity of managing robot fleets far exceeds traditional automation systems. Each machine is a dynamic entity that perceives, thinks, and acts in real-time, requiring sophisticated coordination across multiple subsystems. This demands more than just basic networking – it requires a secure, isolated environment where companies can deploy AI models, integrate supply chain data, and orchestrate multi-robot operations without exposing critical systems to the public internet.
Virtual Private Clouds (VPCs) provide this essential backbone for industrial-scale robotics. Rather than scattering workloads across public services, companies create dedicated enclaves that host advanced Physical AI models, device management layers, and operational software. These private environments ensure that safety-critical processes – from fleet-wide updates to real-time teleoperation – remain under strict organizational control while enabling seamless synchronization between robots, software, and inventory systems.
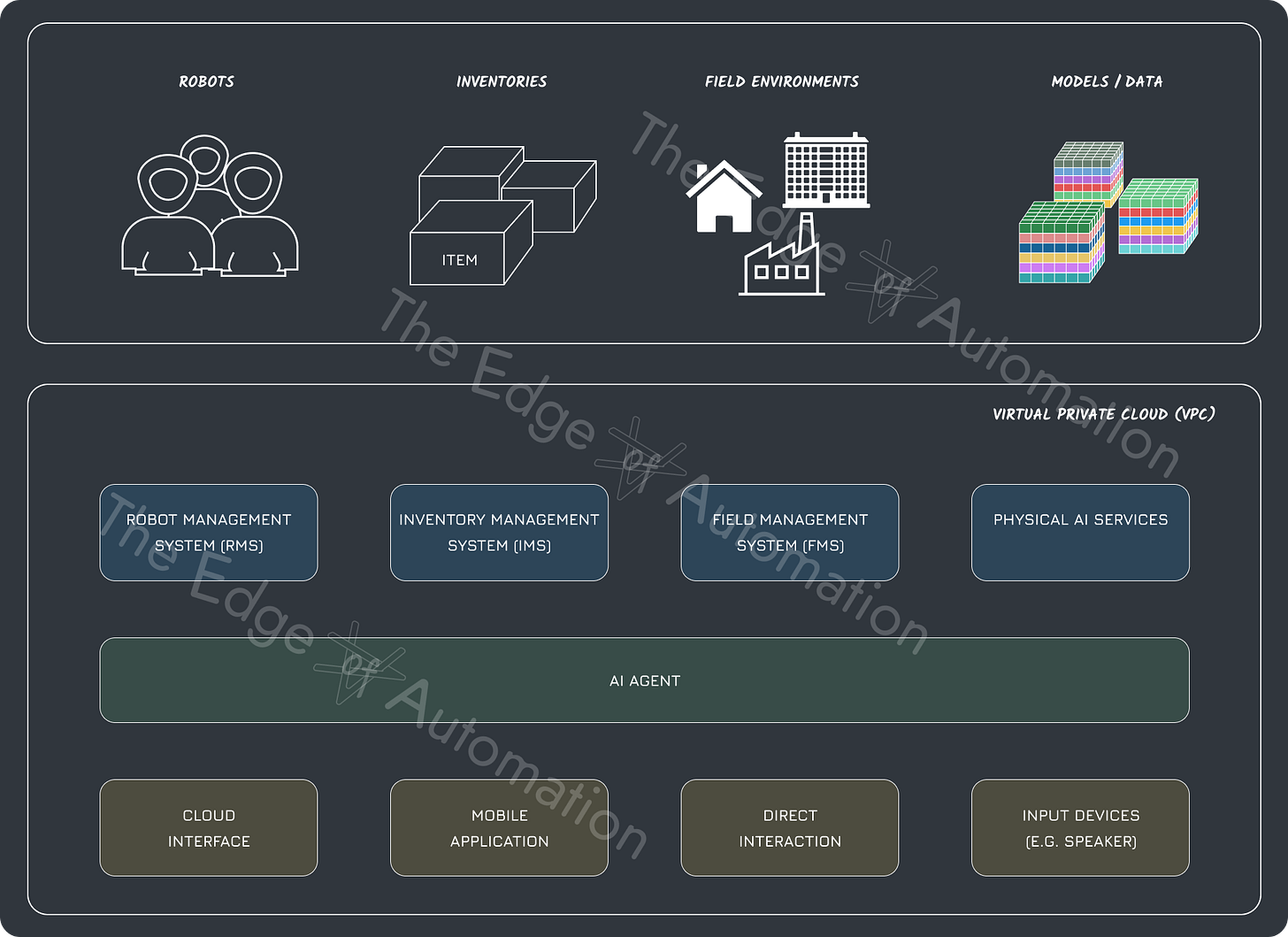
1.5.1. Robot Management Systems
Robot Management Systems (RMS) serve as the nerve center for deployed fleets, elevating basic tracking into comprehensive operational control. Where traditional systems relied on simple dashboards and minimal telemetry, modern RMS platforms fuse real-time oversight with predictive maintenance and granular control.
At the core, RMS provides operators with live access to each robot's status, location, and internal diagnostics. In a factory with hundreds of humanoids, the system enables drilling down to individual units, monitoring sensor streams, and initiating manual overrides during emergencies. If a robot encounters an unfamiliar scenario requiring human guidance, RMS can seamlessly transfer control to remote operators.
Beyond monitoring, these platforms enable predictive maintenance through continuous analysis of performance metrics – from joint torque readings to battery efficiency patterns. By detecting subtle degradation before catastrophic failures occur, RMS helps avoid costly downtime and keeps fleets operating at peak efficiency. The system maintains detailed audit trails of every robot's actions, critical for regulatory compliance and liability protection in heavily regulated industries like pharmaceutical manufacturing.
1.5.2. Inventory Management Systems
While RMS handles robots themselves, Inventory Management Systems (IMS) coordinate the materials and goods flowing through automated environments. Traditional inventory software falls short of what Physical AI demands – next-generation IMS must fuse supply chain data with real-time robot operations. When a humanoid forklift unloads materials, the system instantly updates stock levels, triggers reorders, and dynamically reallocates robot resources based on emerging priorities.
By hosting IMS within the same VPC as robot control systems, companies achieve unprecedented orchestration across supply chains. The system can dispatch robots to reorganize shelves based on demand spikes, redirect surplus inventory to outbound logistics, and even negotiate automatically with suppliers based on real-time consumption patterns. This tight integration transforms traditionally rigid supply chains into fluid networks that adapt continuously to changing conditions.
This capability proves especially crucial as manufacturing becomes more automated. Rather than humans playing phone tag with suppliers, the IMS autonomously manages inventory levels, negotiates pricing, and coordinates just-in-time deliveries. The system optimizes not just storage and movement of goods, but the actual deployment of robot labor – ensuring maximum efficiency across the entire supply chain.
1.5.3. Field Management Systems
Field Management Systems (FMS) extend robot orchestration beyond controlled industrial settings into broader operational environments. In manufacturing complexes, FMS coordinates maintenance robots responding to equipment failures, cleaning units managing public spaces, and specialized teams handling hazardous materials. The system continuously optimizes robot deployment based on real-time facility needs, traffic patterns, and priority tasks.
More importantly, FMS bridges the gap between robots and human-centric spaces. In retail environments, it routes shelf-stocking robots around high-traffic areas while coordinating with inventory systems. In office buildings, it manages robot cleaning crews based on occupancy patterns and maintenance requests. This sophisticated coordination enables robots to work seamlessly alongside humans without disrupting normal operations.
The implications extend into consumer environments, where FMS will evolve beyond today's limited smart home controls. Instead of simply toggling lights or thermostats, next-generation systems will orchestrate household robots handling everything from meal prep to laundry management. The FMS translates simple voice commands or app inputs into complex sequences of robot actions, coordinating multiple units to accomplish household tasks with minimal human oversight.
1.5.4. AI Agent Integration
Above specialized systems sits the final orchestration layer: AI agents that translate human intent into coordinated robot actions. Unlike narrow interfaces focused on specific domains, these agents provide natural language interaction that masks technical complexity behind conversational simplicity. A plant manager can say "reconfigure the line for next quarter's production" and watch as the agent coordinates with robot management systems, inventory databases, and field controllers to execute the change.
These agents don't just simplify interaction – they enable genuinely autonomous operation. By fusing data from multiple subsystems, they can make sophisticated trade-offs around scheduling, resource allocation, and task prioritization. A warehouse agent might redistribute robots based on incoming shipment volumes while ensuring maintenance schedules stay on track. More importantly, these agents learn from experience, continuously refining their understanding of operational patterns and constraints.
The implications extend beyond individual facilities. As agents grow more sophisticated, they accelerate the progression toward Level 4 and 5 autonomy – coordinating thousands of robots across sprawling supply chains or even entire cities. Their ability to reason about complex dependencies while maintaining safety constraints marks a crucial step toward systems that can truly operate without human oversight.
The Virtual Private Cloud represents more than infrastructure – it's the command center that enables true robotics at scale. By unifying AI models, sensor feeds, and operational workflows in secure, centralized environments, VPCs transform robots from isolated machines into orchestrated fleets capable of coordinating across entire facilities. This integration of cloud architecture with standardized hardware platforms paves the way toward Level 4 autonomy, where physical labor becomes cheap, distributed, and infinitely adaptable. The stage is set for our next frontier: the standardized components that will make this revolution possible.
2. The Existing Landscape
The robotics revolution collides with decades of entrenched automation practices across manufacturing, defense, and consumer sectors. Each domain represents a battleground where the existing players must either adapt to or be overwhelmed by the coming wave of intelligent, adaptable machines. This collision will determine not just the pace of adoption but the very shape of the emerging robotics industry.
2.1. Manufacturing
Manufacturing's dense network of equipment, expertise, and supply chains represents the ultimate testing ground for Physical AI. Unlike digital transformation that could bypass legacy systems, factory automation demands machines that seamlessly integrate with decades of established infrastructure. The industry's trajectory will be determined by three competing forces: industrial automators defending their technological moats, end customers facing existential pressure to automate, and channel partners acting as distribution channels. Their interplay will define how rapidly robots penetrate global manufacturing.
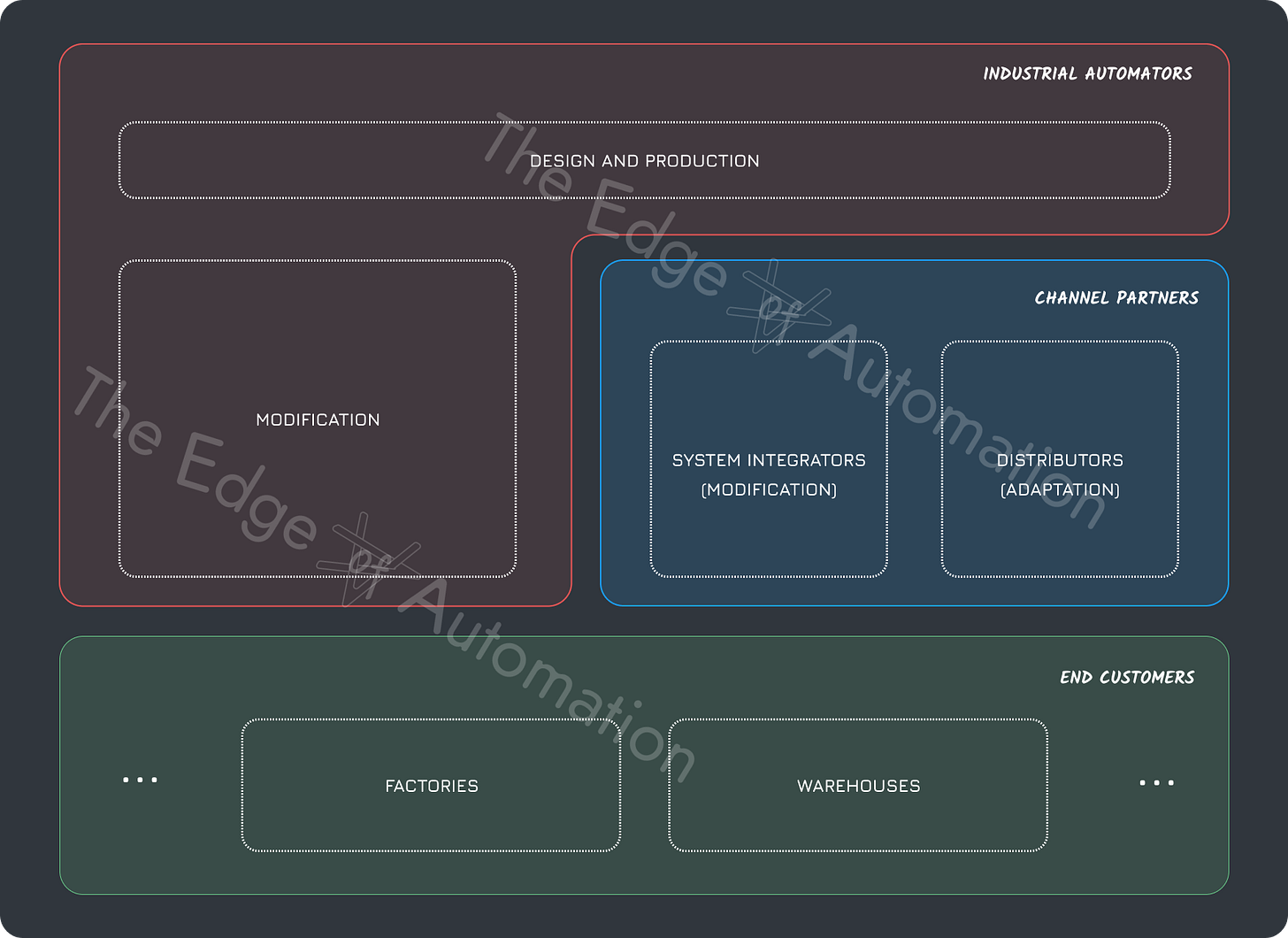
2.1.1. Industrial Automators
The industrial automation landscape has long been dominated by giants like ABB, KUKA, and FANUC – companies that mastered the art of specialized, repetitive automation. These incumbents excel at designing and deploying articulated arms, conveyor systems, and production cells that define modern assembly lines. Their success stems from decades of experience in controlled environments where tasks remain consistent and predictable.
Yet this very specialization now poses an existential challenge. While these companies have perfected the science of structured manipulation (Level 1), they've struggled to deliver the adaptable, general-purpose machines that could truly replace human workers (Level 3). Their robots can weld car frames with superhuman precision but falter at tasks requiring real-time adaptation or fine motor control. This limitation explains why smartphone assembly at Foxconn or intricate operations at TSMC still rely heavily on human labor – the existing automation paradigm simply cannot handle tasks demanding fluid response to changing conditions.
2.1.2. End Customers
Manufacturing end customers span a spectrum from massive multinationals to specialized contract manufacturers, each with distinct automation needs and adoption capabilities. Companies like Tesla represent the vanguard, aggressively pursuing advanced robotics to automate everything from vehicle assembly to battery production. Their deep technical expertise and vertical integration enable direct collaboration with automation providers, often pushing the boundaries of what's possible with current technology.
However, the vast majority of manufacturers lack Tesla's resources and technical sophistication. Many struggle to justify the massive capital expenditure required for traditional automation, especially when their production processes demand flexibility that current systems cannot provide. This creates a paradox: the companies most in need of automation – those facing severe labor shortages or cost pressures – often find themselves least able to adopt existing solutions.
2.1.3. Channel Partners
Channel partners serve as the crucial bridge between industrial automators and the diverse world of manufacturing applications. System integrators like Bright Machines transform generic robots into specialized tools through careful programming and integration, adapting standard platforms for specific manufacturing scenarios. They don't just resell hardware; they create complete solutions that make automation accessible to companies lacking internal robotics expertise.
Distributors form another vital layer, extending the reach of industrial automators through regional sales networks and ongoing support services. Companies like Proax Technologies handle everything from initial deployment to maintenance and upgrades, making automation viable for smaller manufacturers who couldn't otherwise access or maintain sophisticated robotics systems. This established network of relationships and technical expertise represents both an opportunity and a potential bottleneck for the emerging wave of adaptive robotics.
As the robotics revolution accelerates, this traditional manufacturing ecosystem faces inevitable disruption. The emergence of truly adaptive machines – capable of matching human dexterity and intelligence – threatens to undo decades of specialized automation expertise. Success in this transformed landscape demands rethinking how factories operate, how automation solutions are delivered, and how human labor interfaces with increasingly capable machines. The players who survive won't just adopt new technology but will reimagine their entire approach to manufacturing in an age where physical labor becomes an increasingly abundant resource.
2.2. Military
The military domain has emerged as a crucial accelerant for robotics development, where the pressing need for autonomous capabilities drives rapid innovation and deployment. While commercial robotics struggled to find viable business models, defense sectors worldwide poured billions into developing machines that could extend military reach while reducing human risk. Beyond specialized hardware, this investment is creating technological breakthroughs and supply chains that trickle down to the commercial sector.
Video: iRobot 710 Warrior with APOBS
The United States military leads in sophisticated autonomous platforms, deploying everything from carrier-based surveillance drones to underwater vehicles for anti-submarine warfare. These systems, though often tethered to human operators, have demonstrated the viability of robots in high-stakes environments where failure costs lives. Perhaps more importantly, defense contracts have sustained crucial research and development through periods when commercial applications seemed distant. Companies like iRobot exemplify this dynamic – their early success with armed ground robots for the US military created the technical foundation and capital reserves that later enabled their pivot to consumer products.
Video: The Rise of China’s Military Robotics
China's aggressive adoption of military robotics has accelerated this trend, creating a powerful feedback loop between defense needs and industrial capability. Their deployment of Lynx quadrupeds for ground operations and Haisi autonomous submarines for naval missions has spawned a sophisticated ecosystem of suppliers and integrators. Companies like Autel and Poly Technologies provide specialized drone platforms, while tech giants including SenseTime and Tencent develop advanced AI systems for autonomous operation. This military-industrial framework has proven particularly effective at nurturing specialized suppliers – firms like Xiamen Limbach Aircraft (now under US sanctions) for drone engines and Redlepus Vector Industry for critical components.
The Russia-Ukraine War has definitively proven that autonomous systems, particularly drones, represent a fundamental shift in military doctrine. Small, inexpensive drones have repeatedly outperformed traditional platforms costing hundreds of times more, demonstrating unprecedented cost-to-benefit ratios in actual combat. Despite relying primarily on basic autonomy and teleoperation, these systems have transformed modern warfare. As Physical AI matures and enables true autonomy in drone swarms and ground systems, the military applications will expand dramatically. This guarantee of sustained defense spending, combined with the emergence of sophisticated supplier networks, ensures that military demand will remain a powerful force shaping the robotics industry's early years.
2.3. Consumer
Despite widespread fascination with home automation, the consumer sector remains strikingly under-automated. Outside of niche successes – like iRobot's Roomba or Xiaomi's RoboRock vacuums – few examples exist of general-purpose robots capable of genuinely reducing humans at households, stores, and streets. This scarcity isn't due to a lack of market desire but the byproduct of technical and economic hurdles that have, until now, made universal consumer robots impractical.
For the most part, consumer robotics has remained confined to highly specialized gadgets. Robot vacuums and lawn mowers tackle repetitive chores, but each system handles a singular function in a controlled environment. Amazon, Google, and Apple have tried to bridge the gap with connected ecosystems – Alexa, Google Assistant, and Siri – yet these remain stuck at the "smart speaker" stage. Talking to your appliances certainly adds convenience, but it doesn't replace the physical tasks that absorb so much daily effort: cooking meals, washing dishes, restocking groceries, cleaning bathrooms, folding laundry, and more.
Wearables illustrate a similar pattern: devices like the Apple Watch or Meta's Ray-Ban Stories enhance how we receive information, but they don't undertake chores or labor on our behalf. Augmented reality glasses hint at a future where humans and robots might collaborate more seamlessly, sharing sensor data and offloading tasks to automated helpers. However, current AR hardware remains limited by, battery life, heat dissipation, and an underdeveloped software ecosystem – not to mention the weight and social friction of wearing headsets around town.
Video: Mobile ALOHA Housekeeping Robot
All of this points to a massive open space for disruption. The moment a humanoid or mobile manipulator can navigate cluttered homes, handle fragile household items, and interface smoothly with consumer electronics, the game changes. Household chores, from vacuuming under the couch to sorting laundry, become tasks a robot can truly handle on a plug-and-play basis without the need for elaborate reconfiguration. At that point, truly functional cloud-based orchestration platforms (akin to an industrial Virtual Private Cloud for the home) will emerge, enabling families to schedule cleaning runs, grocery restocks, and maintenance calls with the same ease they now order pizza online.
To understand how these robots will integrate with today's consumer environment, it's useful to borrow concepts from manufacturing: industrial automators, channel partners, and end customers. In consumer robotics, the "industrial automators" might be the large tech firms and specialized robot manufacturers building advanced home-service humanoids. They aim to create robust platforms that handle everyday tasks reliably. "Channel partners" could be the retailers and third-party integrators – like big-box electronics stores or specialized installation teams that offer set-up and ongoing support. Finally, the end customers are households and businesses themselves, ready to adopt, deploy, and fund these next-generation machines.
Video: An Early Examples of Agent-Driven Robot Control
Naturally, obstacles remain. Consumer-grade robots must be safer, more intuitive, and cheaper than their industrial counterparts, meaning the bar for reliability is even higher. If a machine flubs a welding seam in a factory, it's a technical hiccup; if it malfunctions while carrying a child's toy at home, it sparks alarm. Still, the underlying breakthroughs we've seen in sensors, actuators, and Physical AI indicate that these hurdles are surmountable. Given the consumer market's scale, any reliable humanoid or all-purpose household machine stands to generate enormous wealth for whoever cracks the puzzle first.
Consumer robotics finds itself on the cusp of transformation. Until now, the grand visions of a robot butler or a fully automated smart home existed mostly in science fiction. But with hardware standardization lowering costs, Physical AI broadening capabilities, and major cloud players eager to stake out new territory, the stage is set for a leap in everyday automation. The same evolutionary force propelling factories into the future – intelligent, capable, and endlessly adaptable robots – is about to do the same for living rooms and neighborhood stores.
From factory floors to military operations and consumer homes, the robotics revolution confronts deeply entrenched systems and practices. Legacy manufacturers who mastered static automation now face adaptable humanoids that threaten their core business. Military forces see autonomous swarms reshaping modern warfare. The consumer market, still waiting for meaningful home automation, stands ready for the first wave of truly capable household robots. This collision between old and new won't be gradual – it represents a fundamental reset that will determine which players survive to see the transformed landscape ahead.
3. New Entrants
A new wave of robotics companies has emerged, fundamentally different from traditional automation players in both vision and execution. Where incumbents remain trapped in specialized, inflexible solutions, these newcomers are building truly adaptable machines capable of replacing human labor across diverse environments. The landscape divides into two clear camps: vertically-integrated manufacturers who control the entire technology stack, and specialized providers who excel in crucial niches like advanced actuators or cloud orchestration. This evolution from fragmented experimentation to focused commercialization signal a critical milestone toward humanity's first true robotics industry.
3.1. Vertically-Integrated Robot Makers
Vertical integration represents the boldest bet in robotics: controlling everything from actuator design to AI models under one roof. Rather than piecing together third-party components and software, these companies build complete systems in-house, much like Apple crafting both silicon and operating systems. This strategy has become particularly visible among humanoid makers – Tesla's Optimus and Figure's humanoid platforms in the US, alongside Xiaomi's CyberOne and UniTree's emerging bipedal projects in China. Though some Chinese manufacturers like Unitree maintain flexibility to specialize in hardware while offering open software platforms, the allure of total control drives many toward comprehensive integration.
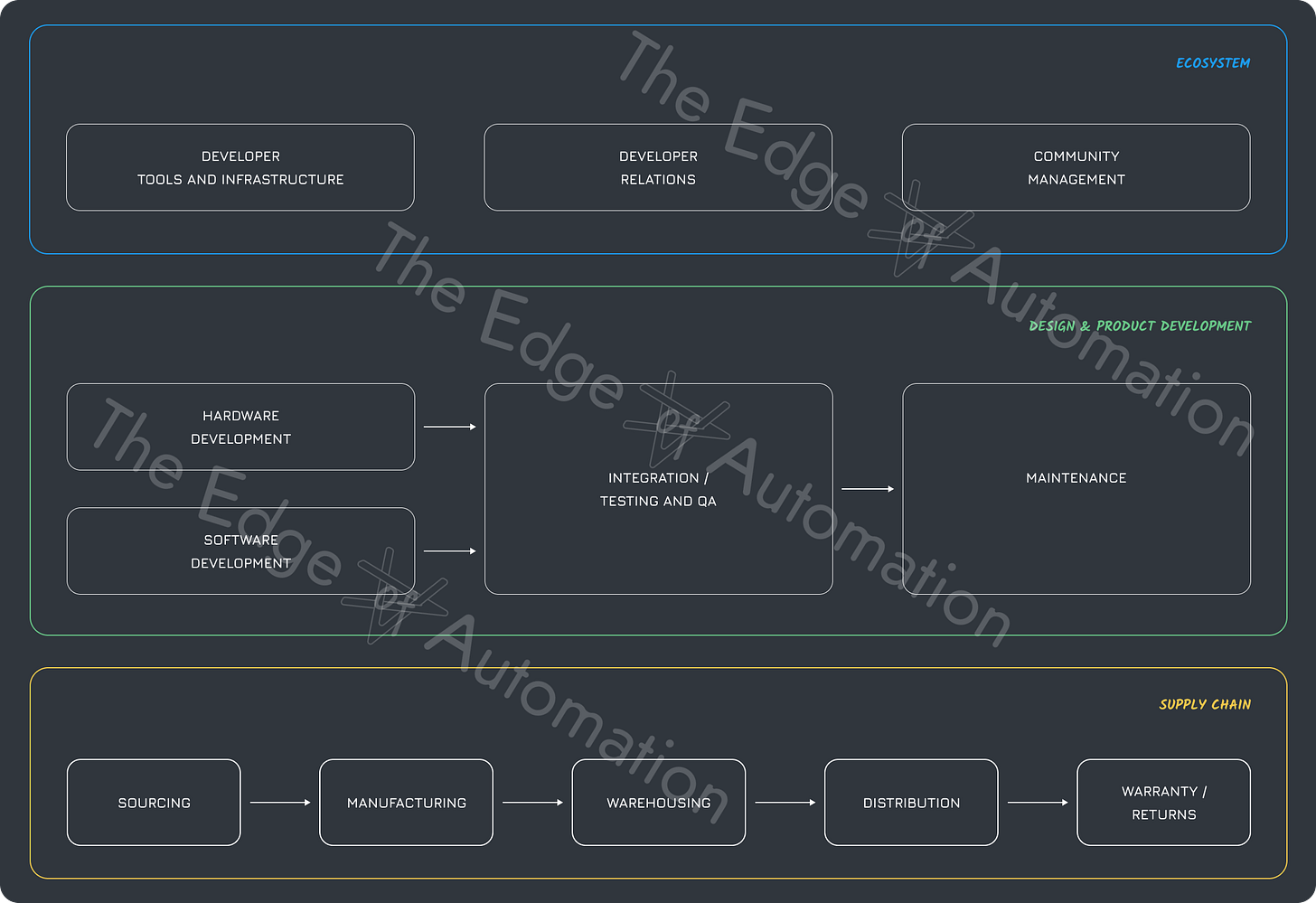
3.1.1. Strenghths and Weaknesses
Vertical integration offers powerful advantages in optimization and market control. When a single team designs both hardware and software, they can tune every component for maximum performance – from motor control algorithms that perfectly match actuator characteristics to AI models optimized for custom sensor arrays. More fundamentally, vertical integration grants independence from external suppliers and platform providers. A company that controls its entire stack can rapidly iterate on both physical and digital elements without waiting for partner upgrades or managing complex vendor relationships.
The market impact proves especially potent in regions with limited manufacturing infrastructure. In the United States, where domestic robotics production remains nascent, a successful vertical integrator can effectively define industry standards. By controlling core technologies while building trust through early deployments, these companies can establish themselves as default platforms that others must build around. This dynamic creates powerful feedback loops – early success attracts talent and capital, accelerating development while raising barriers to competition.
However, vertical integration demands extraordinary resources and organizational capability. Companies must simultaneously master mechanical engineering, electrical design, software development, and AI research – domains that traditionally require separate specialized teams. The cautionary tale of Zoox illustrates these risks: despite raising over $1 billion at a $3.2 billion valuation, their attempt to build both autonomous vehicles and software in-house led to scattered focus and delayed progress. Even with massive funding, coordinating innovation across multiple technical domains while maintaining cohesive product development proves remarkably challenging.
3.1.2. Vertical Integration in the US and China
The American market's relative vacuum in manufacturing creates unique opportunities for vertical integrators. With limited domestic competition and gaps in industrial infrastructure, companies that successfully deploy end-to-end solutions can rapidly establish market dominance. This position carries profound implications: as manufacturing automation accelerates, controlling both hardware and software platforms grants unprecedented influence over global production capabilities. The leaders of successful vertical integrators won't just build profitable companies – they'll reshape the fundamental power dynamics of industrial civilization.
China presents a markedly different landscape for vertical integration. Unlike the US market's relative emptiness, China offers intense competition backed by its colossal manufacturing infrastructure and deep talent pools in both hardware and software. Government oversight and a robust open-source community further complicate attempts to maintain fully proprietary systems. The sheer number of specialized component suppliers and system integrators creates pressure to adopt standardized interfaces rather than completely closed ecosystems.
The verdict on vertical integration isn't simple – it represents both opportunity and risk magnified to extraordinary scale. Those who successfully manage comprehensive development while avoiding organizational paralysis could define the next era of global manufacturing. Yet the path demands not just technical excellence but strategic clarity about which aspects truly require internal control versus where external partnerships might accelerate progress. As the robotics industry matures, we'll likely see a spectrum of approaches emerge, with some players maintaining full vertical integration while others strategically specialize in their areas of greatest strength.
3.2. Specialists
The robotics revolution isn't emerging as a monolithic industry dominated by vertically-integrated giants, but rather as an ecosystem of specialized players focusing on distinct parts of the automation stack. This specialization – from AI models to cloud services to standardized hardware – mirrors how the computer industry evolved once semiconductor makers, operating system developers, and application builders found their distinct niches. As robotics matures from research projects into commercial deployment, these specialists are creating the vital infrastructure that will enable widespread automation.
3.2.1. Model Providers
Model providers represent perhaps the most crucial specialization, delivering the AI that transform mechanical platforms into adaptive workers. These companies focus exclusively on developing and deploying Physical AI – whether foundation models that handle core perception and manipulation, task-specific models optimized for particular industries, or orchestration models that coordinate multiple robots. Their business models typically combine API access for real-time inference with traditional licensing for on-device deployment, making sophisticated AI accessible even to smaller robotics companies that lack massive R&D budgets.
3.2.2. Physical AI Service Providers
Physical AI service providers fill another vital niche by offering the tools and infrastructure needed to deploy robots at scale. Rather than selling models directly, they provide platforms for training, testing, and managing AI systems across large fleets. These services handle everything from fine-tuning models on custom datasets to monitoring deployment performance and generating synthetic training data through advanced simulation. By offering these capabilities as cloud services, they dramatically reduce the overhead of building and maintaining robotics infrastructure.
3.2.3. Virtual Private Cloud Providers
Virtual Private Cloud (VPC) providers take this infrastructure approach further by creating secure, dedicated environments optimized for robotics operations. These specialized clouds integrate AI models, management systems, and operational tools into cohesive platforms that can handle everything from individual robot control to facility-wide automation. Through AI agent interfaces, they enable natural language control over complex robot fleets while maintaining the security and reliability that industrial deployment demands.
3.2.4. Hardware Providers
The hardware side of specialization centers on standardized component makers who produce the physical building blocks of modern robots. From high-performance actuators to sophisticated sensor arrays, these companies are creating plug-and-play modules that dramatically reduce the cost and complexity of robot development. Rather than every manufacturer having to design custom parts, they can source proven components that integrate seamlessly into their platforms.
3.2.5. Specialists in the US and China
In the United States, this specialist ecosystem faces significant headwinds. The dominance of vertically-integrated players in promising sectors like humanoid robotics creates pressure toward closed, proprietary systems. These giants often prefer to develop critical components in-house or through exclusive partnerships, leaving limited space for independent specialists. While opportunities exist in niche markets or as suppliers to well-funded startups, specialists must navigate a landscape where the largest customers may become their biggest competitors.
China presents a starkly different environment, one naturally conducive to specialization. The country's vast manufacturing base already supports countless companies producing everything from precision motors to advanced vision systems. Government initiatives promoting robotics adoption have spawned numerous startups focusing on specific technical challenges, while intense competition drives continuous innovation in both hardware and software. This rich yet crowded ecosystem creates a competition ground for specialists who focus on particular components or capabilities.
The rise of these specialized players signals robotics' evolution from experimental technology into mature industry. While they may lack the high profile of vertically-integrated giants, specialists provide the essential infrastructure and components that make widespread automation possible. Their success suggests that the future of robotics won't be dominated by monolithic corporations but shaped by a complex web of focused innovators each mastering their specific domain.
4. Fueling the Fire: Where Money Comes From
The capital propelling robotics from laboratories into widespread deployment transcends traditional venture funding patterns. An unprecedented wave of investment flows from aging power structures that see automation as an existential imperative. Family dynasties, banking conglomerates, and industrial magnates – those who have built generational wealth on existing labor paradigms – recognize that robotics threatens to dismantle the very foundation of their influence.
This strategic urgency manifests in massive government initiatives that dwarf typical innovation funding. The U.S. CHIPS Act and China's aggressive robotics strategies reflect more than economic planning – they represent desperate bids to secure automated manufacturing supremacy. Nations understand that falling behind in robotics means losing control of production capabilities, effectively surrendering leverage in trade, security, and global power projection.
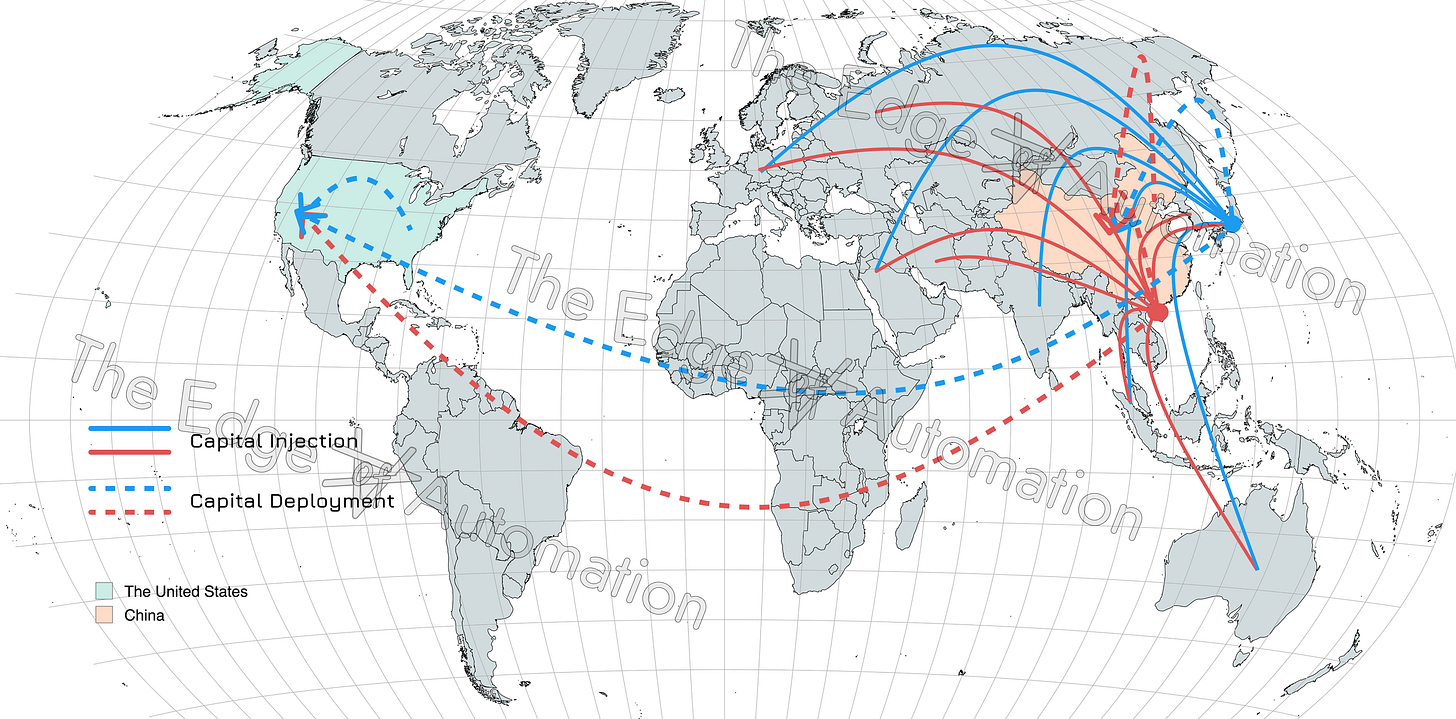
Traditional financial institutions are pivoting sharply toward robotics as conventional sectors show vulnerability. Banks and private equity firms, watching their real estate and energy portfolios face mounting uncertainties, see automated manufacturing as a crucial hedge against disruption. Family offices historically focused on wealth preservation now lead aggressive robotics investments, understanding that their multi-generational empires could crumble if they miss this transformation of human labor.
The investment cascade extends beyond traditional power centers. Tech giants, fearing irrelevance if AI jumps from digital services to physical machines without their involvement, are pouring resources into robotics research and acquisitions. Retailers and logistics companies, squeezed by supply chain volatility and labor costs, view automation as their survival strategy.
From sovereign wealth funds to private family offices, capital floods into robotics at a scale much greater than typical Silicon Valley deployments. The synergy of this desperate capital with maturing technology creates unstoppable momentum toward automation at scale. While breakthrough capabilities in Physical AI and advanced hardware make the robotics revolution possible, it's the fierce urgency of old money and institutional power that drives this unprecedented acceleration. The race isn't optional – for those who control today's economic systems, it's quite literally a matter of survival.
The robotics industry emerging today bears little resemblance to previous waves of automation. Instead of fragmented research projects or isolated factory deployments, we're witnessing the birth of a comprehensive ecosystem – from adaptable humanoid platforms to sophisticated AI models, from specialized component makers to desperate capital seeking refuge from disruption. This fusion of technology, talent, and unprecedented investment signals the approaching storm that will transition all of humnanity to the next chapter of history. Those who master this transformation will define the new world that emerges on the other side.
Conclusion: The Rising Tide
Throughout human history, four fundamental scarcities have constrained civilization's development: raw materials, labor, energy, and technology. While we've made incremental progress in managing these limitations, none has ever truly been overcome. Today, we stand at the threshold of dismantling one of these foundational constraints – the scarcity of physical labor. The robotics revolution marks the first time humanity has gained the capability to fundamentally transcend a core limitation that has shaped every aspect of how societies organize and operate.
The convergence of Physical AI, advanced hardware, and strategic capital creates an inflection point unlike any previous automation wave. This isn't a gradual evolution of existing technology but a systemic transformation that dissolves the bedrock assumption underlying our civilization: that the world requires human physical involvement to operate. When robots can genuinely match human adaptability across unstructured environments, they trigger a reset that reaches far beyond factory floors into the very foundations of economic and political power.
This transformation presents an existential challenge to established orders. Manufacturing will decouple from demographic constraints. Cities will reorganize around automated services. Supply chains will reconfigure based on robotics capabilities rather than labor availability. The power structures that have historically controlled and directed human labor – from industrial dynasties to political establishments – face a stark reality: adapt to the new paradigm or become irrelevant in the automated world ahead.
The coming wave offers no middle path. It creates a binary future: those who master robotics will shape the new civilization that emerges, while others will find themselves trapped in obsolete paradigms of value creation. Understanding these forces won't guarantee survival, but it illuminates the strategic imperatives that will determine which powers persist through this great reset. The tunnel ahead is long and dark, but recognizing the true nature of this transformation – not merely technological but civilizational – offers our best chance of finding a path through to the other side.
I think the coming together of robotics and AI is absolutely an impressive feat for mechanotronics. But what I worry about is the lack of understanding of what it means to embody these physical objects with thoughts. Thought hallucination is one thing, but in a society where we trust each other to be sane and not run each other over while driving or not steal each other's kids at playgrounds, how do we make sure robots don't have glitches? I am all about AI empowering labor forces, increasing efficiency, and optimizing our abilities in thinking and reasoning. Still, physical AI in a non-industrial setting just gives me the scaries. I am really curious to hear what people think.
I previously wrote a primer on physical AI here as well. It's nowhere as comprehensive as yours, though, but it dives deeper into the business side of things.
https://open.substack.com/pub/aiproem/p/physical-ais-chatgpt-moment-a-closer?r=itkz&utm_campaign=post&utm_medium=web&showWelcomeOnShare=false
any update on when parts 3 and 4 are coming out?